Summary
- 44% of respondents reported changing careers plans based on EA principles, while 32% had donated >10% of income, and 28% had applied for a job or degree program based on EA principles
- Higher percentages of male respondents report donating >10% of their income, taking the GWWC pledge, and applying for 80,000 Hours coaching.
- Respondents who selected some racial category other than white were more likely to be member of the EA Facebook group
- Earlier cohorts (EAs who got involved in a given year) are more engaged than more recent cohorts, though this appears to level out for those who joined in 2015 or before. Later cohorts are also more engaged when comparing the 2020 EA Survey than in the 2019 Survey. (That said, we cannot rule out these results being driven by less engaged EAs dropping out over time.)
- We observe inverted u-shape shaped relationships between age and engagement, and the age when respondents got involved in EA and engagement. Across cohorts, engagement is highest among those who get involved in EA at ~20 years old, and lower in those who join at younger or older ages. Engagement peaks at age 20 for those who joined EA most recently, and then is progressively later for those who joined EA earlier.
- We explore a number of models exploring associations with engagement. Across these models we tend to find that older respondents are less engaged, while respondents who live in major EA cities or the UK/Ireland appear more engaged.
Introduction
In this post we describe people’s self-reported levels of engagement in EA, what activities related to effective altruism they have completed and their group membership. We also describe differences in these modes of engagement across groups (gender, race, age, time in EA etc.) and present a series of models looking at factors associated with higher engagement. This may help identify which groups are currently more engaged, which groups are likely to become more engaged and which factors may lead to EAs becoming more engaged.
To see the code for most of the models and graphs presented below, as well as some additional summary statistics (and a further discussion of modeling goals and issues), please see the dynamic document linked here.
Measures of engagement
Self-reported Engagement
We employed the same self-reported engagement item as in 2019. As before, and as we’d expect, we got very few respondents to the survey who reported the lowest level of engagement with EA. As we discussed elsewhere, we expect that there are actually more people in the population at the lowest levels of engagement with EA, per the funnel or concentric circles model, but that people who are less engaged in EA are commensurately less likely to take the EA Survey.
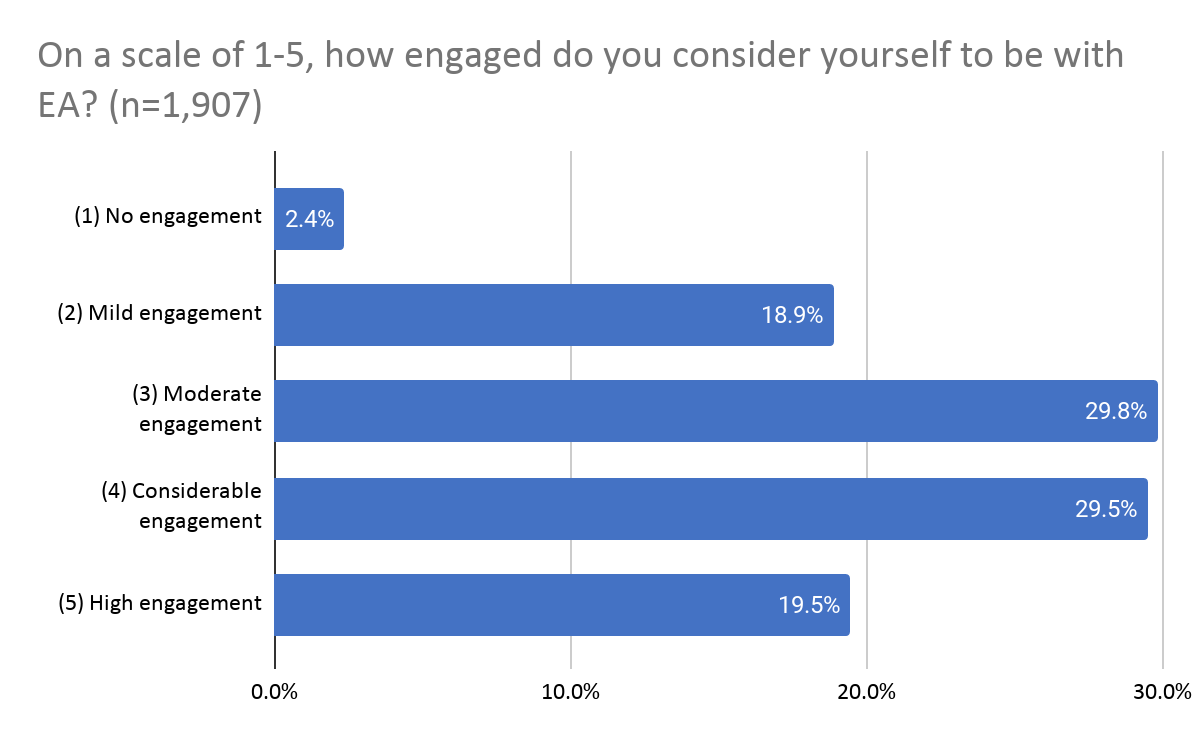
This year the distribution of responses was slightly different, with a lower share of respondents at level 3 and a higher percentage at level 4.[1] (As always, these year-to-year comparisons may reflect either an actual change in average engagement or simply a change in relative survey response rates.)
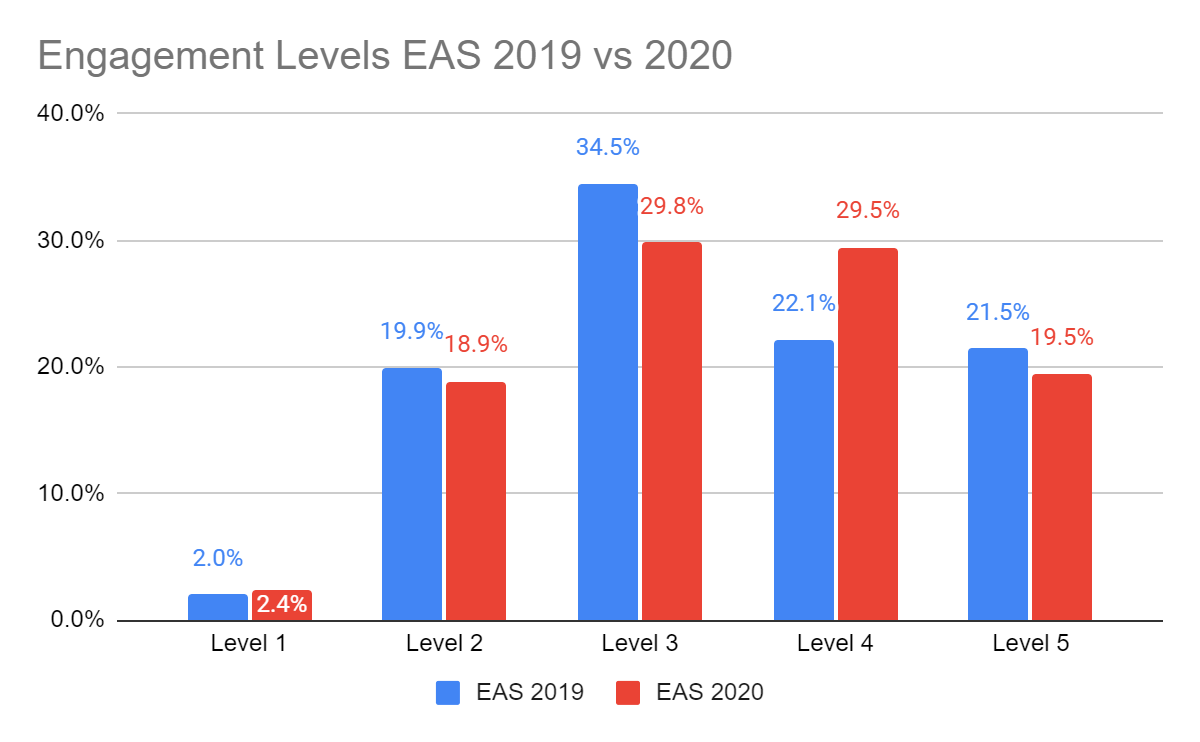
Activities
We also examined a series of activities related to EA that respondents may or may not have taken. As we made explicit in the survey itself, we do not think that it makes sense for all EAs to partake of all of these activities.
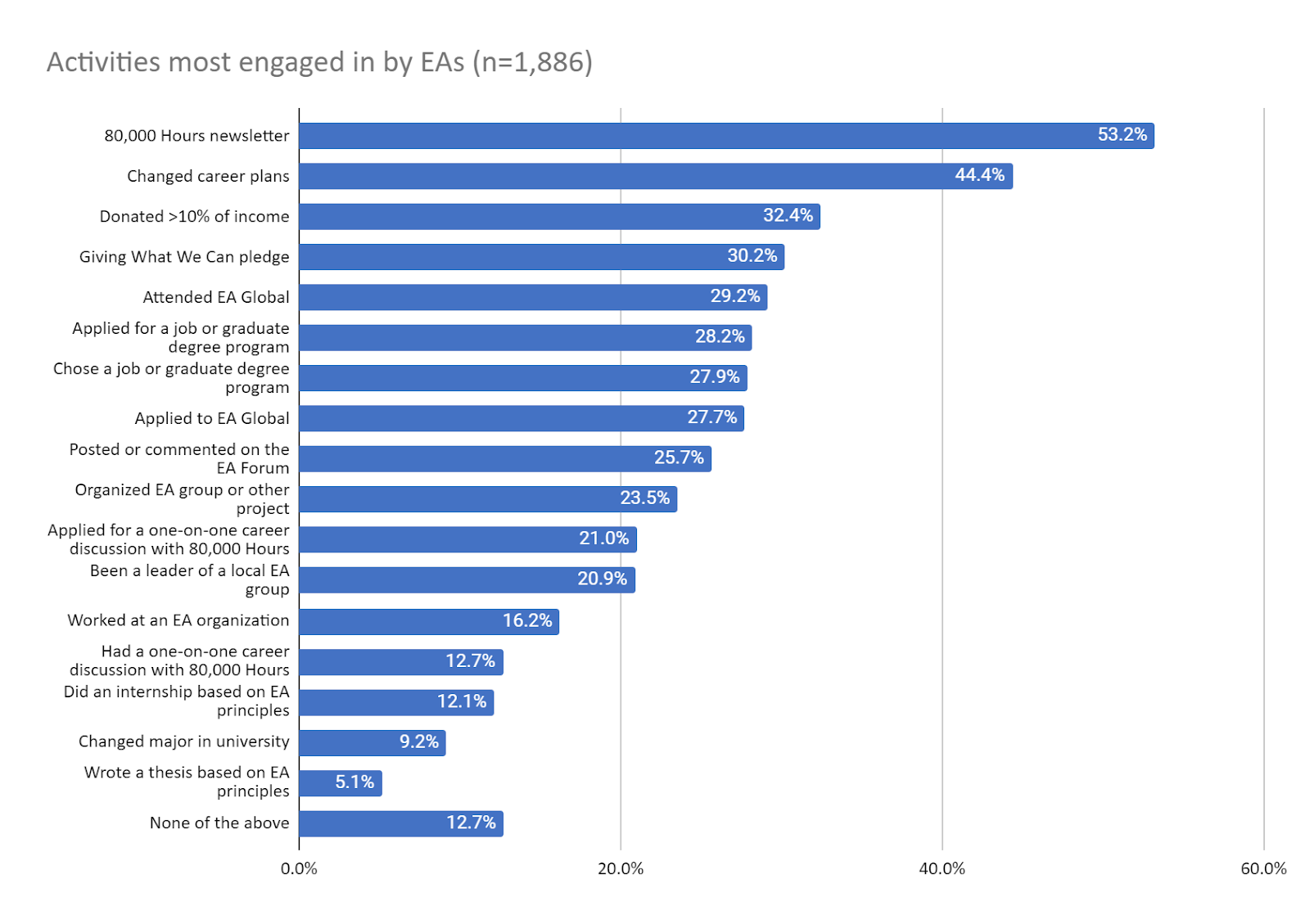
We found that over half of respondents are subscribed to the 80,000 Hours newsletter and almost half report having changed career plans. Markedly more respondents reported having changed career plans that reported having donated >10% of their income or taken the Giving What We Can pledge, and similar percentages (just under 30%) reported having attended or applied to EA Global and applied for or chosen a job or graduate degree based on EA principles. Very few respondents indicated having taken the more student-specific actions, changing major in university or writing a thesis based on EA. Also of note, only slightly over 10% reported having taken none of the specified actions.
Group membership
We found a similar pattern of group membership to previous years. As we have noted before, local group membership has dramatically expanded in recent years, and now close to half of respondents report being members of a local group.
Conversely, only 38.5% are members of the EA Forum, though this itself represents a substantial increase since 2019, when 29.5% of respondents reported being members of the Forum and 2018 when only 20.1% of respondents reported being members of the Forum.
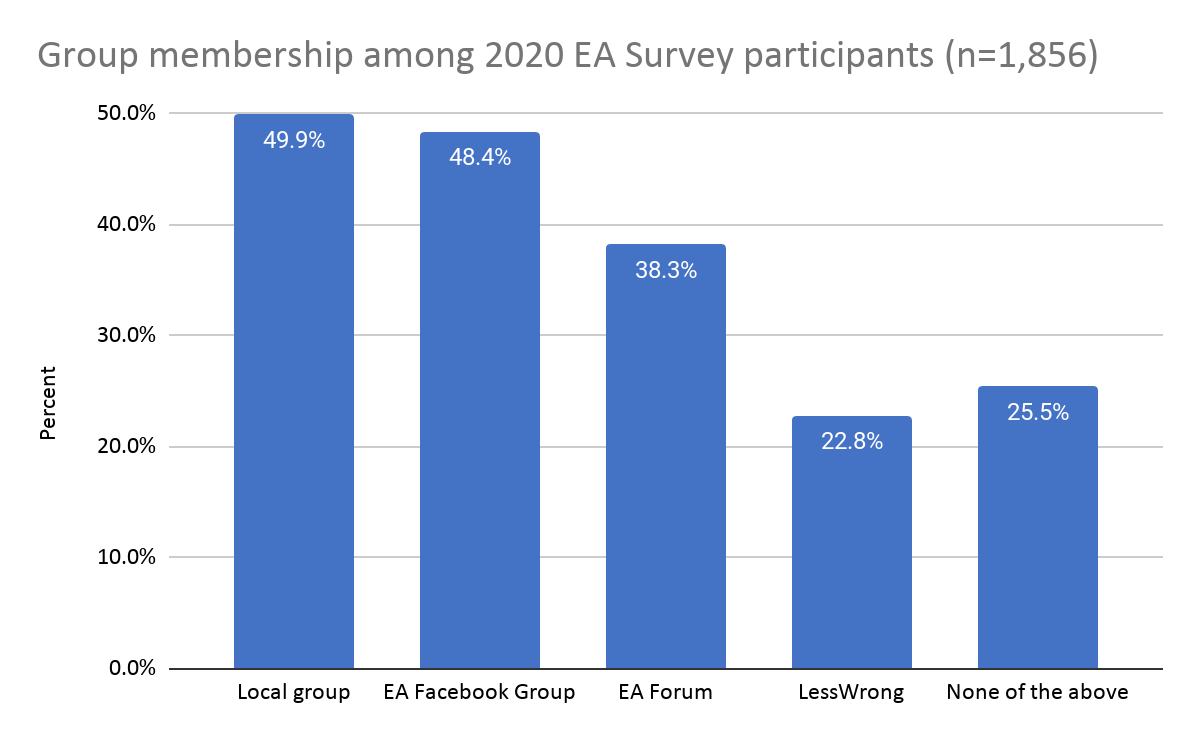
Relationship between engagement measures and validation
We also examine the relationships between the different kinds of engagement discussed above. This may help confirm whether the self-reported engagement scale (which we focus on in later parts of the post) seems to be tracking other, more concrete, kinds of engagement.
Group membership and self-reported engagement
There is a substantial increase in the numbers of respondents who are members of different groups, as we move up the engagement levels. EA Forum membership is particularly acutely associated with only the highest levels of engagement (>80% of Forum members report being levels 4-5 engagement), whereas local groups are slightly more common at lower engagement levels (with only 69% of local group members being levels 4-5 and 25% being level 3).
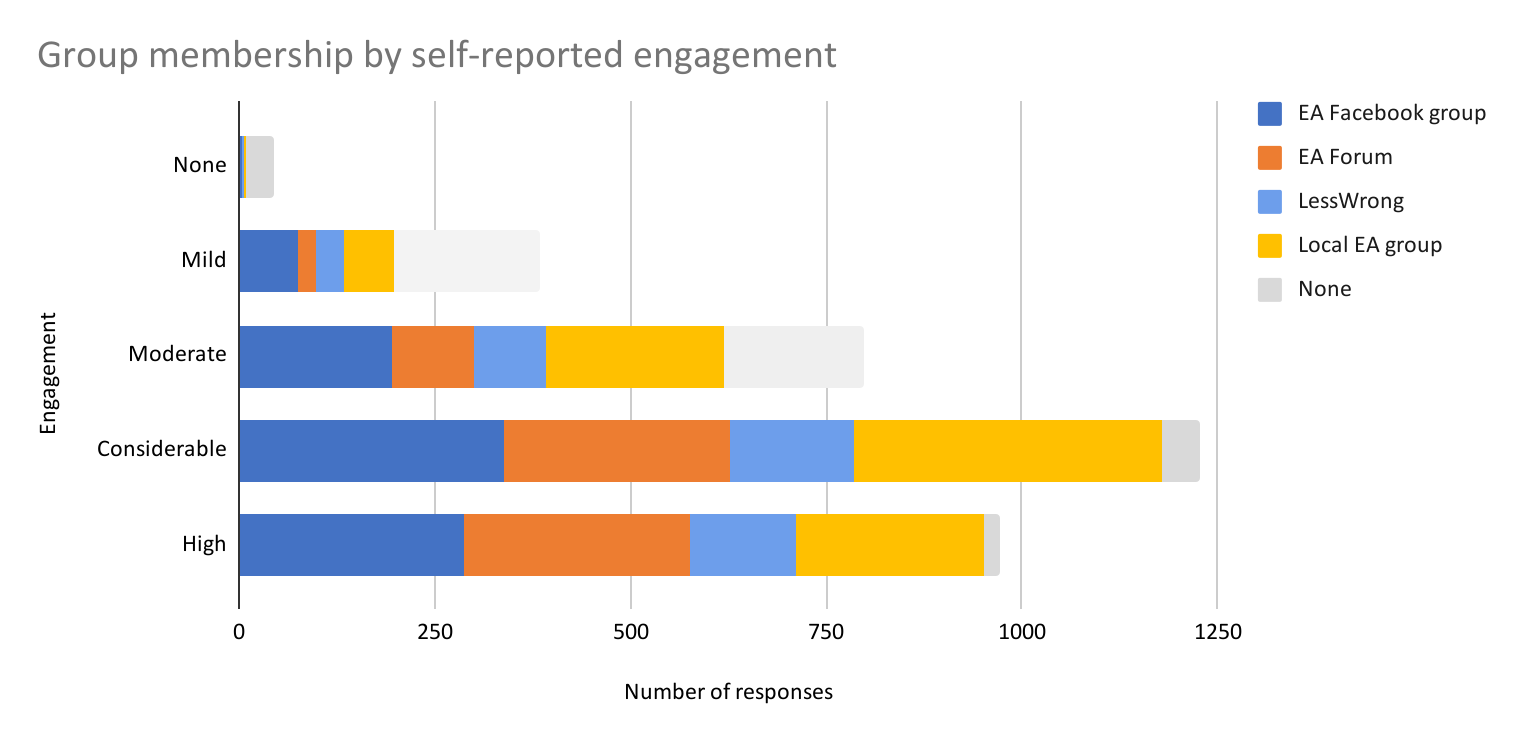
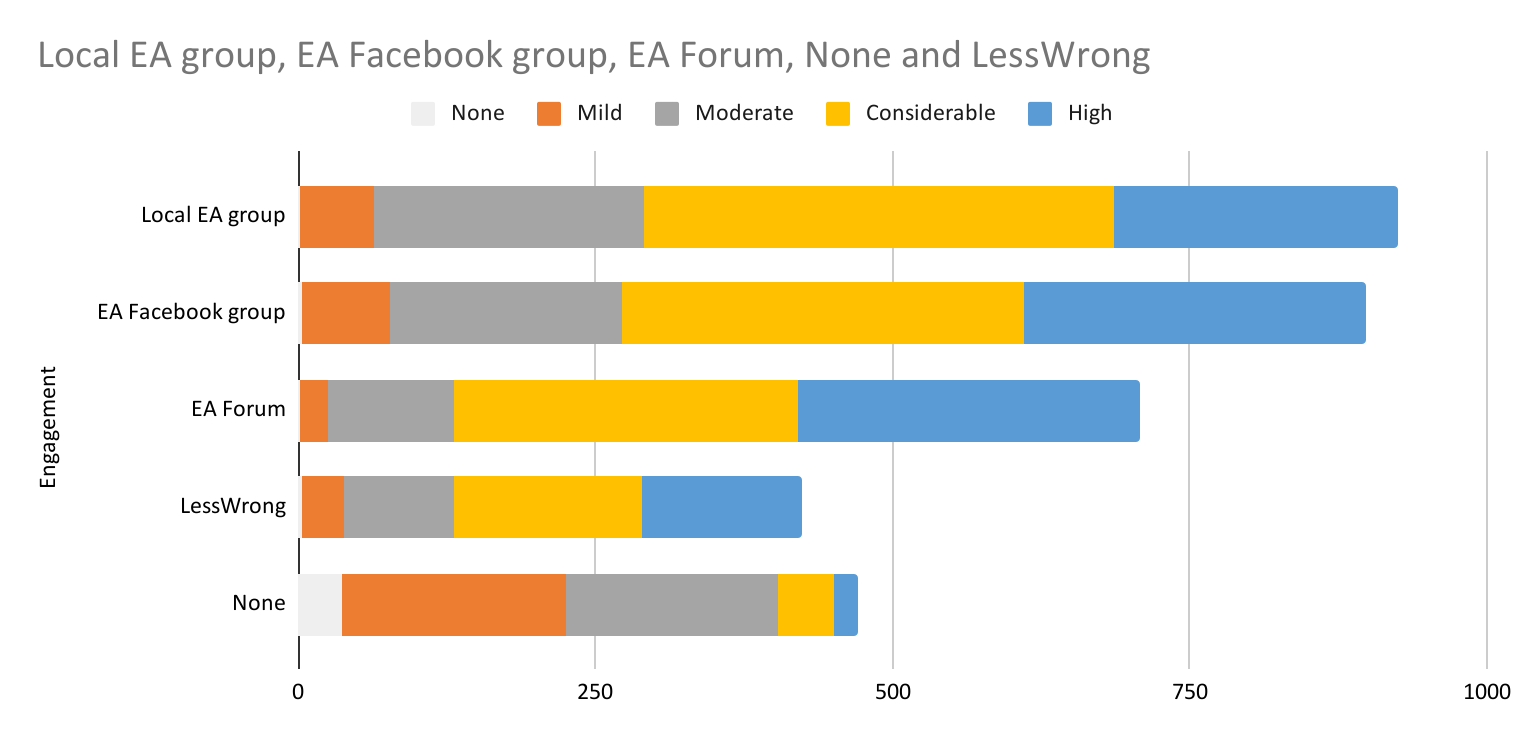
Activities and self-reported engagement
We see a clear increase in the percentage of respondents who have completed different activities as we move up the self-reported engagement levels.
Between levels 1-3 in self-reported engagement, no more than ~25% of respondents report changing their career based on EA principles or donating >10%, with even fewer undertaking most other activities. Conversely, among the most engaged, the majority of respondents have taken the Giving What We Can Pledge, lead an EA group and work(ed) for an EA org.
The relationship with self-reported engagement varies for different activities however. Being a subscriber to the 80,000 Hours newsletter is one of the most commonly reported activities and is comparatively flat across engagement levels (although it still increases from 30% among those reporting the least engagement to 72% among the most engaged). Some of the activities increase fairly steadily across engagement levels, for example, donating >10% or changing career based on EA principles. Conversely, others have very sharp inflection points. For example, leading an EA group is very uncommon among EAs reporting engagement levels 1-3, but dramatically increases in the higher engagement levels. Similarly, working at an EA org is very uncommon in all but the highest engagement levels. As we discussed in 2019, some of this corresponds to the explicit examples that were included to characterise different engagement levels.
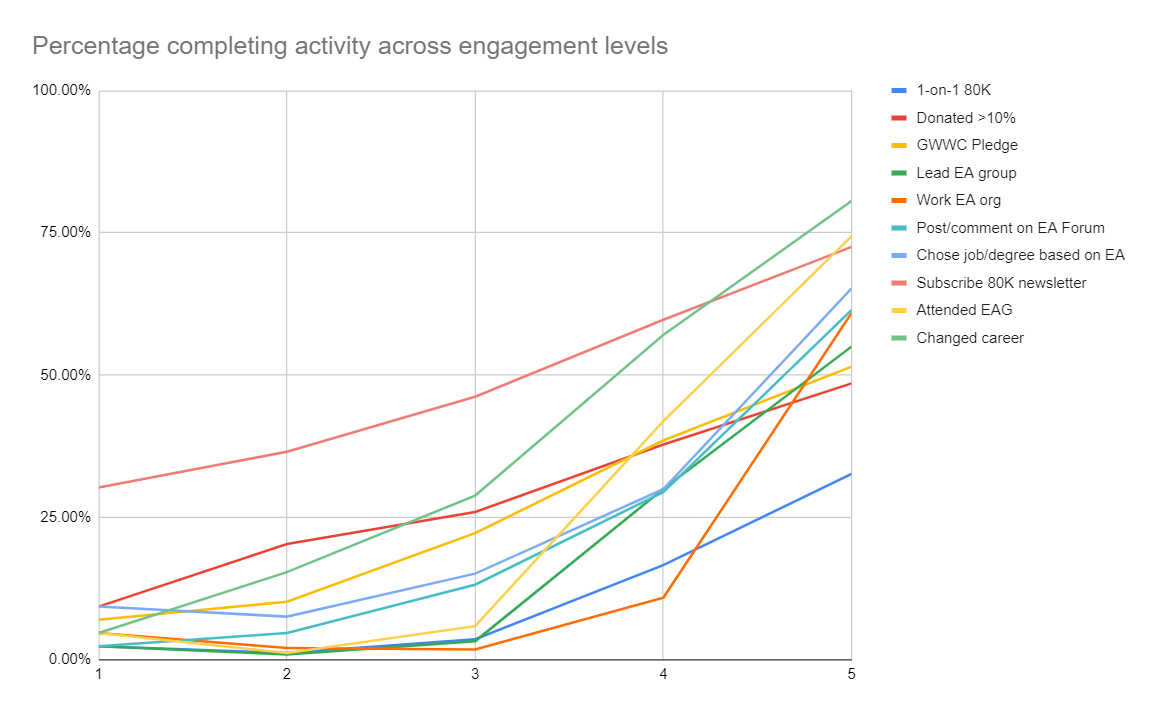
Multiple Correspondence Analysis
We conducted a multiple correspondence analysis, as we did in 2019 (see discussion of the 2019 analysis here), to assess the relationship between different responses (that are intuitively related to engagement) and self-reported engagement. We present full information on our 2020 analysis in the supplement linked HERE.
The chart below plots a point cloud of individuals, colour coded for self-reported engagement level, against the positioning of the variables on the first two axes. When we map self-reported engagement on this plot, we find that the first axis is well correlated with variation in engagement. This offers support for the validity of our engagement measures, as self-reported engagement appears to be clearly associated with the various measures of group membership and engagement in EA activities.
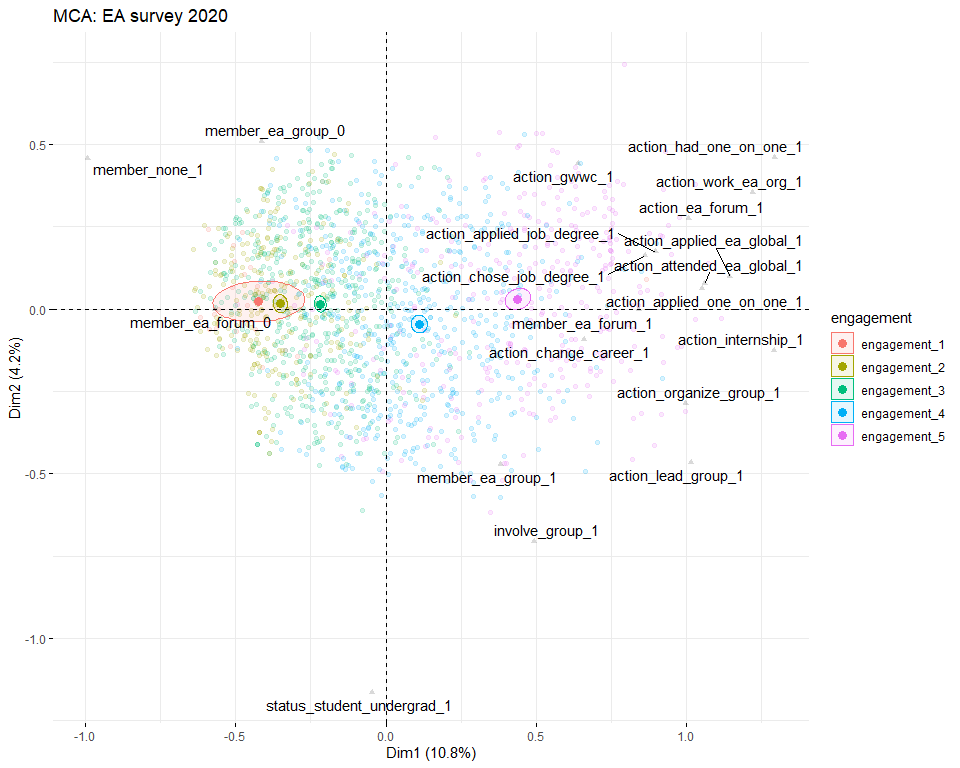
Differences across groups
We consider differences in key characteristics by level of engagement. In the supplement (HERE), we present a numerical table summarizing a key set of socio-demographics, priorities, and behaviors; for each level of self-reported engagement (and overall).
Summary charts
Descriptive measures also help us locate 'where the engaged people are', and compare which groupings are more engaged. Below, we present 'relative frequency stacked bar charts' showing the 'raw' (uncontrolled) relationships between key characteristics and the levels of self-reported engagement. All bars are grouped from 'highest to lowest share with 4-5 engagement', except for age, which is grouped by youngest to oldest. The bars depict 95% confidence intervals for the 'shares of each subgroup with 4-5 engagement', as well as the narrower 1-standard-error bars.
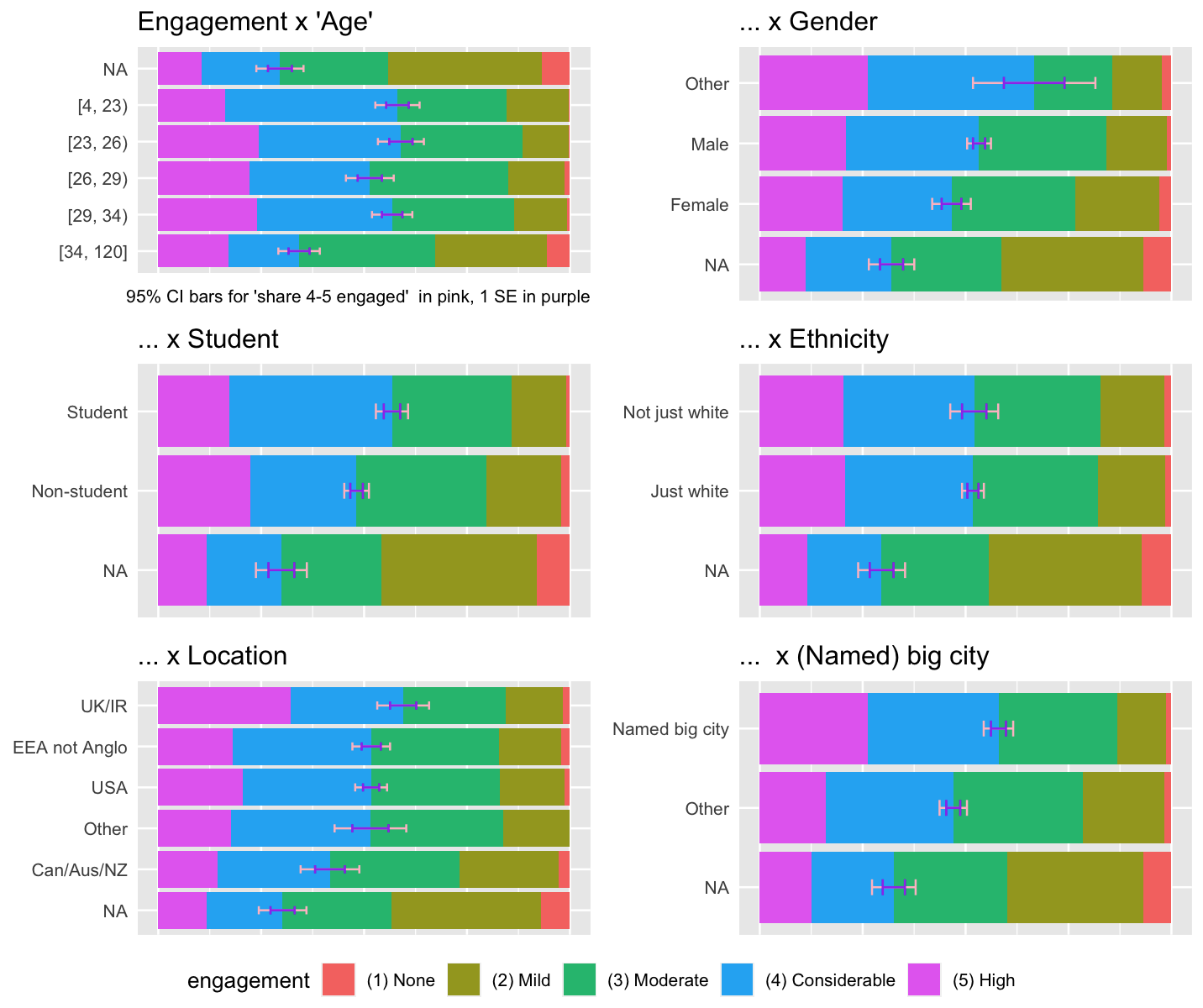
Examining the proportion of respondents who self-identified as levels 1-3 vs 4-5 in terms of engagement, we observe:
- Those >34 years of age reported being less engaged
- Male respondents reported being more engaged
- Students reported being more engaged
- Those living in one of the 22 big cities named in the survey[2] reported being more engaged
- In terms of country of residence groupings, UK + Ireland were the most engaged, and Canada + Australia + New Zealand the least engaged.
When people get involved in EA / Time in EA / Age / Age When First Involved in EA
We examine the relationship between the year when people first got involved in EA (which tells us how long they’ve been in EA or their ‘tenure’), their current age and the age they were when they first got involved in EA.
Disentangling the possible influence of each of these is very difficult, as we discuss in more detail in the supplement (subsection “Engagement: age, tenure, period and cohort effects”).
Cohorts / time in EA
We observe a fairly consistent increase in the percentage of respondents who report high engagement in earlier cohorts.
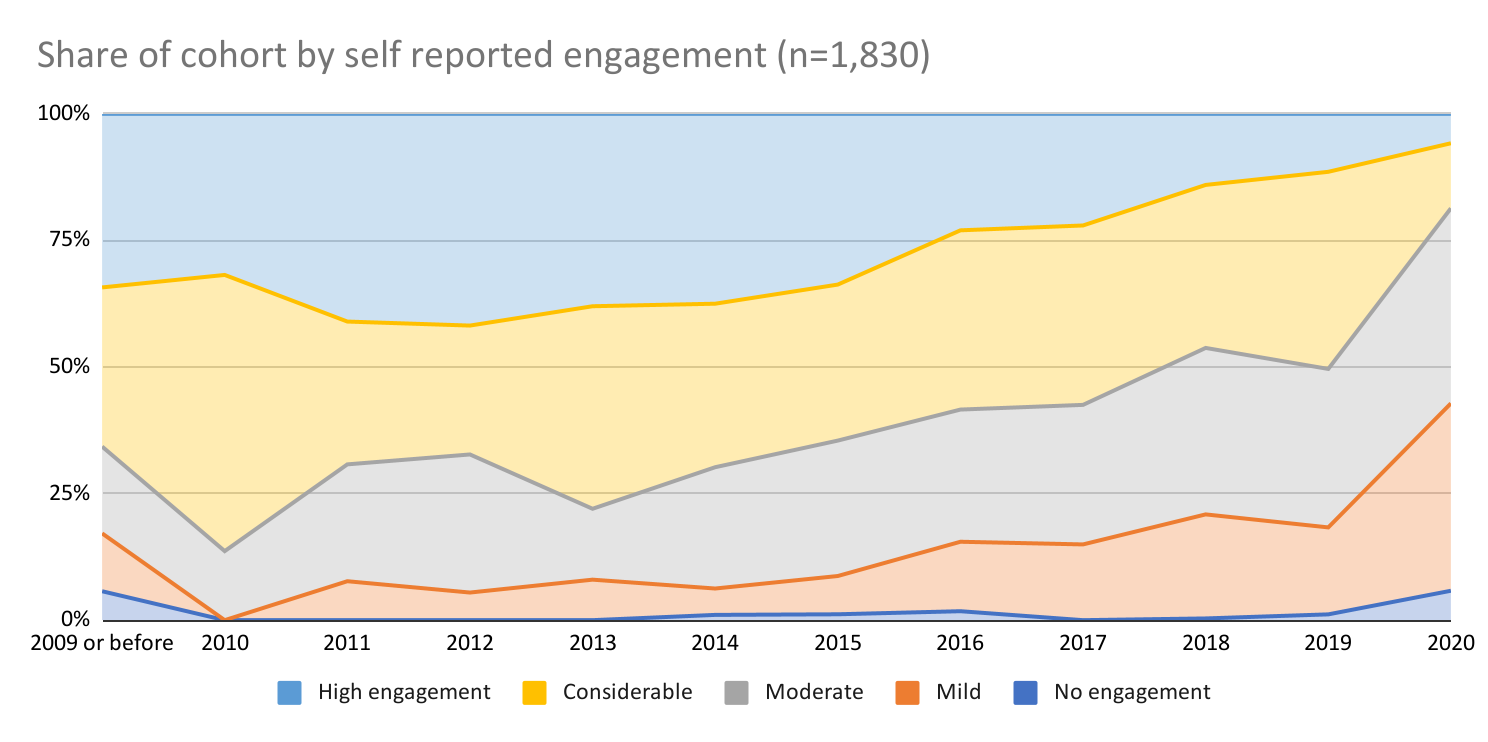
It is worth bearing in mind that this could be explained either by
- people getting more engaged the longer they have been in EA and/or
- differential attrition over time (less engaged people drop out, leaving progressively more engaged respondents in earlier cohorts), and/or
- changes in the composition and nature of specific cohorts (e.g. maybe EAs recruited in more recent cohorts are, on average, disposed to be less engaged).
It’s also worth noting that there is a particularly marked drop in the percentage of respondents who identify with levels 1-3 in terms of engagement between the 2020 and 2019 cohorts. This could be taken to suggest that the first year in EA is most important for people either (quickly) becoming more engaged or dropping out. However, we observe that this ‘drop’ is quite a bit steeper than what we observed in 2019 (the overall pattern is otherwise similar- note that the two graphs are inverted), so we suspect this may largely be an artifact of the fact that we recruited a particularly large share of respondents who had only joined EA in 2020, in this survey.
We also plot the relationship between tenure and engagement, for different age groups. Engagement mainly seems to increase in tenure for all age ranges (the ‘dip’ for the 26-29 age range has very wide confidence intervals and appears driven by a few observations). The slope appears particularly positive in the first few years.
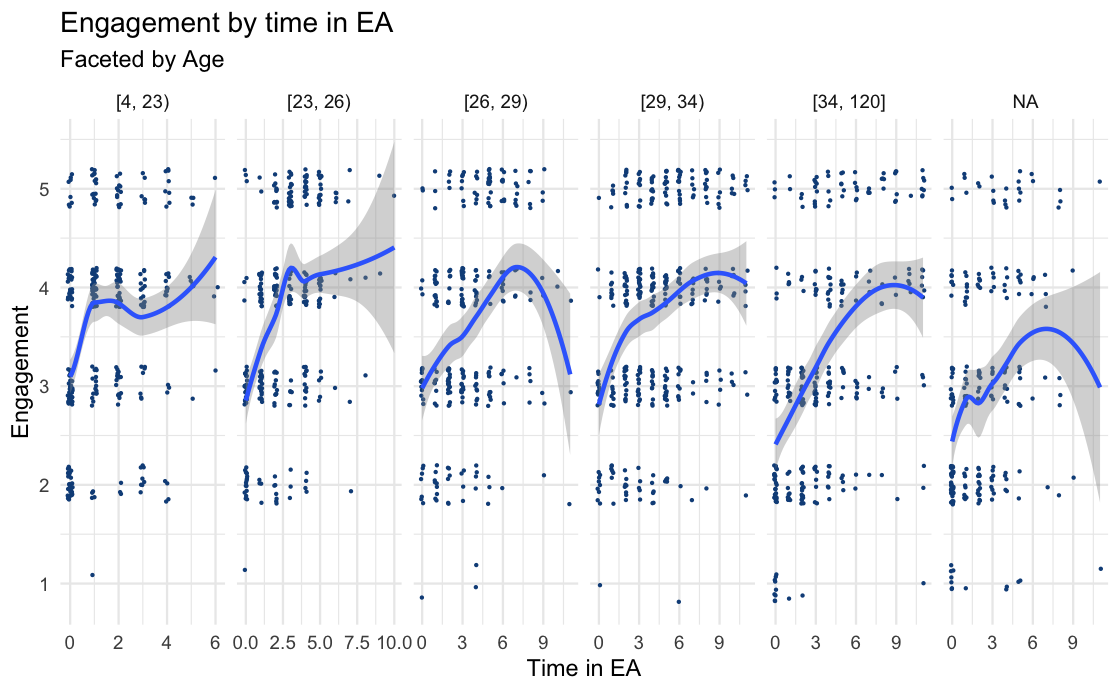
Age
Looking at the average level of engagement of EAs of different ages, we observe a non-linear trend, with engagement increasing until the mid-to-late 20s and then declining. However, this should be expected to be strongly confounded by time-in-EA (or ‘tenure’), as EAs who have been in longer might be expected to be both older and more engaged than those who have joined EA more recently.
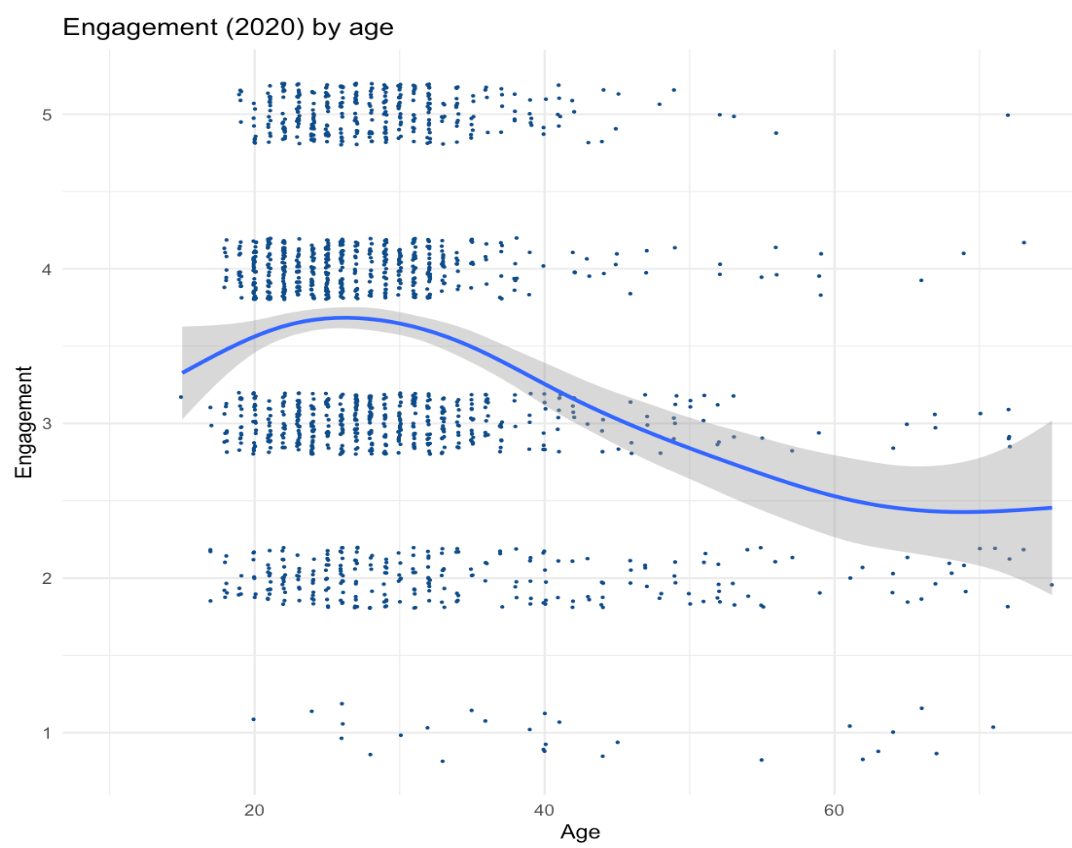
We confirm this with the graph below, showing that tenure seems to increase steeply with age up until around the age of 30. As such, respondents who are younger than around 30, tend to have been in EA for a shorter length of time.
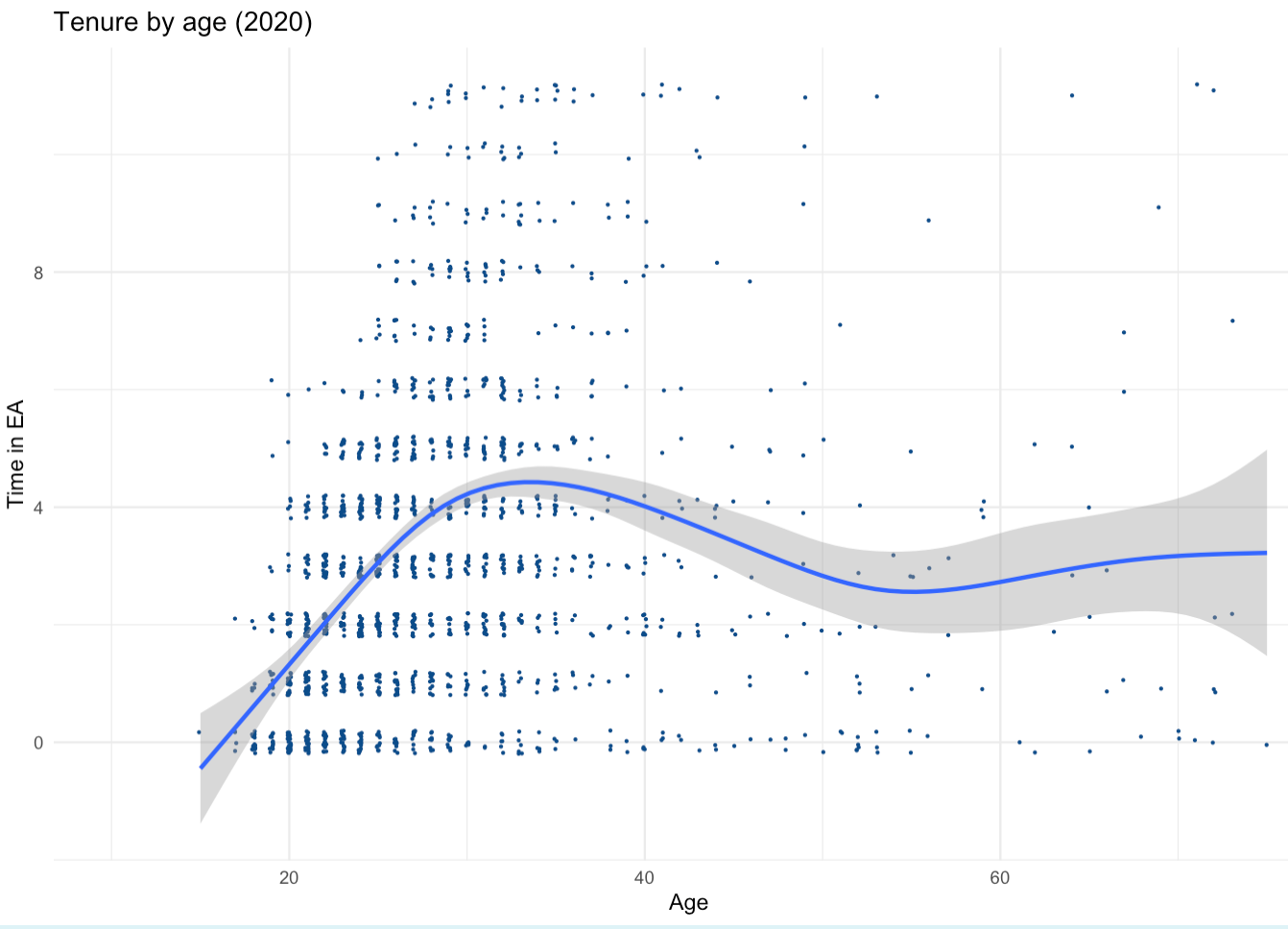
Given this, we also plot the relationship between age and average engagement for different groups of cohorts (0-1, 1-2, 2-3, 3-5 and 5-11 years in EA) below. Here we still see a pattern of average engagement sharply increasing and then declining with increasing age for all groups. Moreover, we can now observe that the peak is now at slightly younger ages: at around 20 for the most recent cohorts and getting commensurately slightly older with each successively earlier set of cohorts.
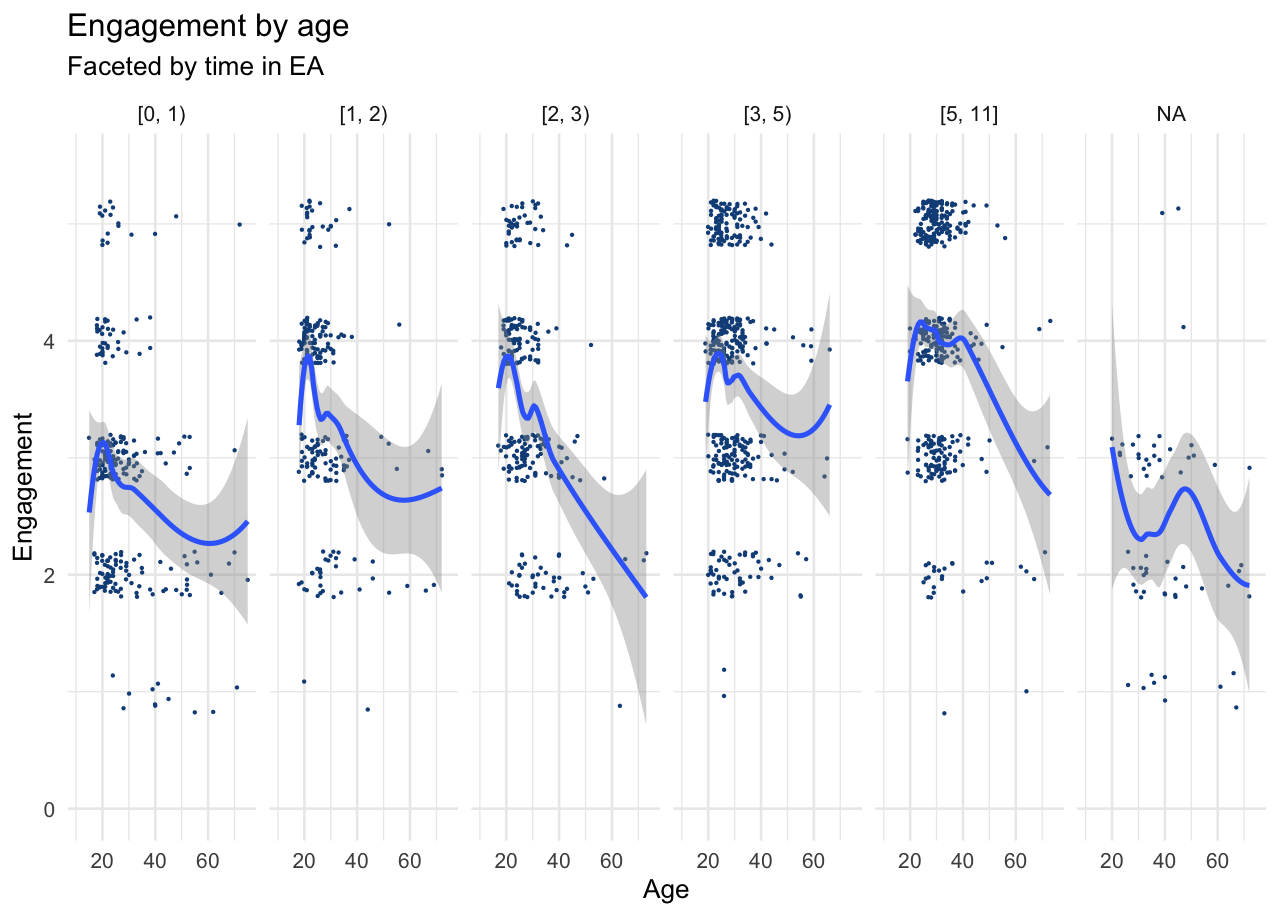
Age when first involved in EA
Our variable for when people first got involved in EA is simply estimated from their reported birth year (from which we calculate their age) and the reported year when they first got involved in EA. The possibility of a relationship between the age when people first get involved in or hear about EA has been previously discussed here.
We observe that within each group of cohorts, engagement seems to peak around 20, and then decline with further age (rather than peaking progressively later, for earlier cohorts, as was the case for current age).
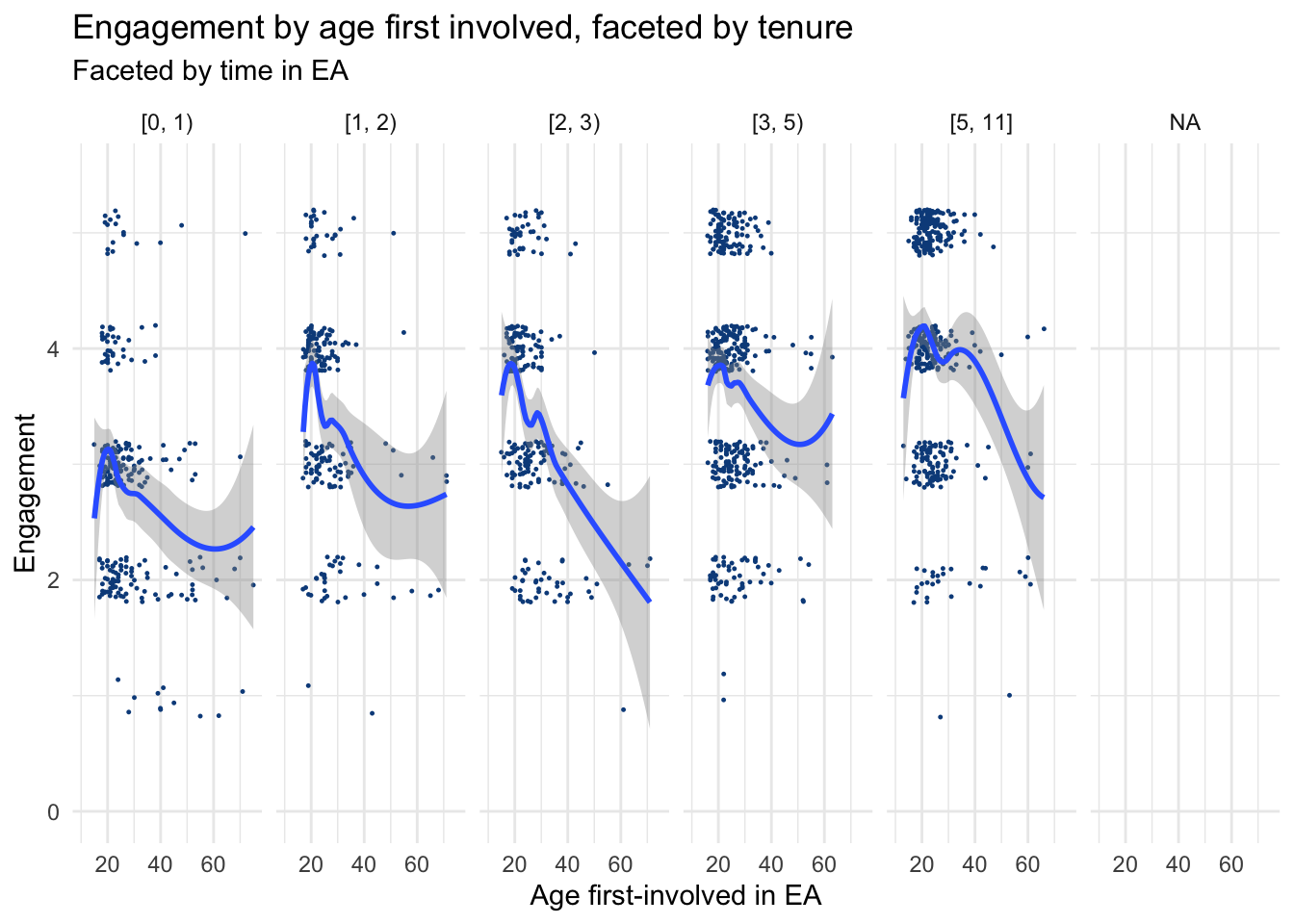
Gender and self-reported engagement
We observe slightly higher percentages of male respondents selecting the higher engagement levels. Using an ordered logistic regression we find that males are 1.25 times more likely to report a higher level of engagement than not males.[3]
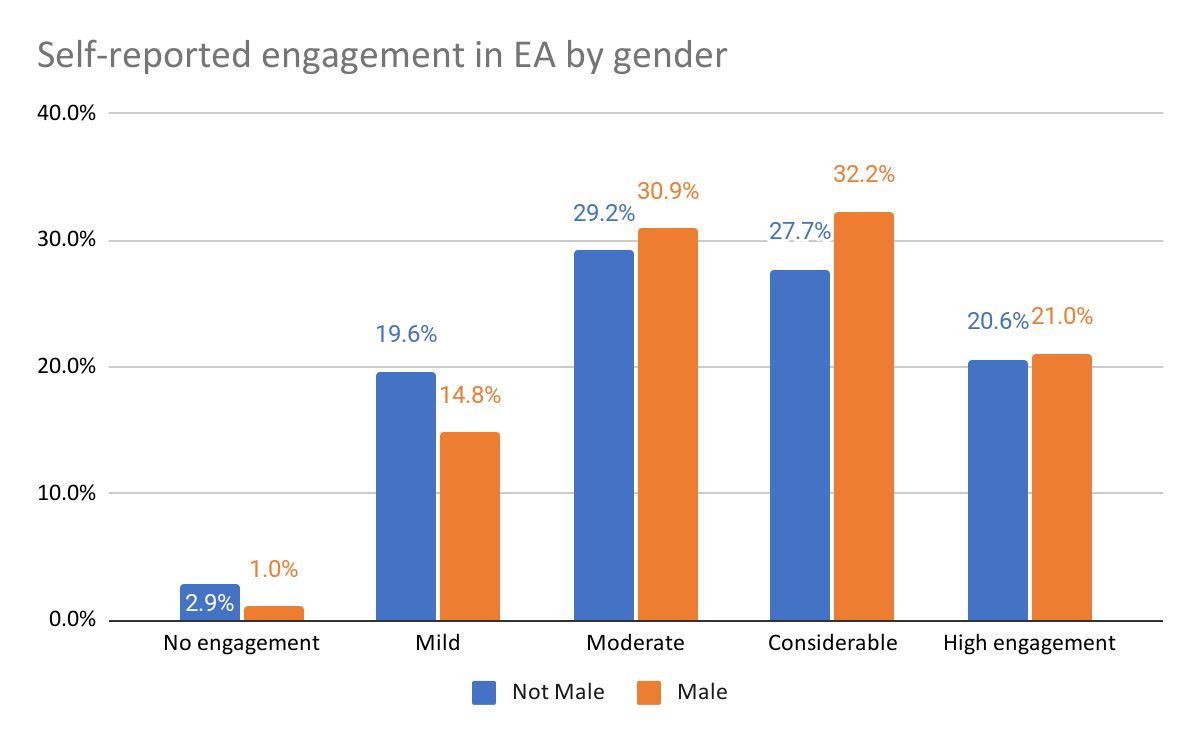
Gender and group membership
We see significant differences in membership in the EA Forum and LessWrong by gender, where men are more likely to be members of each group than non-males. The association by gender for those involved in “none of the above” is also significant, with males being more likely to belong to at least one of the groups listed.
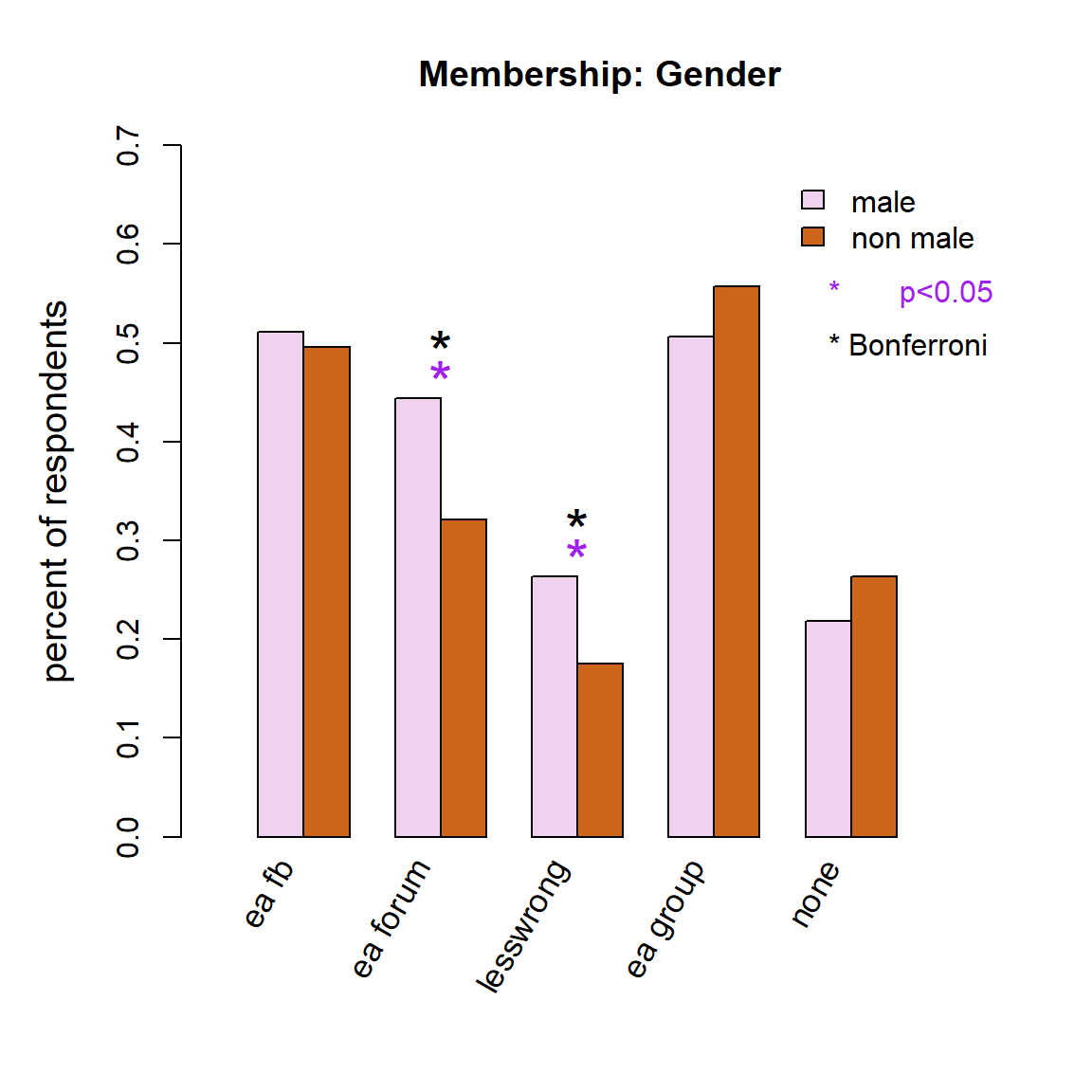
Gender and Activities
There are significant associations between gender and actions taken in the last year. Men in our sample were more likely to have reported donating >10% of their income, taking the GWWC pledge, or applying for 1-on-1 coaching with 80,000 Hours.
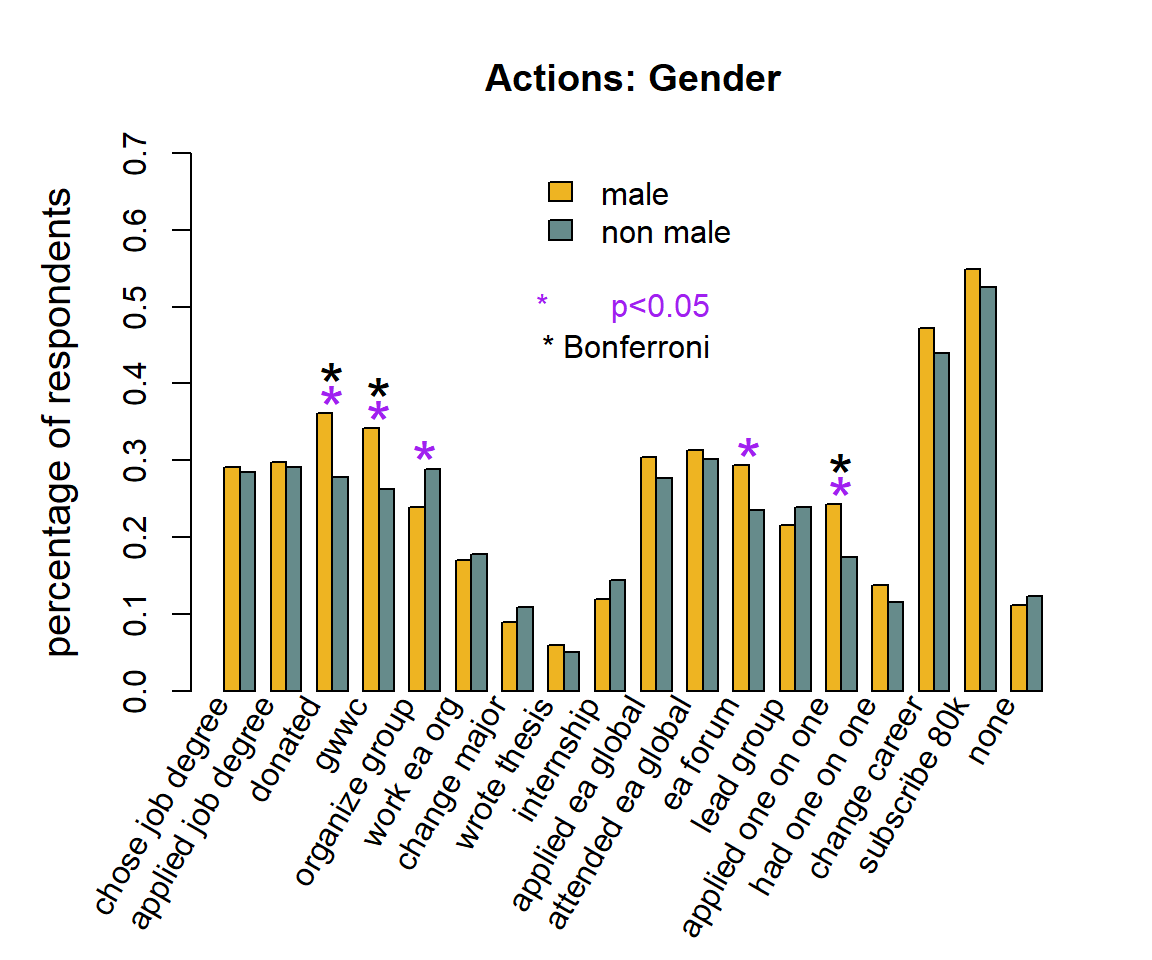
Race and group membership
We find that not white only respondents were more likely to have reported being a member of the EA Facebook group.
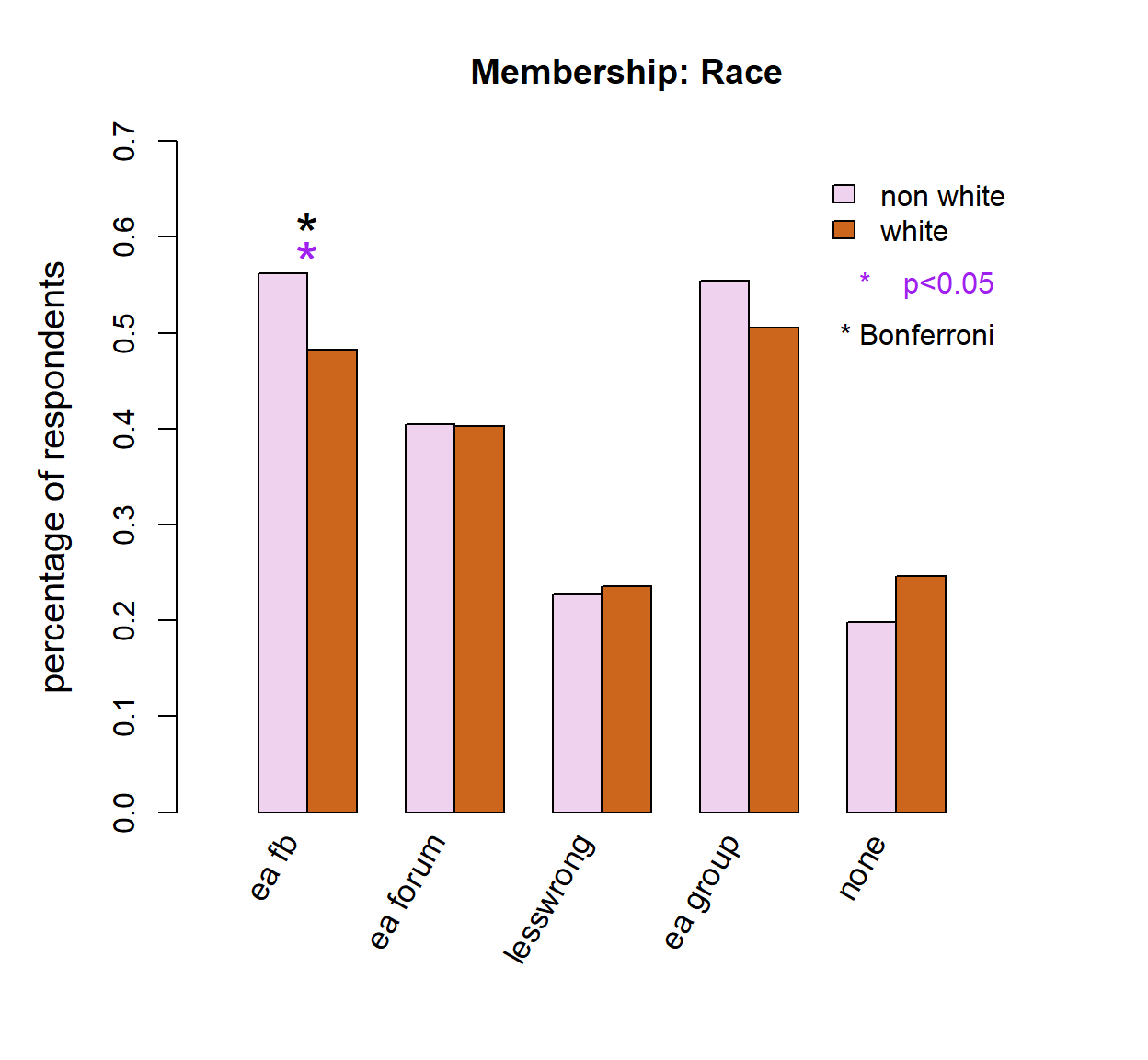
Race and activities
Respondents that identified their race as white only were more likely to have reported donating >10% of their income.
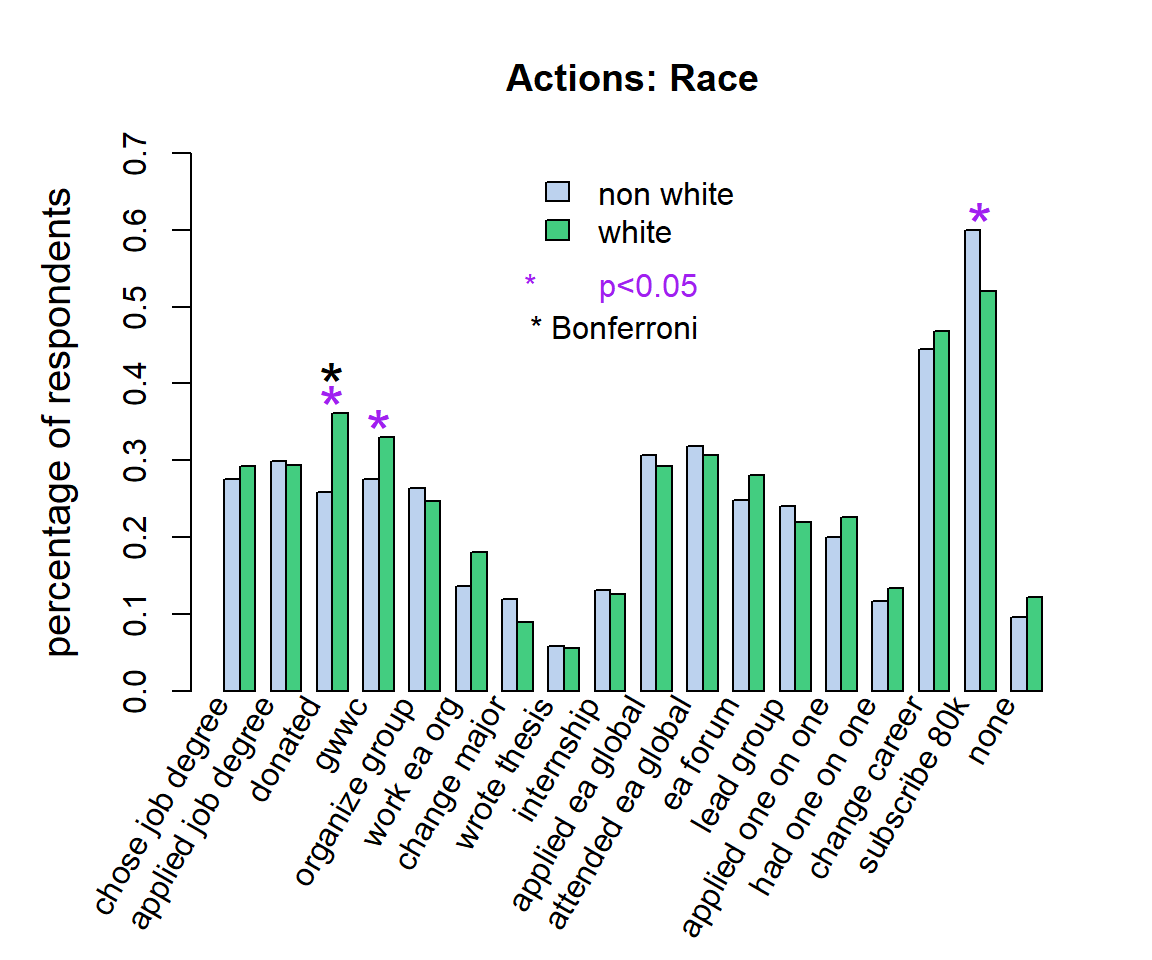
Where people first heard of EA
We analysed differences in engagement between respondents who reported first hearing about EA from different sources in this earlier post. For a quick reference, we give a breakdown of engagement by groups of 'where first heard of EA'below (this is a simpler version of a chart presented in "How EAs get involved in EA").
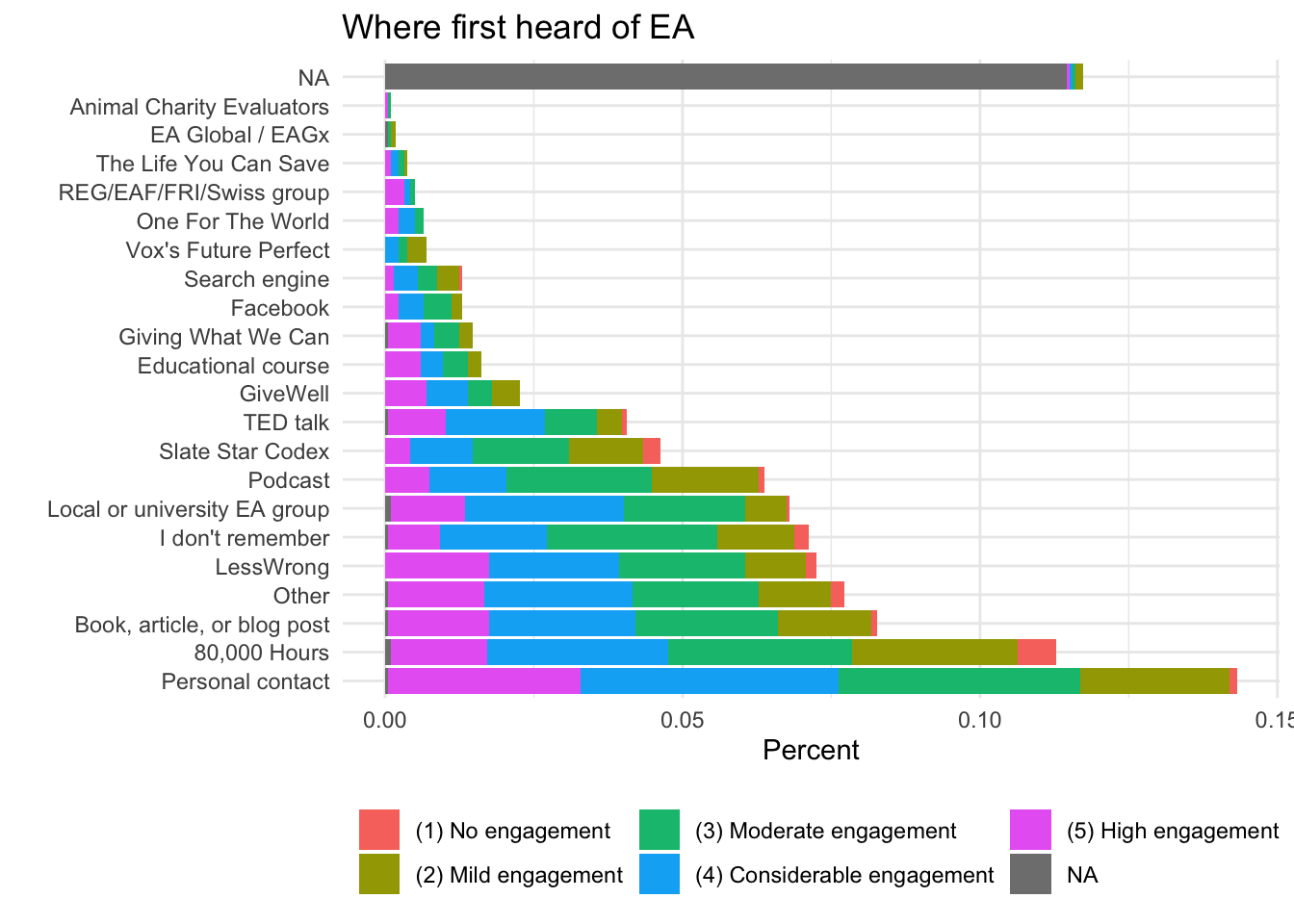
Modeling engagement
Descriptive modeling
There are several reasons that we may want to better understand “what is associated with engagement holding other things constant"? We discuss and model this in more detail in our public supplementary chapter (see ‘"Why do we care about engagement" and "Modeling Engagement").
Predictive (and causal) modeling
In addition to description, we are also interested in more ambitious predictive and even (at least suggestively) causal modeling of engagement for several reasons, also mentioned in previous posts. (We discuss our modeling goals and considerations, and choice of variables/features, in the supplement here and here.)
Models of self-reported engagement level; results
We consider the association between a range of features and their level of engagement. Again, these should not necessarily be interpreted as causal relationships; rather they might be seen as descriptive and, in some cases, predictive.
We focus on an ordered logit model, reporting the odds ratios. As noted in our 'community information' post, these can be loosely interpreted as the "relative probability of stating a 1 unit higher level of satisfaction", relative to the base group.[4] Note that the ordered logit model imposes a particular functional form: a variable must have proportional relative 'effect' on the probability of being in a higher versus a lower category, with this proportional 'effect' being the same between any comparison of adjacent categories. However, a strong justification can be made that this is 'more realistic' than a linear model, which interprets the 1-5 engagement self report as if it were a meaningful cardinal outcome. We present a linear model, for comparison, in the supplement here.
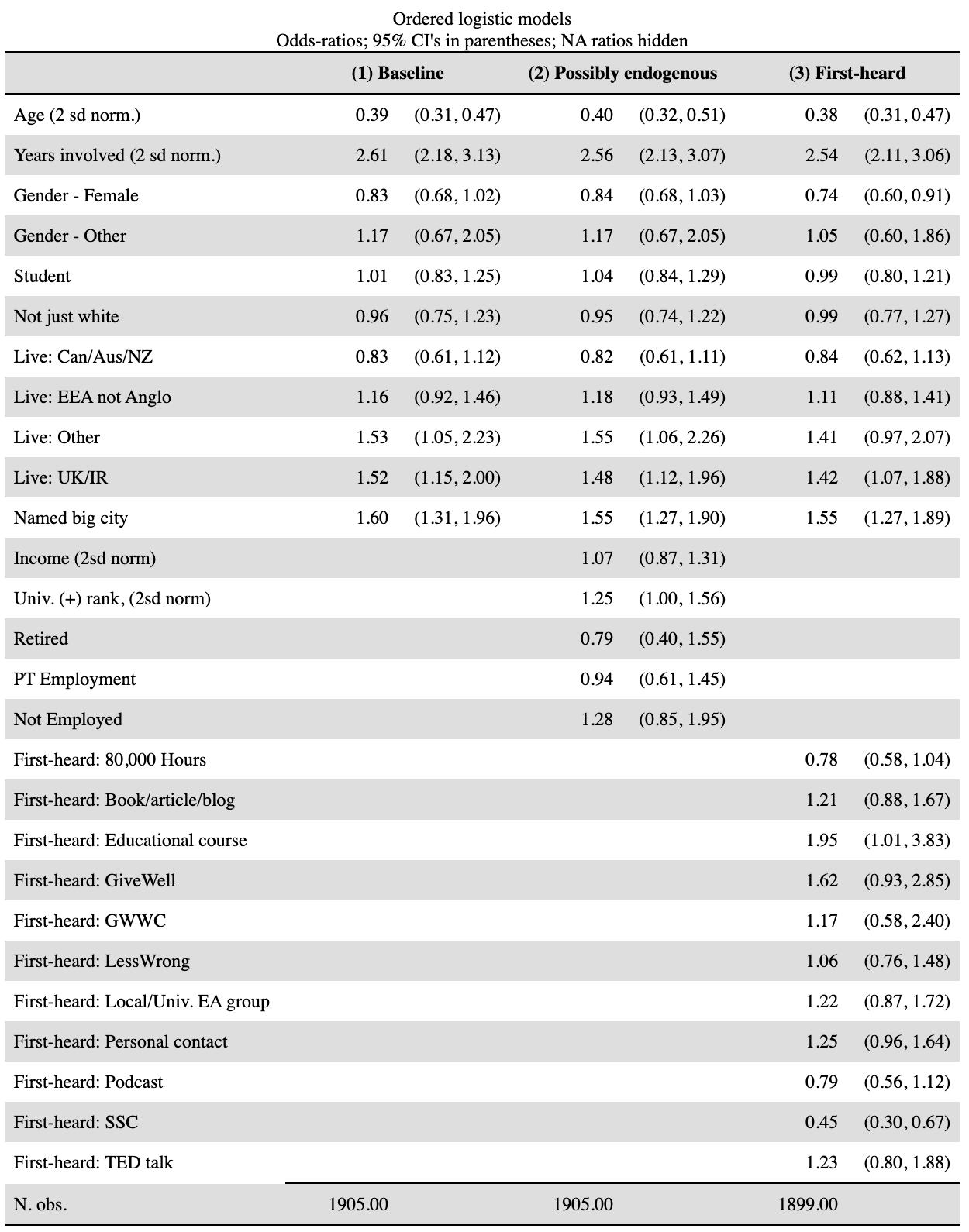
In the Ordered Logit models summarized in the above table, continuous variables (income, age, university rank) are de-meaned and divided by two standard-deviations.[5]
Base groups are set as the most numerous group in each category. Thus, all dummy coefficients represent adjustments from the 'overall base group': an individual who is Male, works full-time, 'Just White' ethnicity, USA-based, not based in a (survey-named) big city, and ‘first heard of EA’ response is 'other or don't remember'.
The above models include dummy variable 'controls' for nonresponses to each of the given categorical variables. These coefficients are hidden in the above table, but shown further down.
We next present a forest plot of the coefficients from the baseline model, considering only ex-ante factors (with imputation):[6]
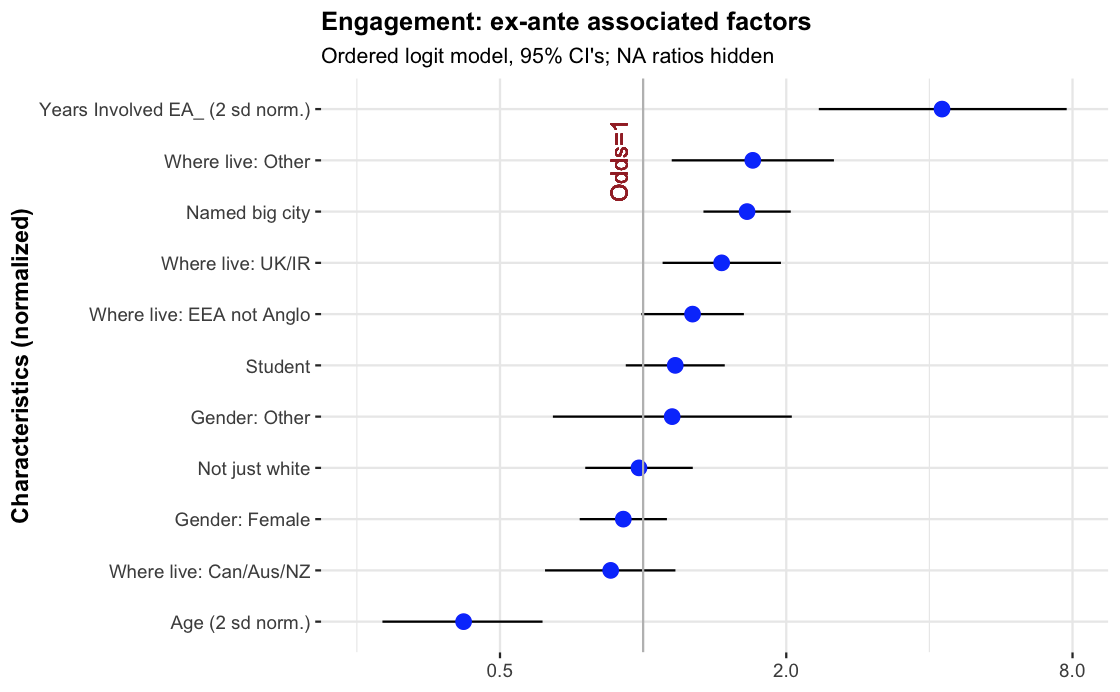
Next, we present a similar forest plot of the model that includes some characteristics that may not be strictly ex-ante (model 2 in the first table):
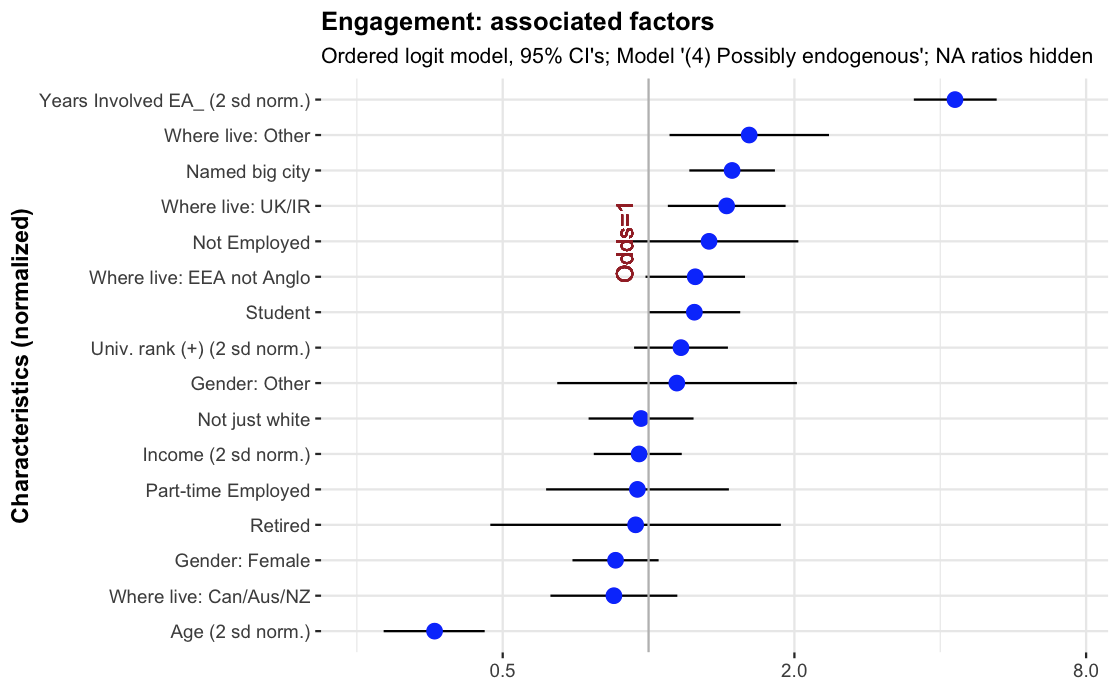
Nonresponse: The table below presents the coefficients from a selection of the above models, on the 'lack of a response to' (i.e., skipping) certain questions.
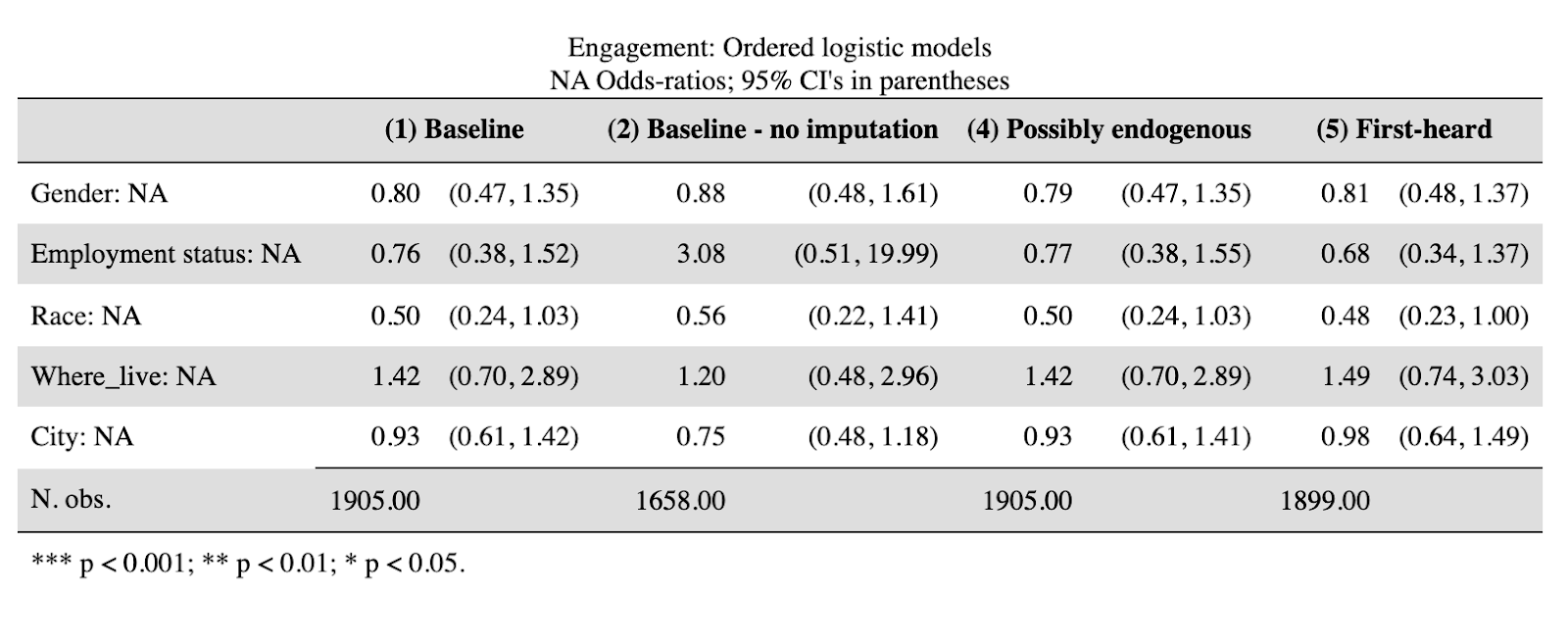
This nonresponse seems to largely be associated with less engagement, particularly for the race/ethnicity question. However, non-response to the 'location' question seems to be an exception, perhaps because the base group, USA-residence, tends to be lower-engagement.
Interpretation, robustness, further exploration
We next present a series of similar models to delve more deeply into what is driving the above, and test robustness.[7]
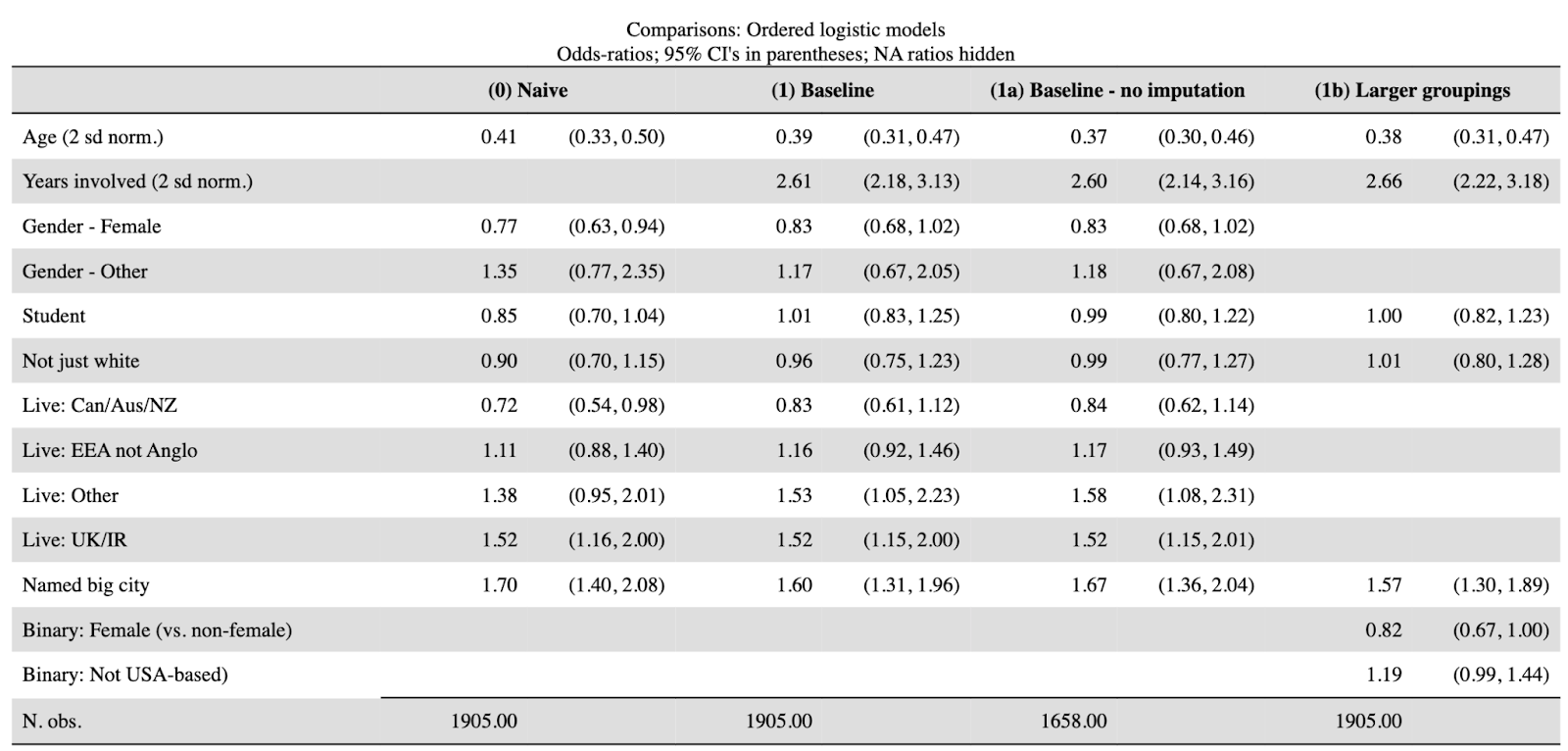
Column (0) presents a 'naive' model that does not control for time-in-EA. This model may be helpful as a description of the engagement levels of those currently in EA. However, it is confounded with differences that are driven by the changing nature of recruitment into the movement, as is not likely to be predictive (nor causal). Nonetheless, we mostly do not see strong differences from our baseline model, presented (again) in column (1). This does seem to impact the odds-ratio for 'Students'. The naive model suggests students are less engaged, while the baseline model suggests they are engaged at about the same rate as others, given their (typically shorter) tenure in EA.[8]
Column (1a) repeats the base model, but dropping (rather than imputing) participants that did not respond to the age or 'years involved' questions (note the smaller number of observations). These results are very close to the base model.[9]
Column (1b) adjusts the baseline model to use larger groupings. The results here suggest that those based in the USA are less engaged, controlling for other factors. (Other results are similar to previously-reported columns).
Further controls for age/tenure:
In the dynamic document (here - see "Engagement: ex-ante associated factors, robust controls") we present a similar model with a robust set of controls for age and tenure – in addition to the linear controls for age and tenure we include controls for five age-range groups and four time-in-ea-range groups. The results for the remaining variables are nearly identical, suggesting that these findings are not particularly sensitive to the functional form used to control for age and time in EA.
These models are only able to “explain” a moderate share of the variation in engagement (but note we did not choose the models parameters to maximize this. The pseudo-r-sq for above models (Nagelkerke method) is 0.17 and 0.15 for the "(1) Baseline" and "(2) Baseline - no imputation", respectively. The comparable statistic for most of the other models is close to that of model (1).
**Selection and differential attrition: focus on recent (2017-2019) recruits **
We previously discussed how differential selection and attrition could drive the above models to suggest unwarranted predictions (with further discussion here, see ‘differential attrition issue’). We thus present a model isolating just those who say they became involved in 2019, 2018, or 2017. This includes most of 'those who have been in EA less than the average duration.' However, it excludes those who joined in the survey year itself (2020), as those in their first year may be less able to gauge their own engagement. We might suppose that the differential selection is less substantial over this shorter range of tenures. We might also imagine that patterns among 'newer recruits' are likely to be more reflective of future patterns. If so, the results below might be more predictive of engagement levels, and more useful for certain 'policy choices.'
As we are only considering three such tenure durations, we include a dummy for each 'year first involved'. Given the short tenure, we also do not anticipate that income or university rank could be particularly affected by EA engagement at this point; thus we focus on the larger set of features without worrying about endogeneity. Below, we plot the coefficients from the model (similar to the ones above) for the 807 EAs who became involved between 2017-19.
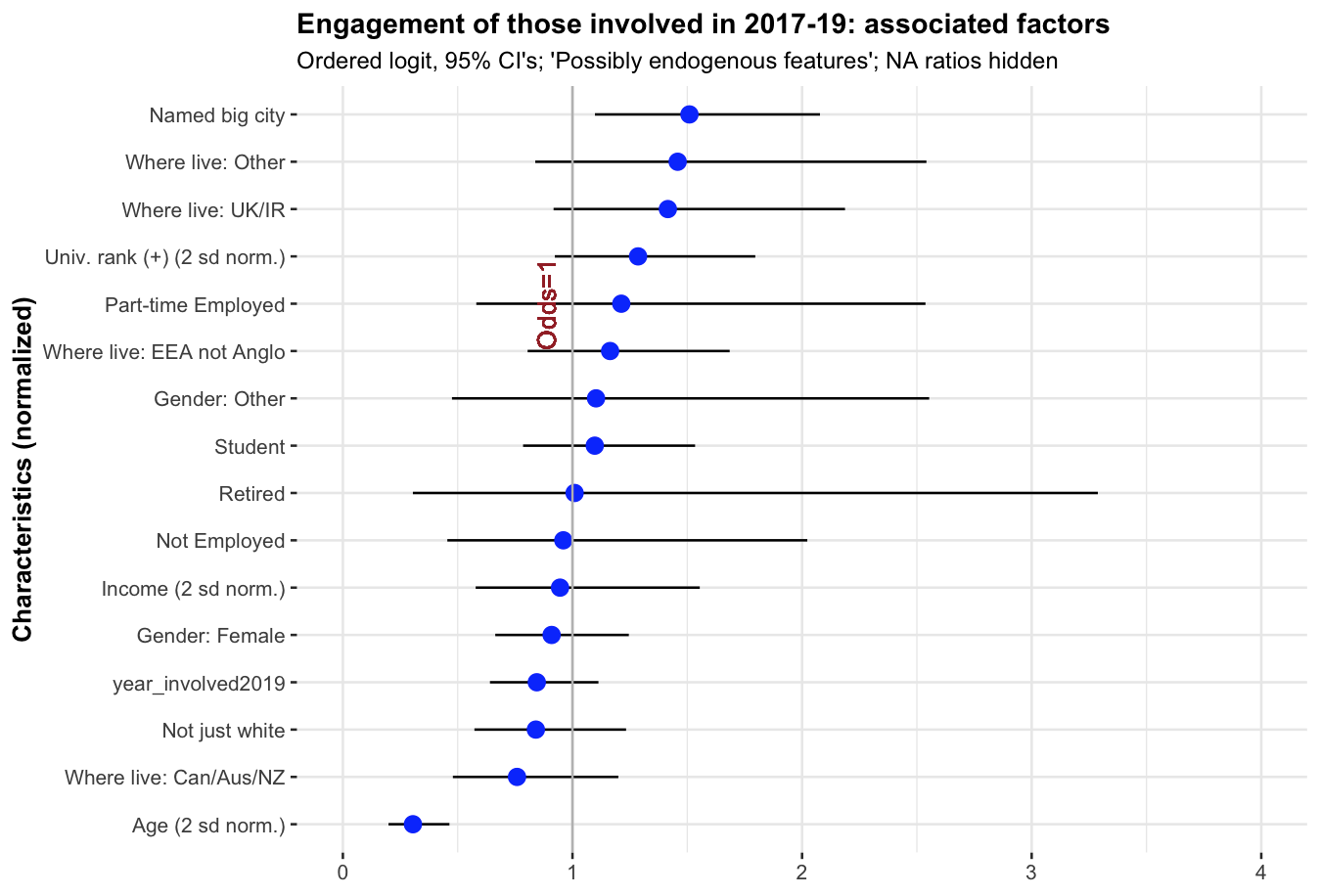
Making inferences from the above graph, for those joining in 2017-19, we see greater self-reported engagement among[10]:
- Those located in one of the big cities named in the survey
- UK and Ireland (and less so, the EEA)
- Non-'western' countries
- Those who attended high-ranked universities
- Students
- Younger people (the negative of "Age")
(The above list is reported in approximate order of the magnitudes of these odds, also considering the tightness of the confidence bounds.)
We see less engagement for those living in Canada, Australia, or New Zealand, relative to the base group. (The base group, the USA, is also marginally less engaged than the average for other countries; see other models).
Conclusions and Future work
This post has presented a descriptive picture of the ways EAs engage, how they rate their own engagement, and how these relate to other attitudes and observable characteristics. This broadly aids our understanding of "what engagement in the EA movement looks like” and “who/what/where" EA’s engage.
Summarizing some of our findings
- Self-reported engagement is strongly associated with membership in EA groups and with taking more EA-relevant actions, arguing for the validity of this measure
- Engagement tends to decrease in age after age-20 but increase with time-in-EA
- People reporting that they first heard of EA from certain sources (such as Educational Courses, and Personal Contact tend to have higher engagement than average, even after controlling for factors like age and time-in-EA
- Those living in one of the big cities we name in the survey (EA Hubs), as well as certain geographic regions (e.g., UK/Ireland) tend to have higher engagement than average, even after controlling for other factors
However, given our data is retrospective, many of these differences could reflect differential attrition processes between groups, as discussed above.
In addition to description, we are also interested in more ambitious predictive and even causal modeling of engagement for several reasons. Key questions include
- Which groups are more or less likely to become engaged in EA?
- What approaches lead people to become more involved in EA?
- How is the EA movement (and in particular, the highly engaged EA population) likely to grow over time, and potential proxies for engagement can predict this? This could inform our plans for building infrastructure, as well as our impact strategies and approaches.
We suspect that more in-depth analysis of these issues is warranted, and we hope to pursue this going forward. For example:,
- We are working to bring together the EA survey data across years, allowing us to separate “time in EA” from “year joined EA”, and perform “Age-Period-Cohort” analysis.
- Considering “movement growth”: We hope to combine this with medium-term prediction models explicitly quantifying our uncertainty into probabilistic forecasts of key outcomes.
- We aim to build (ML) models to predict engagement outcomes from initially-observable characteristics.
- In particular, we could utilise initial responses from earlier EAS data to predict presence in later surveys, as one measure of engagement.
We expect causal inference, learning what approaches would “make people become more involved”, will be more difficult. Key explanatory variables are entangled, and were not ‘experimentally assigned’: the ways that people are introduced to EA, the groups they are associated with, their experiences, and the ways that EA groups and organizations interact with members, etc. Nonetheless, further analysis, using techniques from the fields of causal inference, statistics, and econometrics, may allow us to make some reasonable causal inferences.

The annual EA Survey is a project of Rethink Priorities. This post was written by David Moss, Jacob Schmiess and David Reinstein. Thanks to Peter Hurford, Ben Todd and Ben West for comments.
We would also like to express our appreciation to the Centre for Effective Altruism for supporting our work. Thanks also to everyone who took and shared the survey.
If you like our work, please consider subscribing to our newsletter. You can see all our work to date here.
Notes
x2(df=4,n=4032) = 30.93, p=<0.000001. Notably, despite the change in the overall pattern of responses, the average level of engagement is very similar (mean 3.41 vs 3.45). ↩︎
These were cities known to have relatively high EA populations in previous EA Surveys: Amsterdam, Auckland, Berlin, Boston / Cambridge (USA), Cambridge (UK) , Canberra, Chicago, London Los Angeles Melbourne, New York City, Oslo, Oxford, Philadelphia, Seattle, SF Bay Area, Stockholm, Sydney, Toronto, Vienna, Washington DC, Zürich. ↩︎
Prob>chi2=0.0266, p>|z| 0.027 ↩︎
e.g., if the coefficient for 'jazzy' is 1.5, this means that, controlling for everything else in the model, comparing any two levels of engagement (2 versus 1, 5 versus 4, etc) jazzy people are approximately 50% more likely to be in the higher group. ↩︎
This is for comparability to dummy-coded categorical variables, see Gelman, 2007. Where these are missing they are coded as zero, the mean of the transformed variable. ↩︎
It is important to note that these are in multivariable models. Thus, while the odds-ratio shifter for 'Where live: Other' is among the most positive, this does not imply that the most-engaged EAs are necessarily in these other countries. ↩︎
For further robustness checks considering functional form, see the linear models presented in the dynamic document [HERE]. ↩︎
The control for 'years in EA' also had a more substantial effect on the coefficients of the different 'first-heard' coefficients in column (3) of the previous table. ↩︎
We also ran a comparable model that dropped all cases with missing values for any of the variables in this mode. Again, the results (available by request) are largely similar. ↩︎
As some of the confidence intervals for these odds ratios cross the threshold of unit (even) odds, these are not all statistically significant in a conventional sense. Nonetheless, one might reasonably update beliefs in this direction. ↩︎