If you want to get people to do things (like learn about AI Safety) you have to offer them something valuable.
Here’s one of the posters we used when I was in charge of marketing for the Columbia EA group:
It’s a pretty graphic, but what valuable thing is it offering?
The message is “scan this link to talk about AI.” To be fair, people like talking about AI. We had applicants.
But we didn’t attract talented ML students.
If you want to attract talented people, you have to know what they want. Serious and ambitious people probably don’t want to sit around having philosophical discussions. They want to build their careers.
Enter ML @ Berkeley, a thriving group of 50 ML students who put 15 hours per week into projects and courses to become better at ML. No one gets paid – not even the organizers. And they are very selective. Only around 7% of applicants get in.
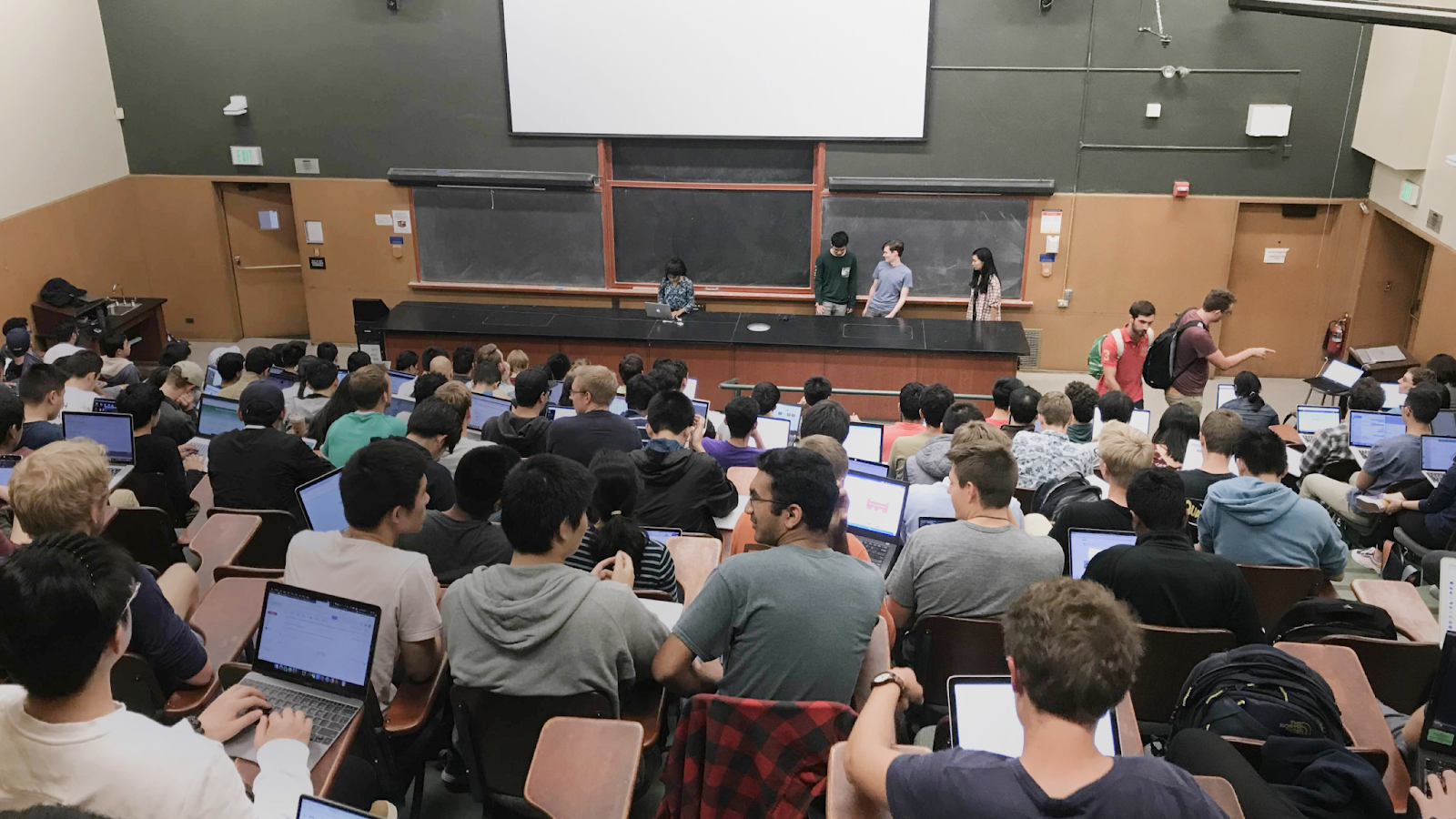
Why is this group successful? For starters, they offer career capital. They give students projects that often turn into real published papers. They also concentrate talent. Ambitious people want to work with other ambitious people.
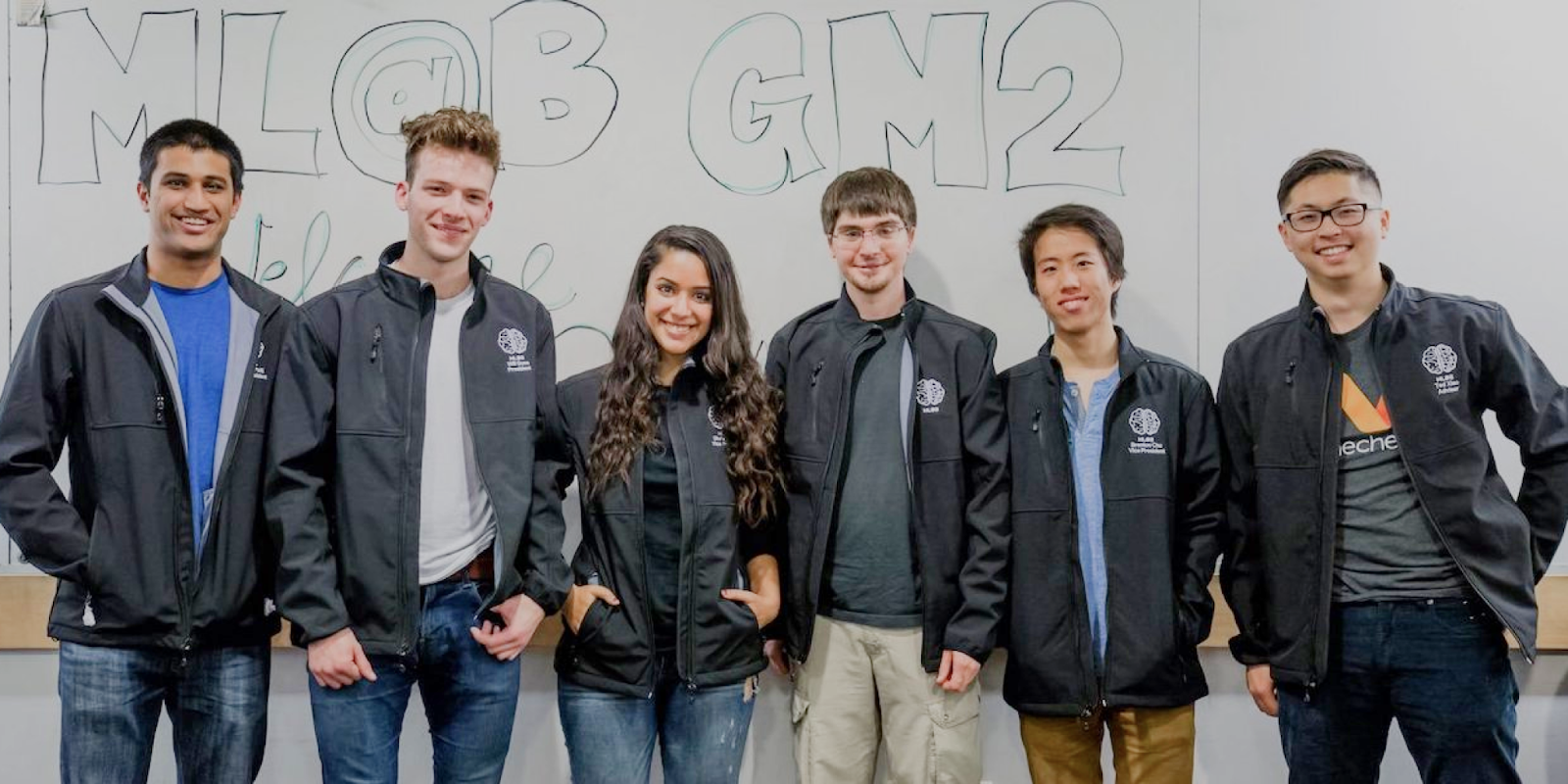
AI safety student groups should consider imitating ML @ Berkeley.
I’m not saying that we should eliminate philosophical discussions and replace them with resume boosting factories. We still want people to think AI Safety and X-risk are important. But discussions don’t need to be the primary selling point.
Maybe for cultivating conceptual researchers, it makes more sense for discussions to be central. But conceptual and empirical AI Safety research are very different. ML students are probably more interested in projects and skill-building.
More rigorous programming could also make it easier to identify talent.
- Talking about AI is fun, but top ML researchers work extremely hard. Rigorous technical curricula can filter out the ones that are driven.
- There is nothing like a trial by fire. Instead of trying to predict in advance who will be good at research, why not have lots of people try it and invest in those that do well?
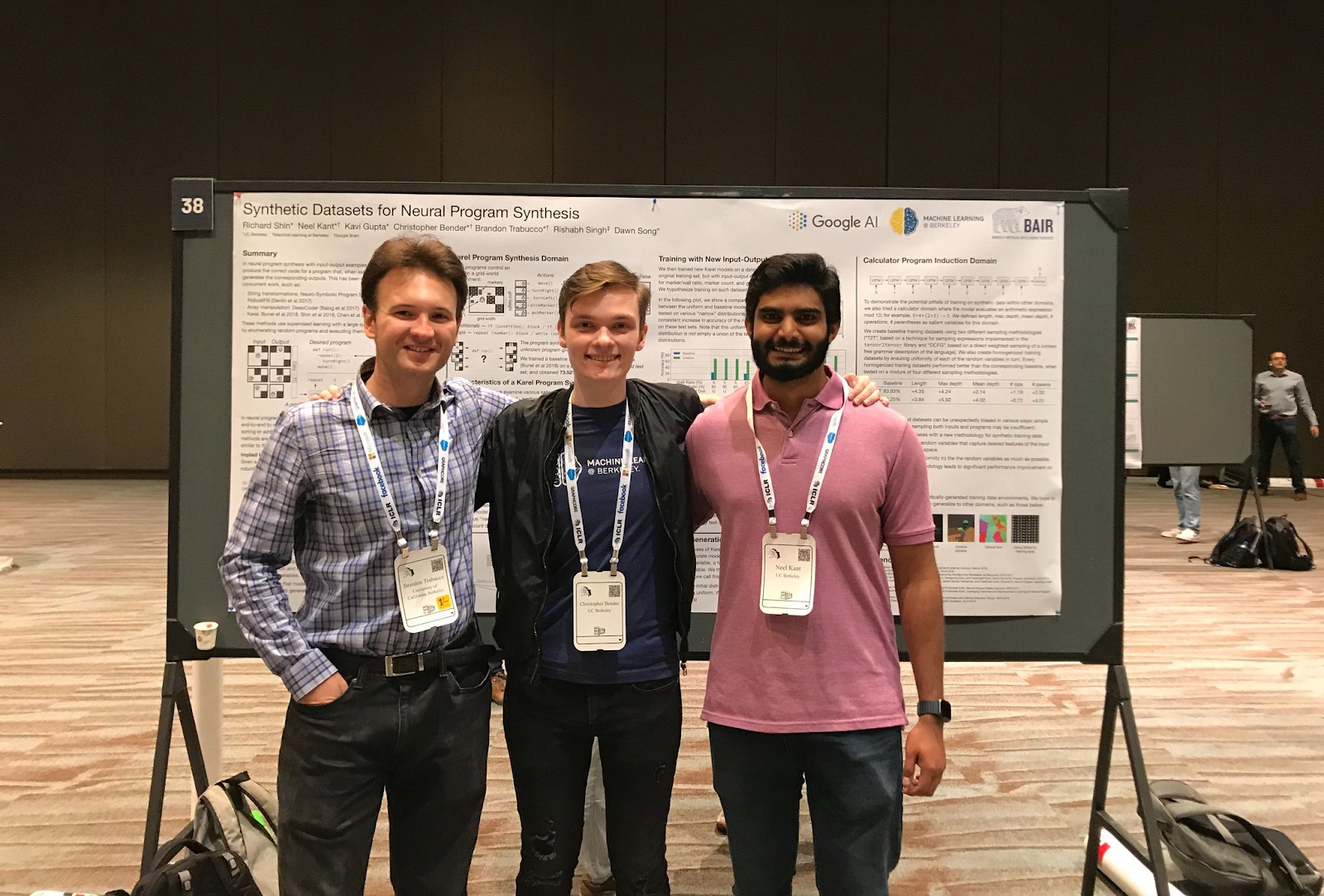
USC field builders are experimenting with a curriculum that, in addition to introducing X-risk, is packed-full with technical projects. In their first semester, they attracted 30 students who all have strong ML backgrounds. I’m interested in seeing how this goes and would be excited about more AI Safety groups running experiments on these lines.
People could also try:
- checking whether grad students are willing to supervise group research projects.
- running deep learning courses and training programs (like Redwood’s MLAB)
- running an in-person section of intro to ML Safety (a technical course that covers safety topics).
Conclusion
As far as I can tell, no one has AI safety university field-building all figured out. Rather than copying the same old discussion group model, people should experiment with new approaches. A good start could be to imitate career development clubs like ML @ Berkeley that have been highly successful.
Thanks to Nat Li and Oliver Zhang for thoughts and feedback and to Dan Hendrycks for conversations that inspired this post.
Hey thanks for the post, I agree with a lot of the takeaways here. I think these projects are also good for helping less confident students realise they can contribute to safety research.
I just wanted to flag that we've been running a program in Oxford that's much as you described. It is related to Oxford's AI society, which has a research program similar to ML@UCB.
So far the projects have been great for students "later in the pipeline" and to be honest, a lot of fun to run! If anybody is interested in setting a program as Josh describes, feel free to reach out to me at my email or see our post.
Oo exciting. Yeah, the research program looks like it is closer to what I'm pitching.
Though I'd also be excited about putting research projects right at the start of the pipeline (if they aren't already). It looks like AGISF is still at the top of your funnel and I'm not sure if discussion groups like these will be as good for attracting talent.
I really appreciate this kind of post :) Agree that no one has AIS field-building figured out and that more experimentation of different models would be great!
One of my main uncertainties about putting these kinds of research projects early on in the pipeline (and indeed one of the main reasons that the Oxford group has been putting it after a round of AGISF) is that having one early on makes it much harder to filter for people who are actually motivated by safety. Because there is such demand for getting to do research projects among ML students, we worried that if we didn't filter by having them do AGISF first, we might get lots of people who are actually mainly interested in capabilities research; and then be putting our efforts and resources towards potentially furthering capabilities rather than safety (by giving 'capabilities students' skills and experience in ML research). Do you have any thoughts on this? In particular; is there a particular reason that you don't worry about this?
If there's a way of running what you describe without having this be a significant risk, I'd be very excited about some groups trying this approach! And as Charlie mentions, the AI Safety Hub could be very happy to support them in running the research project (even at this early stage of the funnel). :))
That's a good point. Here's another possibility:
Require that students go through a 'research training program' before they can participate in the research program. It would have to actually help prepare them for technical research though. Relabeling AGISF as a research training program would be misleading, so you would want to add a lot more technical content (reading papers, coding assignments, etc.) It would probably be pretty easy to gauge how much the training program participants care about X-risk / safety and factor that in when deciding whether to accept them into the research program.
The social atmosphere can also probably go a long way in influencing people's attitudes towards safety. Making AI risk an explicit focus of the club, talking about it a lot at socials, inviting AI safety researchers to dinners, etc might do most of the work tbh.
Ha, I saw the first poster and thought "I really like that!" But I think you make good points here - appreciate your thinking.