Update 2022: Looking back on younger Remmelt's writing on decision-making, it is useful but cocky. It explained what I now call 'monitoring values' for carving out states of the world to decide between, but not how choice arises from 'deep embedded values'.
---
This post contains:
1. an exposition of a high-level model
2. some claims on what this might mean strategically for the EA community
Effective Altruism is challenging. Some considerations require you to zoom out to take an eagle-eye view across a vast landscape of possibility (e.g. to research moral uncertainty), while other considerations require you to swoop in to see the details (e.g. to welcome someone new). The distance from which you're looking down at a problem is the construal level you're thinking at.
People involved in the EA community can gain a lot from improving their grasp of construal levels – the levels they or others naturally incline towards and even the level they're operating at any given moment (leading, for instance, to less disconnect in conversations). A lack of construal-level sense combined with a lack of how-to-interact-in-large-social-networks sense has left a major blindspot in how we collectively make decisions, in my view.
The Values-to-Actions Decision Chain (in short: 'decision chain') is an approach for you to start solving the awe-inspiring problem of 'doing the most good' by splitting it into a series of decisions you will make from high to low construal levels (creating in effect, a hierarchy of goals). It is also a lens through which you can more clearly see your own limits to doing this and compensate by coordinating better with others. Beware though that it’s a blurry and distorted lens – this post itself is a high construal-level exercise and many important nuances have been eliminated in the process. I'd be unsurprised if I ended up revising many of the ideas and implications in here after another year of thinking.
Chain up
To illustrate how to use the V2ADC (see diagram below):
Suppose an ethics professor decided that...
- ...from the point of view of the universe... (meta-ethics)
- ...hedonistic utilitarianism made sense... (moral theories)
- ...and that therefore humans in developing countries should not suffer unnecessarily... (worldview)
- ...leading him to work on reducing global poverty... (focus area)
- ...by reducing a variety of easily treatable diseases... (problems)
- ...by advocating for citizens of rich countries to pledge 1% of their income... (intervention)
- ...by starting a non-profit... (project)
- ...where he works with a team of staff members... (workflow)
- ...to prepare his TED talk... (task batches)
- ...to cover the decision to donate for guide dogs vs. treating trachoma... (task)
- ...which he mentions... (execute)
- ...by vibrating his vocal chords (actuate)
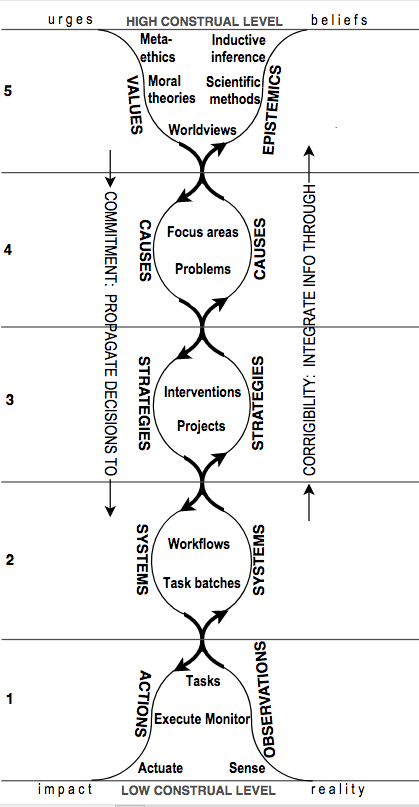
The Values-to-Actions Decision Chain. Note that the categorisation here is somewhat arbitrary and open to interpretation; let me know if something's unclear or should be changed (or if you disagree with the diagram on some fundamental level). Also see the headings on either side of the diagram; it could also be called the Observations-to-Epistemics Integration Chain but that's less catchy.
It should be obvious at this point that the professor isn't some lone ranger battling out to stop world suffering by himself. Along the way, the professor studies classical moral philosophers and works with an executive director, who in turn hires a website developer who creates a donation button, which a donor clicks to donate money to a malaria prevention charity, which the charity's treasurer uses to pay out the salary of a new local worker, who hands out a bednet to a mother of three children, who hangs the net over the bed in the designated way.
It takes a chain of chains to affect those whose lives were originally intended to be improved. As you go down from one chain to the next, the work a person focuses on also gets more concrete (lower construal level).
However, the chain of chains can easily be broken if any person doesn't pay sufficient attention to making decisions at other construal levels (although some are more replaceable than others). For example, if instead of starting a charity, the philosopher decided to be content with voicing his grievances at a lecture, probably little would have come out of it. Or if the executive director or website developer decided that working at any foreign aid charity was fine. Or if the donor decided not to read into the underlying vision of the website and instead donated to a local charity. And so on.
Making the entire operation happen requires tight coordination between numerous people who are able to both skilfully conduct their work, and see the importance of more abstract or concrete work done by others they are interacting with. If instead, each relied on the use of financial and social incentives to motivate others, it would be a most daunting endeavour.
Cross the chasm
Imagine someone visiting an EA meetup for the first time. If the person stepping through the doorway was an academic (or LessWrong addict), they might be thrilled to see a bunch of nerdy people engaging in intellectual discussions. But an activist (or social entrepreneur) stepping in would feel more at home seeing agile project teams energetically typing away at their laptops. Right now, most local EA groups emphasise the former format, I suspect in part due to CEA's deep engagement stance, and in part because it's hard to find volunteer projects that have sufficient direct impact.
If the academic and activist happened to strike up a conversation, both sides could have trouble seeing the value produced by the other, because each is stuck at an entirely different level of abstraction. An organiser could help solve this disconnect by gradually encouraging the activist to go meta (chunking up) and the academic to apply their brilliant insights to real-life problems (chunking down).
As a community builder, I've found that this chasm seems especially wide for newcomers. Although the chasm gets filled up over time, people who've been involved in the EA community for years still encounter it, with some emphasising work on epistemics and values, and others emphasising work to gather data and get things done. The middle area seems neglected (i.e. deciding on the problem, intervention, project, and workflow).
Put simply, EAs tend to emphasise one of two categories:
- Prioritisation (high level/far mode): figuring out in what areas to do work
- Execution (low level/near mode): getting results on the ground
Note: I don't base this on any psychological studies, which seems like the weakest link of this post. I'm curious to get an impartial view from someone well-versed in the literature.
Those who instead appreciate the importance of making decisions across all levels tend to do more good. For example, in my opinion:
- Peter Singer is exceptionally impactful not just because he is an excellent utilitarian philosopher, but because he argues for changes in foreign aid, factory farm practices, and so on.
- Tanya Singh is exceptionally impactful not just because she was excellent at operations at an online shopping site, but because she later applied to work at the Future of Humanity Institute.
Commit & correct
The common thread so far is that you need to link up work done at various construal levels in order to do more good.
To make this more specific:
Individuals who increase their impact the fastest tend to be
- corrigible – quickly integrate new information into their beliefs from observations through to epistemics
- committed – propagate the decisions they make from high-level values down to the actions they take on the ground (put another way: actually pursue the goals they set for themselves)
To put this into pseudomaths:

Where level refers to the level numbers as in the diagram.
Note: This multiplication between levels assumes that if you change decisions on higher levels (e.g. by changing what charity organisation you work at), you are able to transfer the skills and know-how you've acquired on lower levels (this works well with e.g. operations skills but not as well with e.g. street protest skills). Also, though the multiplication of traits in this formula implies that the impact of some EAs is orders of a magnitude higher than others, it's hard to evaluate these traits as an outsider.
Specialise & transact
Does becoming good at all construal levels mean we should all become generalists? No, I actually think that as a growing community we’re doing a poor job at dividing up labour compared to what I see as the gold standard – decentralised market exchange. This is where we can use V2ADC to zoom out over the entire community:
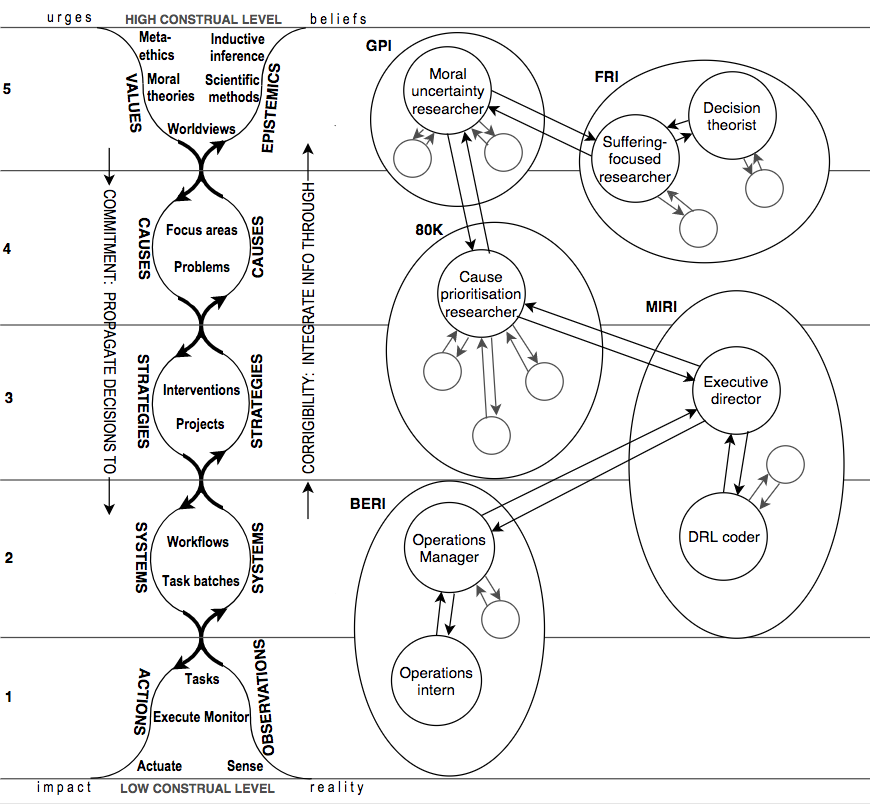
The circles illustrate network clusters of people that exchange a lot with each other. Interestingly, a cluster of agents working toward (shared) goals can be seen as a super-agent with resulting super-goals, as can one person with sub-goals be seen as being the amalgamation of sub-agents interacting with each other.
A more realistic but messy depiction of our community's interactions would look like this (+ line weights to denote how much people exchange). Also, people tend to actually cluster at each construal level – e.g. there are project team clusters, corporate animal welfare outreach clusters, factory farming reduction clusters, animal welfare clusters, and so on. Many of these clusters contain people who are not (yet) committed to EA, which makes sense, both in our ability to do good together and for introducing new people to the principles of EA.
See here for a great academic intro to social networks.
As more individuals become connected within the EA network, each should specialise at construal levels in a role they excel at. They can then transact with others to acquire other needed information and delegate remaining work (e.g. an operations staff member can both learn from a philosopher and take over organisational tasks that are too much for them). Exceptions: leaders and professional networkers often need to have a broad focus across all of these levels as they function as hubs – judiciously relaying information and work requests from one network cluster to another.
With a transaction, I mean a method of giving resources to someone (financial, human, social, and temporal capital) in return for progress on your goals (i.e. to get something back that satisfies your preferences).
Here are three transactions common to EAs:
- Collaborations involve giving resources to someone whom you trust, has the same goals and is capable of using your resources to make progress on those goals. When this is the case, EAs will tend to transact more and cluster in groups to capture the added value (the higher alignment in collaborations reduces principal agent problems).
- Reciprocal favours involves giving up a tiny amount of your resources to help someone make disproportional progress on goals that are unaligned with yours (e.g. connect someone working on another problem with a colleague), with an implicit expectation of that person returning the favour at some point in the future (put another way, it increases your social capital). It's a practical alternative to moral trade (instead of e.g. signing contracts, which is both time consuming and socially awkward). The downside of reciprocal favours is that you often won't be able to offer a specific resource that the other party wants. This is where a medium of exchange comes in useful instead:
- Payments involve giving someone money based on the recipient's stated intent of what they will do with that money.
EAs are never perfectly (mis)aligned – they will be more aligned at some levels and less aligned at others. For example:
- You can often collaborate with other EAs on shared goals if you pick the levels right. For one, most EAs strongly value internal consistency and rigour, so it's easy to start a conversation as a high-level collaboration to get closer to 'the truth'. To illustrate, though Dickens and Tomasik clearly disagreed here on moral realism vs. anti-realism, they still collaborated on understanding the problem of AI alignment better.
- Where goals diverge, however, reciprocal favours can create shared value. This can happen when e.g. a foreign aid policy maker types out a quick email for an animal charity director to connect her with a colleague (though if the policy maker made the decision because he's uncertain whether global poverty is the best focus area, it's a collaboration). But in a fundamental way, we're all unaligned: EAs, like other humans, have an intrinsic drive to show off their altruistic deeds through signalling. The recipients of these signals have the choice whether or not to encourage them to make these decisions again by giving back compliments, gifts and other tokens of social status. This in turn influences the community's norms at large.
- Although employers who pay money to cover the salaries tend to roughly agree with employees on e.g. what problems and interventions to work on, the employees also have sub-goals (such as feeling safe, comfortable and admired by others) that are personal to them.
By 'transacting' with others you're able to compensate for your personal limitations to achieving your goals: the fact that you'll never be able to acquire all required knowledge yourself nor be able to do all work as skillfully as the few things you can become particularly capable at.
Integrate the low-level into the high-level
Most of this post has been about pushing values down into actions, which implies that people doing low-construal level work should merely follow instructions from above. Although it's indeed useful for those people to use prioritisation advice to decide where to do work, they also fulfill the essential function of feeding back information that can be used to update overarching models.
We face a major risk of ideological rust in our community. This is where people who are working out high-level decisions either don't receive enough information from below or no longer respond to it. As a result, their models separate from reality and their prioritisation advice becomes misguided. To illustrate this…
At a Strategies level, you find that much of AI alignment research is built on paradigms like 'the intelligence explosion' and 'utility functions' that arose from pioneering work done by the Future of Humanity Institute and Machine Intelligence Research Institute. Fortunately, leaders within the community are aware of the information cascades this can lead to, but the question remains whether they're integrating insights on machine learning progress fast enough into their organisations' strategies.
At a Causes level, a significant proportion of the EA community champions work on AI safety. But then there's the question: how many others are doing specialised research on the risks of pandemics, nanotechnology, and so on? And how much of this gets integrated into new cause rankings?
At a Values level, it is crazy how one person's worldview leads them to work on safeguarding the existence of future generations, another on preventing their suffering and another to work on neither. This reflects actual moral uncertainty – to build up a worldview, you basically need to integrate most of your life experiences into a workable model. Having philosophers and researchers explore diverse worldviews and exchange arguments is essential in ensuring that we don't rust in our current conjecture.
Now extend it to organisational structure:
We should also use the principle of decentralised experimentation, exchange and integration of information more in how we structure EA organisations. There has been a tendency to concentrate resources (financial, human and social capital) within a few organisations like the Open Philanthropy Project and the Centre for Effective Altruism who then set the agenda for the rest of the community (i.e. push decisions down their chains).
This seems somewhat misguided. Larger organisations do have less redundancy and can divide up tasks better internally. But a team of 24 staff members is still at a clear cognitive disadvantage at gathering and processing low-level data compared to a decentralised exchange between 1000 committed EAs. By themselves, they can't zoom in closely on enough details to update their high-level decision models appropriately. In other words, concentrated decision-making leads to fragile decision-making – just as it has done for central planning.
Granted, it is hard to find people you can trust to delegate work to. OpenPhil and CEA are making headway in allocating funding to specialised experts (e.g. OpenPhil's allocation to CEA, which in turn allocated to EA Grants) and collaborating with organisations who gather and analyse more detailed data (e.g. CEA's individual outreach team working with the Local Effective Altruism Network). My worry is that they're not delegating enough.
Given the uncertainty they are facing, most of OpenPhil's charity recommendations and CEA's community-building policies should be overturned or radically altered in the next few decades. That is, if they actually discover their mistakes.
This means it's crucial for them to encourage more people to do local, contained experiments and then integrate their results into more accurate models.
EDIT: see these comments on where they could create better systems to facilitate this:
Private donors who have the time and aptitude to research specialised problem niches and feed up their findings to bigger funders should do so (likewise, CEA should actively discourage these specific donors from donating to EA Funds). Local community builders should test out different event formats and feed up outcomes to LEAN. And so on.
However, if bigger organisations would hardly use this information, most of the exploration value would get lost. Understandably, this outpour of data is too overwhelming for any human (tribe) to manually process.
We therefore need to build vertically integrated data analysis platforms that separate the signal from the noise, update higher-level models, and then share those models with relevant people. On these platforms, people can then upload data, use rigorous data mining techniques and share the results through targeted channels like the PriorityWiki.
I introduced this decision chain in a previous post, which I revised after chatting with thoughtful people at two CEA retreats. Thanks to Kit Harris for his incisive feedback on the categorisation, Siebe Rozendal and Fokel Ellen for their insightful text corrections, and Max Dalton, Victor Sint Nicolaas and all the other people who beared with me last year when I was still forming these ideas.
If you found this analysis valuable, considering making a donation or email me at remmelt@effectiefaltruisme.nl (also if you want to avoid transaction costs through a bank transfer). My student loan payments are stopping in August so you're close to funding my work at peak marginal returns (note: I have also recently applied to EA Grants to extend my financial runway).
Also, if you're at EAGx Netherlands, feel free to grill me at my workshop. :-)
Cross-posted on LessWrong.
I think you're making some valuable points here (e.g. making sure information is properly implemented into the 'higher levels') but I think your posts would have been a lot better if had skipped all the complicated modelling and difficult language. It strikes me as superfluous and the main result seems to me that it makes your post harder to read without adding any content.
Hi Denise, can you give some examples of superfluous language? I tried to explain it as simply as possible (though sometimes jargon and links are needed to avoid having to explain concepts in long paragraphs) but I’m sure I still made it too complicated in places.
It is still not clear to me how your model is different to what EAs usually call different levels of meta. What is it adding? Using words like 'construal level' complicates the matter further.
I'm happy to elaborate more via PM if you like.
Hmm, so here are my thoughts on this:
1) I think you’re right that the idea of going meta from the object level is an idea that’s known to many EAs. I’d argue though that the categorisations in the diagram are valuable though because I don’t know of any previous article where they’ve all been put together. For veteran EAs, they’ll probably be obvious but I still think it’s useful for them to make the implicit explicit.
2) The idea of construal levels is useful here because of how thinking in far vs. near mode affects psychology. E.g. when people think in far mode they
have to ignore details, and tend to be less aware that those nuances actually exist
tend to associate other far-mode things with whatever they think of. E.g. Robin Hanson’s point that many sci-fi/futurism books (except, of course, Age of Em) focus on values and broad populations of beings that all look similar, and have blue book covers (i.e. sky, far away)
So this is why I think referring to construal levels adds value. Come to think of it, I should have mentioned this in the post somewhere. Also my understanding of construal level theory is shoddy so would love to hear opinions of someone who’s read more into it.
BTW, my sister mentioned that I could have made the post a lot more understandable for her if I just started with ‘Some considerations like X are more concrete and other considerations like Y are more abstract. Here are some considerations in between those.’ Judging by, that I could have definitely written it more clearly.
I've added some interesting links to the post on near vs. far mode thinking, which I found on LessWrong and Overcoming Bias.
Or add a tl;dr
Hmm, I personally value say five people deeply understanding the model to be able to explore and criticise it over say a hundred people skimming through a tl;dr. This is why I didn’t write one (besides it being hard to summarise anything more than ‘construal levels matter – you should consider them in the interactions you have with others’, which I basically do in the first two paragraphs). I might be wrong of course because you’re the second person who suggested this.
This post might seem deceptively obvious. However, I put a lot of thinking into both refining categories and the connections between them and explaining them in a way that hopefully enables someone to master them intuitively if they take the time to actively engage with the text and diagrams. I probably did make a mistake by outlining both the model and its implications in the same post because it makes it unclear what it’s about and causes discussions here in the comment section to be more diffuse (Owen Cotton-Barratt mentioned this to me).
If someone prefers to not read the entire post, that’s fine. :-)
For me tl;dr is mainly useful for two things:
Excellent work. I hope you'll forgive me taking issue with a smaller point:
I'm not so sure that this is true, although it depends on how big an area you imagine will / should be 'overturned'. This also somewhat ties into the discussion about how likely we should expect to be missing a 'cause X'.
If cause X is another entire cause area, I'd be pretty surprised to see a new one in (say) 10 years which is similar to animals or global health, and even more surprised to see one that supplants long term future. My rationale for this is I see broad funnel where EAs tend to move into the long term future/x-risk/AI, and once there they tend not to leave (I can think of a fair number of people who made the move from (e.g.) global health --> far future, but I'm not aware of anyone who moved from far future --> anything else). There are also people who have been toiling in the long term future vinyard for a long time (e.g. MIRI), and the fact we do not see many people moving elsewhere suggests this is pretty stable attractor.
There are other reasons for a cause area being a stable attractor besides all reasonable roads lead to it. That said, I'd suggest one can point to general principles which would somewhat favour this (e.g. the scope of the long term future, that the light cone commons, stewarded well, permits mature moral action in the universe to whatever in fact has most value, etc.) I'd say similar points to a lesser degree to apply to the broad landscape of 'on reflection moral commitments', and so the existing cause areas mostly exhaust this moral landscape.
Naturally, I wouldn't want to bet the farm on what might prove overconfidence, but insofar as it goes it supplies less impetus for lots of exploratory work of this type. At a finer level of granulariy (and so a bit further down your diagram), I see less resilience (e.g. maybe we should tilt the existing global poverty portfolio more one way or the other depending how the cash transfer literature turns out, maybe we should add more 'avoid great power conflict' to the long term future cause area, etc.) Yet I still struggle to see this adding up to radical alteration.
First off, I was ambiguous in that paragraph about the level I actually thought decisions should be revised or radically altered. i.e. in say the next 20 years, did I think OpenPhil should revise most of the charities they fund, most of the specific problems they funded or broad focus areas? I think I ended up just expressing a vague sense of ‘they should change their decisions a lot if they put in much more of the community’s brainpower into analysing data from a granular level upwards’.
So I appreciate that you actually gave specific reasons for why you'd be surprised to see a new focus area being taken up by people in the EA community in the next 10 years! Your arguments make sense to me and I’m just going to take up your opinion here.
Interestingly, your interpretation that this is evidence for that there shouldn't be a radical alteration in what causes we focus can be seen both as an outside view and inside view. It's an outside view in the sense that it weights the views of people who've decided to move into the direction of working on the long term future. It's also an inside view in that it doesn't consider roughly what percentage of past cosmopolitan movements where members converged on working on a particular set of problems were seen as wrong by their successors decades later (and perhaps judged to have been blinded by some of the social dynamics you mentioned: groupthink, information cascades and selection effects).
A historical example where this went wrong is how in the 1920's Bertrand Russell and other contemporary intelligentia had positive views on communism and eugenics, which later failed in practice under Stalin's authoritarian regime and Nazi Germany, respectively. Although I haven't done a survey of other historical movements (has anyone compiled such a list?), I think I still feel slightly more confident than you that we'll radically alter what we'll work on after 20 years if we'd make a concerted effort now to structure the community around enabling a significant portion of our 'members' (say 30%) to work together to gather, analyse and integrate data at each level (whatever that means).
It does seems that we share some intuitions (e.g. the arguments for valuing future generations similarly to current generations seem solid to me). I've made a quick list on research that could lead to fundamental changes in what we prioritise at various levels. I'd be curious to hear if any of these points has caused you to update any of your other intuitions:
Worldviews
more neuroscience and qualia research, possibly causing fundamental shifts in our views on how we feel and register experiences
research into how different humans trade off suffering and eudaimonia differently
a much more nuanced understanding of what psychological needs and cognitive processes lead to moral judgements (e.g. the effect on psychological distance on deontologist vs. consequentialist judgements and scope sensitivity)
Focus areas:
Global poverty
use of better metrics for wellbeing – e.g. life satisfaction scores and future use of real-time tracking of experiential well-being – that would result in certain interventions (e.g. in mental health) being ranked higher than others (e.g. malaria)
use of better approaches to estimate environmental interactions and indirect effects, like complexity science tools, which could result in more work being done on changing larger systems through leverage points
Existential risk
more research on how to avoid evolutionary/game theoretical “Moloch” dynamics instead of the current "Maxipok" focus on ensuring that future generations will live and hope that they have more information to assess and deal with problems from there
for AI safety specifically, I could see a shift in focus from a single agent produced out of say a lab that presumably gets so powerful to outflank all other agents to analysing systems of more similarly capable agents owned by wealthy individuals and coalitions that interact with each other (e.g. like Robin Hanson's work on Ems) or perhaps more research on how a single agent could be made out of specialised sub-agents representing the interests of various beings. I could also see a shift in focus to assessing and ensuring the welfare of sentient algorithms themselves.
Animal welfare
more research on assessing sentience, including that of certain insects, plants and colonial ciliates that do more complex information processing, leading to changed views on what species to target
shift to working on wild animal welfare and ecosystem design, with more focus on marine ecosystems
Community building
Some concepts like high-fidelity spreading of ideas and strongly valuing honesty and considerateness seem robust
However, you could see changes like emphasising the integration of local data, the use of (shared) decision-making algorithms and a shift away from local events and coffee chats to interactions on online (virtual) platforms
I agree history generally augurs poorly for those who claim to know (and shape) the future. Although there are contrasting positive examples one can give (e.g. the moral judgements of the early Utilitarians were often ahead of their time re. the moral status of women, sexual minorities, and animals), I'm not aware of a good macrohistorical dataset that could answer this question - reality in any case may prove underpowered.
Yet whether or not in fact things would change with more democratised decision-making/intelligence gathering/ etc., it remains an open question whether this would be a better approach. Intellectual progress in many areas is no longer an amateur sport (see academia, cf. ongoing professionalisation of many 'bits' of EA, see generally that many important intellectual breakthroughs have historically been made by lone figures or small groups versus more swarm-intelligence-esque methods), and there's a 'clownside' risk of lot of enthusiastic, well-meaning, but inexperienced people making attempts that add epistemic heat rather than light (inter alia). The bar to appreciate 'X is an important issue' may be much lower than 'can contribute usefully to X'.
A lot seems to turn on whether the relevant problems are more high serial depth (favouring intensive effort) high threshold (favouring potentially-rare ability) or broader and relatively shallower, favouring parallelization. I'd guess relevant 'EA open problems' are a mix, but this makes me hesitant for there to be a general shove in this direction.
I have mixed impressions about the items you give below (which I appreciate was meant more as quick illustration than some 'research agenda for the most important open problems in EA'). Some I hold resilient confidence the underlying claim is false, for more I am uncertain yet I suspect progress on answering these questions (/feel we could punt on these for our descendants to figure out in the long reflection). In essence, my forecast is that this work would expectedly tilt the portfolios, but not so much to be (what I would call) a 'cause X' (e.g. I can imagine getting evidence which suggests we should push more of a global health portfolio to mental health - or non-communicable disease - but not something as decisive where we think we should sink the entire portfolio there and withdraw from AMF/SCI/etc.)
I appreciate you mentioning this! It’s probably not a minor point because if taken seriously, it should make me a lot less worried about people in the community getting stuck in ideologies.
I admit I haven’t thought this through systematically. Let me mull over your arguments and come back to you here.
BTW, could you perhaps explain what you meant with the “There are other causes of an area...” sentence? I’m having trouble understanding that bit.
And with ‘on-reflection moral commitments’ do you mean considerations like population ethics and trade-offs between eudaimonia and suffering?
Sorry for being unclear. I've changed the sentence to (hopefully) make it clearer. The idea was there could be other explanations for why people tend to gravitate to future stuff (group think, information cascades, selection effects) besides the balance of reason weighs on its side.
I do mean considerations like population ethics etc. for the second thing. :)
I like the model a lot, thanks for posting!
One input: I think it could be useful to find a term for it that's easier to memorize (and has a shorter abbreviation).
Hmm, I can’t think of a clear alternative to ‘V2ADC’ yet. Perhaps ‘decision chain’?
Yeah, that sounds great. Decision chain (abbreviated "DC").
Changed it in the third paragraph. :-)
Good stuff! You might be interested in both OODA loops and Marr's levels of analysis.
Thanks for the pointers!
Would you see OODA loops translated to V2ADC as cycling up and down (parts of) the chain as quickly as possible?
I found this article on Marr’s levels of analysis: http://blog.shakirm.com/2013/04/marrs-levels-of-analysis/ Seems like a useful way of guiding the creation of algorithms (never heard of it before – I don’t know much about of coding or AI frameworks).
I appreciate this model and feel like it has the potential to be foundational in a rigorous account of group rationality.
This especially because I find that the higher levels lack a proper feedback mechanism. A common pattern seems to be that we often discuss high-level moral philosophy without making any hard decisions on which philosophy to employ. I even use “so have we fixed morality yet?” As a joke. People laugh, not because I pretend that it’s easy, but because I pretend that making a decision is the point. I suspect that this lack of pressure leads to impoverished thinking.
Imagine a world where an institution strived to deliver solutions to moral problems as an input to another institution that further carried it out. I’d expect the sense of responsibility to lead to much better thinking. No more belief as attire.
But then maybe this is already happening in places I haven’t been. Still, I’d love to see more people take responsibility for providing workable answers to philosophy. Even when they’re just chatting at social events.
Fantastic post! It's a significant upgrade from the "terminal/instrumental values" mental model I was previously using.
When I first joined EA, I looked at the annual survey of EAs and was surprised to see so much variation in how EAs ranked the importance of the major causes. I thought that the group would be moving towards a consensus, and that each individual member would be able to trace their actions up towards their understanding of the most important causes.
Personally, I tried to build up my own understanding of the cause priority from strong foundations, doing my best to answer meta questions like "do I value all people equally", "how do I weight animal suffering vs human happiness". From there, I worked my way down the V2ADC, trying to meta-analyze the research on causes, eventually coming to an area that I felt confident was the best place to add value.
I think with a bit more nuance, the EA survey could serve as a good feedback mechanism to see where on the chain we all see ourselves, and to see if the sum of the parts adds up to anything resembling a consistent whole. Will the EA community end up converging in beliefs and strategy? Is it an elephant in the room to say that half of the people working on X cause aught to shift to Y cause because the people up the chain are confident that it is a better move for the community? Even if the exploratory folks at the bottom raised their evidence up the chain, would we have enough corrigibility to pivot? (Love that word, totally gonna use it more!)
Hi @Naryan,
I’m glad that this is a more powerful tool for you.
And kudos for working things from the foundations up! Personally, I still need to take a few hours with a pen and paper to systematically work myself through the decision chain myself. A friend has been nudging me to do that. :-)
Gregory Lewis makes the argument above that some EAs are moving in the direction of working on long term future work and few are moving back out. I’m inclined to agree with him that they probably have good reasons for that.
I’d also love to see the results of some far mode — near mode questions put in the EA Survey or perhaps send out by Spencer Greenberg (not sure if there’s an existing psychological scale to gauge how much people are in each mode when working throughout the day). And of course, how they corellate with cause area preferences.
Max Dalton explained to me how ‘corrigiblity’ was one of the most important traits to look for for selecting people you want to work with at EA Global London last year so credit to him. :-) My contribution here is adding the distinction that people often seem more corrigible at some levels than others, especially when they’re new to the community.
(also, I love that sentence – “if the exploratory folks at the bottom raised evidence up the chain...”)
I really liked this post and the model you've introduced!
With regards to your pseudomaths, a minor suggestion could be that your product notation is equal to how agentive our actor is. This could allow us to take into account impact that is negative (i.e., harmful processes) by then multiplying the product notation by another factor that takes into account the sign of the action. Then the change in impact could be proportional to the product of these two terms.
I'm happy to hear that it's useful for you. :-)
Could you clarify what you mean with agentive? The way I see it, at any of the levels from 'Values' to 'Actions', a person's position on the corrigibility scale could be so low to be negative. But it's not an elegant or satisfactory way of modelling it (i.e. different ways of adjusting poorly to evidence could still lead to divergent results from an extremely negative Unilateralist's Curse scenario to just sheer mediocrity)
By agentive I sort of meant "how effectively an agent is able to execute actions in accordance with their goals and values" - which seems to be independent of their values/how aligned they are with doing the most good.
I think this is a different scenario to the agent causing harm due to negative corrigibility (though I agree with your point about how this could be taken into account with your model).
It seems possible however that you could incorporate their values/alignment into corrigibility depending on one's meta-ethical stance.
Ah, in this model, I see ‘effectiveness in executing actions according to values’ a result of lots of directed iteration of improving understanding at lower construal levels over time (reminds of the OODA loop that Romeo mentions above, will also look into the ‘levels of analysis’ now ). In my view, that doesn’t require an extra factor.
Which meta-ethical stance do you think this wouldn’t fit into the model? I’m curious to hear your thoughts to see where it fails to work.
Ah okay - I think I understand you, but this is entering areas where I become more confused and have little knowledge.
I'm also a bit lost as to what I meant by my latter point, so will think about it some more if possible.
Do you know of a tax-deductible way to support you as a UK donor?
Maybe via EA Grants?
EA UK (the organisation behind EA London) could potentially help for large enough donations, but I'd need to check and it would take a bit of time to administer. I was just wondering if there was an existing mechanism.
@Peter, any idea how EA Grants could be used as an intermediary here? (I did apply myself to EA Grants but I’m not expecting to cover the financial runway of myself or EAN for any longer than 6 months with that)
I was just speculating that maybe EA Grants could help match up individual donors with projects by having the donor make a tax deductible donation to CEA and CEA passing it along as an EA Grant. I don't know if this actually could work and I now see several reasons why maybe it wouldn't.
Good question... I haven’t really thought about it but if it’s a £20,000+ donation perhaps EA Netherlands could register at the HMRC? https://www.givingwhatwecan.org/post/2014/06/tax-efficient-giving-guide-uk-donors/