Summary
Human decision-making and concrete, observable actions about how to develop and use AI now will influence how successfully we navigate the transitions to a world with advanced AI systems. In this article, I apply behaviour science to increasing adoption of audit trails in AI development (Avin et al, 2021, after Towards Trustworthy AI, 2020) as a worked example to illustrate how this approach can identify, prioritise, understand, and change important decisions and actions. One useful heuristic is to ask the question “WHO needs to do WHAT differently” to help cut through complexity, build shared understandings of problems and solutions, measure progress or change, and improve credibility of & appetite for transformative AI governance work among AI actors.
Behaviour change is necessary for effective governance of transformative AI
AI Governance "relates to how decisions are made about AI, and what institutions and arrangements would help those decisions to be made well", (Dafoe, 2020). Behaviours are the observable actions that result from decisions, including:
- introducing a bill into parliament that prohibits companies in your country from selling AI hardware to entities in another country
- applying to work at Anthropic, an AI safety company
- documenting machine learning development processes in preparation for third party audits
That means that good human decision-making and behaviours about how to develop and use AI can steer us towards a much better future - one characterised by vastly increased flourishing and well-being, and bad human decision-making and behaviours about how to develop and use AI can steer us towards a much worse future.
Work in longtermist AI governance (especially in policy and field-building; Clarke, 2022) tries to create some valued outcome (e.g., improving US emerging technology policy) by designing or implementing some intervention (e.g., Open Philanthropy Technology Policy Fellowship). The way that these interventions would create the valued outcome is by changing behaviour (e.g., applying to fellowship; applying for a US policy role; persuading others to adopt a policy option that is more robust to advanced AI).
But how effective are these interventions in influencing the important behaviours that lead to these valued outcomes? In my experience working with government departments and large organisations on policy-relevant research and implementation, most groups that try to create some outcome like 'get people to comply with regulation X' just do what they usually do (e.g., try to educate, coerce, incentivise, punish) without investigating ‘what works’ to influence that specific behaviour in that specific audience and context. Sometimes this is due to a lack of understanding about what influences behaviour in other people (e.g., the typical mind fallacy), and sometimes it’s because the interventions they have the power to design and implement don’t address the behavioural influences.
Lots of organisations I've worked with say that in hindsight, "it's obvious" why their intervention didn't work... but if they knew that then they should have chosen a different intervention! Other organisations are sure they know why people aren’t enrolling in a program that would deliver valued outcomes, but when you investigate, the organisation’s intuition is totally wrong.
Incorporating behaviour into theories of change and program logics for AI governance activities is useful because it can help make assumptions explicit about how and why an intervention (e.g., offering a paid fellowship in fundamentals of AI safety) is supposed to lead to valued outcomes (safer development and use of AI). Behaviour science research can then test these assumptions by identifying and measuring influences on behaviour, and evaluating the effectiveness of interventions in changing behaviour[1].
This approach of identifying concrete, measurable actions to improve AI governance is aligned with Filling gaps in trustworthy development of AI (Avin et al, 2021, after Towards Trustworthy AI, 2020)[2]:
“[corporate AI ethics] principles often leave a gap between the “what” and the “how” of trustworthy AI development. Such gaps have enabled questionable or ethically dubious behavior, which casts doubts on the trustworthiness of specific organizations, and the field more broadly. There is thus an urgent need for concrete methods that both enable AI developers to prevent harm and allow them to demonstrate their trustworthiness through verifiable behavior.”
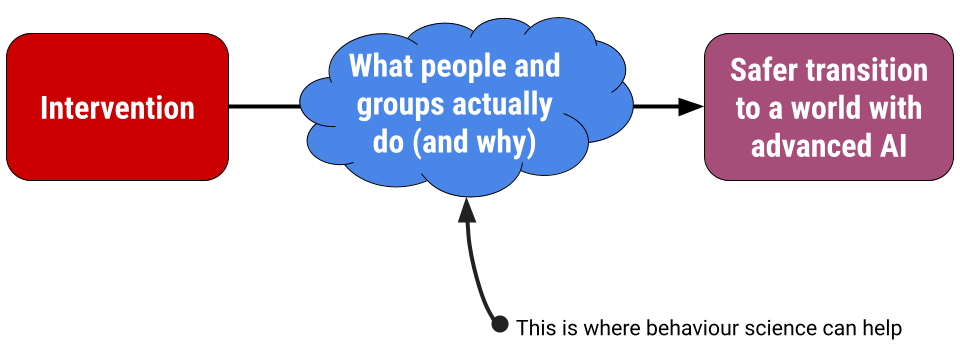
In the next section, I introduce a worked example of audit trails in AI development firms, one specific action recommended in Filling gaps in trustworthy development of AI .
Worked example: increasing the use of audit trails in AI development companies
In this worked example, I apply some common methods of behaviour science to demonstrate how it can be useful in understanding and influencing actions that lead to valued outcomes for AI governance.
In Filling gaps in trustworthy development of AI (Avin et al, 2021), one recommendation for improving safe development of AI is documentation of development processes, with the intent to make these processes available for third party auditing (“auditing trails”).[3] Examples of behaviours provided in the paper include documenting answers to questions asked during the development process (e.g., “Who or what might be at risk from the intended and non-intended applications of the product/service?”, Machine Intelligence Garage Ethics Framework, 2020), or recording the source and permissions of all data used in model training (e.g., “What mechanisms or procedures were used to collect the data? How were these mechanisms or procedures validated?”, Partnership on AI: ABOUT ML, 2021).
Identifying behaviour
For the purpose of the worked example, let’s imagine that we’re a well-known and respected non-profit with limited resources that is trying to improve transparency and verifiability of machine learning (ML) research done in mid-size AI development firms.[4]
Let’s imagine that we have already conducted or commissioned research to identify and prioritise a specific behaviour and a specific audience[5].
In this worked example, the result of this identification and prioritisation process is that we’ve selected documentation of development processes as a priority behaviour, because we think that increasing this behaviour will lead to improved AI safety and that it’s possible to influence with the levers / interventions we have access to.
WHO do we want to change behaviour | WHAT behaviour should they change |
ML development teams in mid-size AI development firms | Document ML development processes to create an audit trail |
Understanding influences on behaviour
Once we have identified WHO needs to do WHAT differently, we’d need to conduct further user research to diagnose the influences on this behaviour for this audience. What makes it more or less likely that this audience would do this behaviour? One good example of this kind of audience research is a report on a pilot of the ABOUT ML documentation framework, which sought to identify the enablers and barriers of documenting ML development in a pharmacovigilance organisation (Chang, 2022).
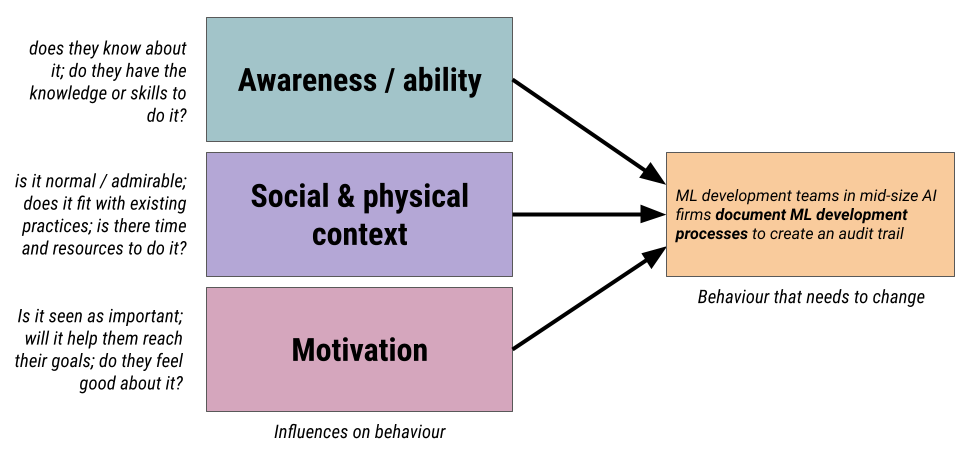
For the purpose of the worked example, let’s imagine we’ve conducted or commissioned some user research with our mid-size AI development firms, such as interviews, surveys, or observation of work practices. We’ve then categorised the behavioural influences according to awareness/ability, social and physical context, and motivation (this is a popular evidence-based framework used in behaviour change; Michie et al, 2011; Curtis et al, 2021). An example of one observation that fits under the social and physical context category is a concern from firms that documenting ML development for third party auditors may expose customer-provided data and cause a breach of privacy regulations.
Behavioural influence | Examples of observations from user research |
Awareness / ability: does the audience know about the behaviour, and do they have the knowledge or skills to do it? |
|
Social & physical context: is the behaviour normal and valued by others in the audience, does the audience have other current practices that support or conflict with doing it, and is there time and resources to make it happen? |
|
Motivation: does the audience believe the behaviour is important and/or valuable, does it align with goals, are there positive emotions associated with the behaviour? |
|
Designing and testing interventions to change behaviour
After identifying and diagnosing the behaviour, we now know a lot more than before about WHO needs to do WHAT differently, and also the influences on their behaviour. With this understanding, we are in a position to be able to design or select interventions to change behaviour.[6]
- We need to assess which interventions are feasible to design and test in a given context, with our resources and skills.
- We also need to assess which interventions are likely to be effective in creating behaviour change, considering our place within the actor ecosystem and what we know about influences on behaviour.
- Finally, we may want to design or select interventions that can be adapted to different jurisdictions or scaled up after a pilot phase if effective.
In this table, I describe several categories of behaviour change interventions and provide examples for the target behaviour of documenting ML development processes.
Intervention type | Examples |
Enablement Removal of external barriers to increase opportunities to carry out the behaviour |
|
Contextual restructuring Change to physical or social context in which the behaviour is performed |
|
Social modelling Social rules that indicate what are common and acceptable behaviours |
|
Persuasion Tailored communication in response to audience beliefs, emotions and biases to motivate behaviour |
|
Restriction Reduced opportunities to engage in alternative competing behaviour |
|
Training Personal strategies and skills to increase capacity to carry out the behaviour |
|
Coercion Expectations of punishment or cost for not doing the behaviour |
|
Incentives Expectations of financial or social rewards |
|
Education Increase knowledge and understanding |
|
The design process would iterate to try and select and then optimise an intervention (or package of interventions) that is feasible, effective, and scalable. Quick examples:
- Financial penalties (coercion) might not be feasible for this non-profit because it doesn’t have the power to punish non-compliance
- Assisting external firms to develop software to help document development (enablement) might be ineffective because it doesn’t address the key influences for behaviour, namely that there aren’t existing good documentation practices
- Creating a prize for best audit trail (incentives) might be highly scalable but its effectiveness needs to be validated - will it influence the behaviour of firms that aren’t winners?
The selected intervention(s) could be tested in a pilot trial to validate assumptions about feasibility, effectiveness, and scalability. What happens next depends a lot on the capacity of the organisation and its partners to evaluate the pilot trial, advocate for adaptation of a successful intervention into new contexts, or scale up the intervention to reach more firms or influence different practices.
The worked example in the context of AI governance
By stepping through this worked example, my intention was to describe the value proposition of behaviour science for identifying, understanding, and influencing concrete actions that lead to valued outcomes in AI governance.
- The behaviour of documenting ML development processes to create an audit trail among mid-size AI firms ML development teams was identified and prioritised.
- Research was conducted to understand influences on the behaviour: who was doing the behaviour, who wasn’t, and why.
- Then, behaviour change interventions were designed and tested to validate assumptions about feasibility, effectiveness, and scalability.
In this example, we focused on the context of a non-profit organisation going through this process alone, but behaviour science is also well suited to collaboration or co-design with other AI ecosystem actors. For example, AI safety researchers could be involved in identifying and prioritising behaviours. Regulators, policymakers, firms, and customers could be involved in exploring influences on behaviours, and can help to build momentum or appetite for change. Firms or policymakers could help validate assumptions about the feasibility of interventions during intervention design, lead a pilot trial, engage in impact measurement, and accelerate wider implementation of an effective intervention at scale.

Although the example was not specifically focused on advanced AI, this process could be applied to many different audiences and behaviours, such as:
- What are the behavioural influences on AI development firm organisational leaders to advocate for a windfall clause?
- Are AI safety courses focused on training and education addressing the behavioural drivers for career change in young talented professionals?
In the final section, I expand the perspective of behaviour science from this process focus to consider other ways that I think behaviour science can help advance AI governance goals.
Other ways behaviour science can help AI governance
There are at least four other ways I think applying behaviour science can help address challenges in AI governance.
First, behaviour science can help reduce complexity. The interdependence of technical and social changes in the development and use of advanced AI (Maas, 2021; Hendrycks, 2021; for a broader view see Kaufman, Saeri et al, 2021) means that it's very difficult to figure out what the problem is, where to intervene in the system, or understand the counterfactual effects of an intervention. Behaviour science is intentionally reductionist it can reduce the dimensions of a problem by identifying & extracting specific decisions or actions. Behaviour science methods also tend to be highly practical or pragmatic (e.g., behaviour identification and prioritisation), work well with methods for navigating complexity (e.g., systems or stakeholder mapping), and can leverage existing knowledge about that decision or action in other contexts (e.g., evidence and practice reviews).
Second, behaviour science can help to coordinate action among different system actors, all of whom have different perspectives on the problems and solutions in governance. These perspectives are often in tension, for example between narrow and transformative AI (Baum, 2017; Cave, 2019), or between the engineering and social science perspectives on feasible and effective strategies for safe AI (Baker, 2022; Irving & Askell, 2019; Winter, 2019). Questions such as “who needs to do what differently to achieve a valued outcome” or “what are people already doing, and why” can align these perspectives around concrete problems or solutions[7]. If agreement can be reached on which actors holds responsibility for change, or which actions need to increase / decrease, this can break through uncertainty / hesitancy biases, and improve collaborative capacity among system actors (e.g., each actor can then take or give responsibility for certain strategies to improve the situation). These questions are also readily answerable using behaviour science methods.
Third, behaviour science can help to measure progress towards or away from valued futures. Opaque or missing outcomes among people and organisations that advocate for better AI governance can make it hard to determine whether activity X or organisation Y is making progress, especially if they are pushing for policy or legislative changes (Animal Ask, 2022). And even if policy is successfully changed, this does not by itself develop, deploy, or use safer AI systems. Instead, it’s about human compliance with or adoption of the policy - both of which are observable actions (i.e., behaviours). The benefit of behavioural outcomes is that they can be counted / measured and this can be tracked over time. People and organisations can then see whether their activities are making progress towards or away from valued futures. This approach can be further enriched with adaptive policy-making (Hasnoot et al, 2013; Malekpour et al, 2016) and other methods for planning under uncertainty (Walker et al, 2013)
Fourth, behaviour science (especially when framed as behavioural economics, behavioural public policy, or behavioural insights) is a credible and normal approach to shaping public and private policy design and implementation (Afif et al, 2019). The framing of existential risk or unborn trillions as moral patients can be seen as ‘weird’ and way beyond the time or influence horizon that most people incorporate into their decision-making. Today, AI is usually seen through narrow lenses of equity, ethics, transparency in decision-making, and data security. Using behaviour science to identify, explain, or suggest behaviour changes aligned with better transitions to worlds with advanced AI is more likely to be accepted and acted upon because this approach is common in dealing with other social issues.
Conclusion
Human decision-making and concrete, observable actions about how to develop and use AI now will influence how successfully we navigate the transitions to a world with advanced AI systems. Through a worked example focusing on documentation of development processes among mid-size AI firms ML development teams, I described how behaviour science can help to identify and prioritise behaviours that are important for achieving valued outcomes; understand the influences on those behaviours; and design and test interventions that can change those behaviours. I also argued that applying behaviour science to challenges in AI governance can help cut through complexity, build shared understandings of problems and solutions, measure progress or change, and improve credibility to groups outside the core AI safety community.
- ^
Behaviour science is an applied interdisciplinary approach that uses social science methods (especially psychology and economics) to try and understand, predict, and/or influence human behaviour. Theories or models of behaviour change often distinguish between several types of behavioural influences that make behaviour more or less likely. The suite of behaviour science methods can be applied to identify who needs to do what differently as a first step in designing interventions that target specific groups (the ‘who’) and attempt to change specific behaviours linked to valued outcomes (the ‘what’). They can also be applied to identify and understand drivers of behaviours, which can help to design interventions that address those drivers and are therefore more likely to work. Although behaviour science has been applied with success to making incremental improvements to relatively stable systems, I have also led and supported behaviour science research (e.g., Kaufman, Saeri et al 2021 [open access]) that has grappled with sustainability transitions, and other systems under change (for more on the relationship between AI development, AI governance and systems change, see Maas, 2021; Hendrycks, 2021)
- ^
This paper, published in the journal Science, is a summary of a longer report from a wide range of people and organisations who work in AI (Towards Trustworthy AI; Brundage, Avin, Wang, Belfield et al, 2020).
- ^
The topic of how or whether third party audits improve AI safety is discussed in more detail in the full Towards Trustworthy AI report (p. 11).
- ^
In this worked example, we focus on ‘mid-size AI development firms’ as a way of indirectly setting a lower bound on the number of actors, e.g., at least tens or hundreds of firms. Behaviour science works best at scale by identifying population-level (or segment-level) influences on behaviour and designing interventions that address these influences. While behaviour science is also often applied within / for a single organisation or a small number of actors, different methods to those discussed in this worked example can be a better fit (for an academic discussion of these different ‘lenses’ on behaviour, see Kaufman, Saeri et al 2021 [open access]).
- ^
We could identify audiences and behaviours through a combination of desktop research (e.g., evidence or literature review, which would have identified the Avin et al and Partnership on AI papers), and practitioner engagement (e.g., interviews or workshops with policymakers, ML development teams, AI safety researchers). We could prioritise and select behaviours using multi-criteria decision analysis (MCDA; Slattery & Kneebone, 2021; Esmail & Geneletti, 2018) or other expert (Hemming et al, 2017) and participatory decision-making approaches (Bragge et al, 2021).
- ^
More on feasibility: in this worked example, we are a well-known and respected non-profit with limited resources. This means the interventions / levers available to us are different to those available to individuals or teams within an organisation, regulators, civil society, industry networks, or other actors in the AI development ecosystem. Interventions that rely on clear and persuasive communication, leveraging social relationships or reputation, or capacity-building (e.g., training or education) are likely to be more feasible for our organisation. In contrast, coercing firms through legislation, or punishing non-complying firms might be impossible for our organisation.
More on effectiveness: In this worked example, we might be highly trusted compared to other actors, which might increase our access to teams within firms or increase the prestigiousness of a non-cash prize we establish for audit trails. Conversely, we may lack content knowledge about what kinds of process documentation is in fact effective for reducing risk or improving safety, or we may lack capacity to deliver training and education. Ultimately, effectiveness also depends on the perceived acceptability or tolerance of an intervention by the audience (e.g., firms and teams).
More on adaptability / scalability: We may want other ecosystem actors to take responsibility for large scale implementation (e.g., have a regulator adapt a voluntary reporting scheme used by some firms into a mandatory reporting requirement required for all firms). If so, then we would want to ensure that any intervention was simple, tested in real-world settings, adaptable to different organisational contexts, were highly acceptable to people and groups responsible for implementing, and had credible evidence for their effectiveness (scaleuptoolkit.org; Saeri et al, 2022).
- ^
Behaviour science is not the only approach that can pose and answer questions like this. However, behaviours are a useful 'boundary object', meaning a concept that is understandable to groups from different perspectives (e.g., academics, policymakers, industry), and which can act as a coordinating mechanism for action. For a brief introduction watch Lee, 2020, Boundary objects for creativity & innovation.
Acknowledgements
Thank you to Shahar Avin, Emily Grundy, Michael Noetel, Peter Slattery, and one additional reviewer for their helpful feedback on this article. This article is adapted from a talk I gave at EAGxAustralia in July 2022 (YouTube recording).