This is a cross-post from my blog Maximum Progress.
More lives were saved by vaccines than by any other response to Covid-19. Vaccines save more lives if they are available earlier. I use two methods to estimate how many lives might have been saved given an earlier vaccine rollout. I then consider safety and efficacy information tradeoffs and non-regulatory constraints to vaccine rollout. I find that a 4 month acceleration to a vaccine rollout in August of 2020 was achievable and would have saved between 130 and 350 thousand lives over the next two years of the pandemic. I conclude with recommendations for future pandemics given the large benefits of vaccine acceleration.
I. Introduction
Of all measures taken to curb the spread or danger of the Covid-19 pandemic, vaccines were by far the most effective. With three doses, a vaccinated person is 41 times less likely to die from Covid than an unvaccinated person. Incredibly, these vaccines were designed essentially over a weekend at the earliest stage of the pandemic in January of 2020. It took 11 months, billions of dollars, and thousands of man hours for these life-saving vaccines to get through the FDA’s emergency testing process. This was the fastest vaccine rollout ever, and it was the result of immense effort and ingenuity. Still, over three hundred thousand people died in those 11 months. The lives we could have saved with a faster vaccine rollout are in an invisible graveyard since we cannot observe the counterfactual worlds where they still live. In sections II and III, I use two methods: linear estimation and SIR modeling, to perform counterfactual analyses of earlier vaccine approvals using CDC data and find that hundreds of thousands of lives could have been saved even with modest accelerations. Then, in section IV I analyze the costs and feasibility of an accelerated rollout. Section V concludes with recommendations for future pandemics.
II. Linear Estimation
In this section I approach the question of how many lives could have been saved by an earlier vaccine rollout by simply scaling down observed cases by a constant “vaccine effect” term which is calculated from CDC data on differences in death rates depending on vaccination status.
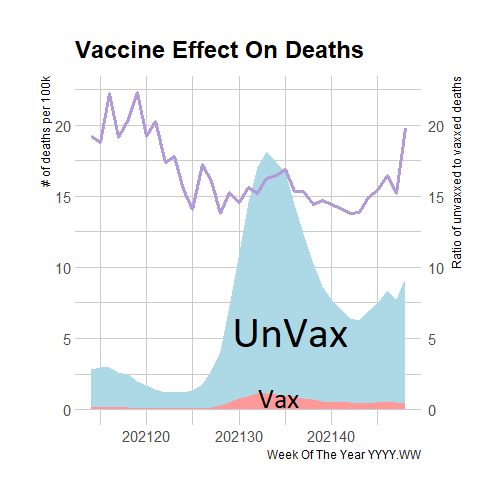
Figure 1. Deaths Rates Among Unvaccinated (blue) and Vaccinated (red)
Source: CDC Data on differential death rates found on their website here.
This data shows that unvaccinated people are at somewhere between a 15 and 20 times higher risk of death from Covid than vaccinated people. Some of this effect is probably due to the selection of more cautious people into the vaccinated category who would have been less likely to die anyway. But there is also a selection of the most vulnerable into the vaccinated category, especially older people, which would make the vaccinated group as a whole look more likely to die. Direct studies of the vaccine’s protection confirm large effects so I take the mean of this vaccine effect (16.6) as the constant vaccine effect for the rest of this section. The CDC’s data on the vaccine effect only goes back to April of 2021, but they have data on Covid-19 cases and deaths since the start of the pandemic. This data is what will be scaled up or down by the vaccine effect.
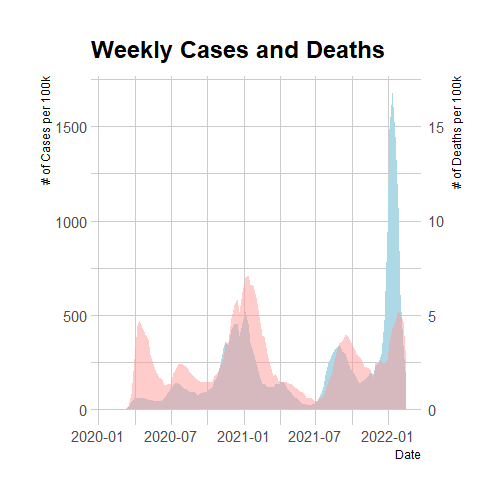
Figure 2. New Cases (blue) and Deaths (red) Per 100k Each Week Since January 2020
Source: CDC Data found on their website here.
In the counterfactual estimations of earlier vaccine rollouts, the vaccine scaling effect will only apply to the percentage of the population who are vaccinated, over and above the percentage who were already vaccinated at that point in the actual rollout schedule, graphed below.
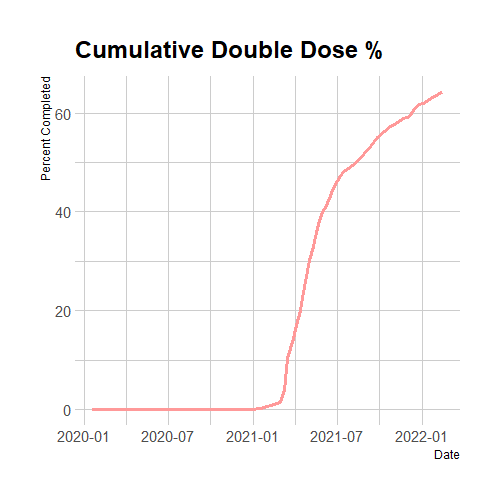
Figure 3. Cumulative Double Dose Percentage
Source: CDC Data on vaccination rates found on their website here.
The estimation of counterfactuals essentially works by shifting the above curve backwards in time. Formally, let Dₜ = Observed Covid deaths per 100k on week t, let VaxPctₜ = The percentage of the population with at least two vaccine doses on week t, let VE = 16.6, the vaccine effect, and let i = The number of weeks you wish to shift the rollout schedule back by. Then equation (1) describes the estimated counterfactual deaths for any week.
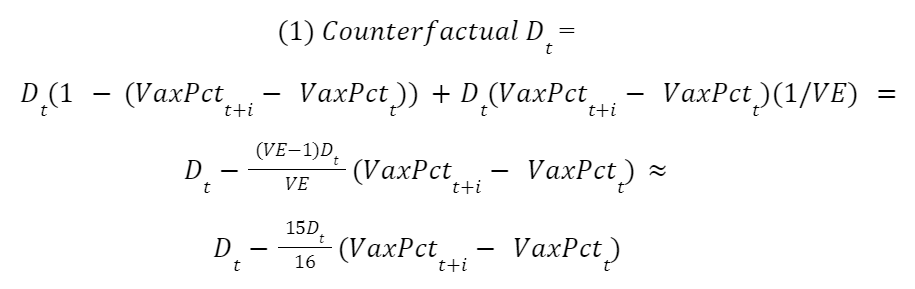
You can interpret this equation as follows: Since the vaccine effect for Covid is ~16, we know that a world where everyone is vaccinated has 1/16th the number of deaths compared to a world with no vaccinations. This is reflected in the equation. When VaxPctt+i - VaxPct = 1, counterfactual Dt = Dt - 15/16(Dt). We never reach 100% vaccination even in the most optimistic counterfactuals so we only get a portion of this decrease in practice.
I estimate 12 counterfactual scenarios by shifting the approval date back 4 weeks at a time from the actual approval date on December 14th 2020, to January 20th 2020, a week after Moderna’s vaccine was first designed. Since these two dates are not an exact multiple of 4 weeks apart, I estimate two counterfactuals for approval in November of 2020 which are only 21 days apart. Additionally, I assume that in the future the double dose vaccination rate will rise linearly over 48 weeks until 70% of the population is double-dose vaccinated. This fills in the future vaccination data that the accelerated counterfactuals would already be at now. Rising linearly is somewhat unrealistic, but double dose vaccination percentage rising 5 percentage points over 48 weeks is conservative compared to the observed growth rates. Below, I print 2 examples of counterfactual death rates compared to the observed ones and a graph which shows the cumulative lives saved for each of the 12 early approval scenarios.
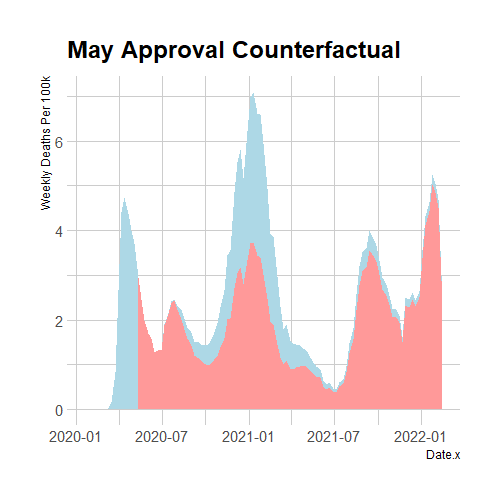
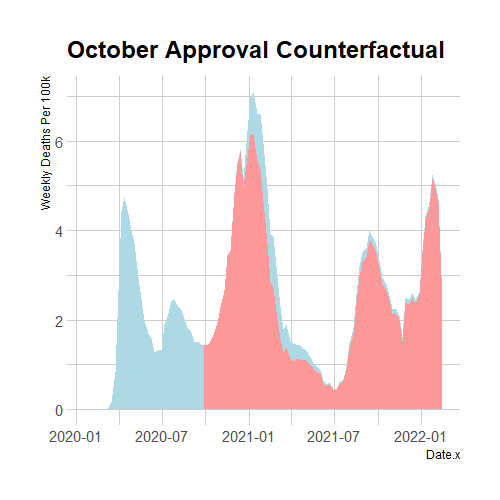
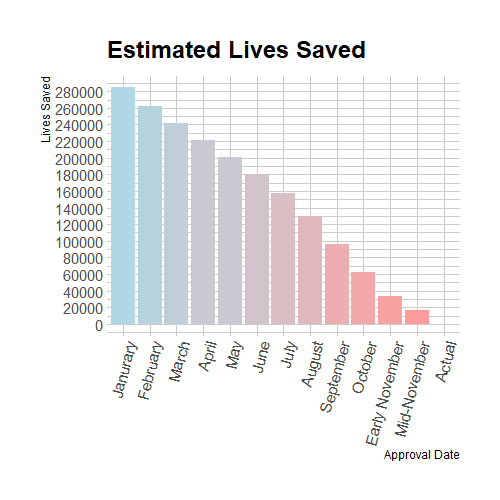
Figure 4. Extra Lives Saved At Different Approval Dates
Linearly scaling down death rates without changing anything else is clearly an inaccurate model of how pandemics spread. This estimate assumes that vaccines simply change the levels of infection and death, but leave the growth rates unchanged. In actual fact, the growth rates of infection and death are proportional to the levels. This proportionality means that decreasing the absolute number of infected people leads to slower growth rates which lead to lower future levels which lead to slower growth rates etc. So although our model is inaccurate, it can serve as an informative lower bound because a better model which takes this relationship between levels and derivatives into account would find larger impacts of early vaccine approval as the decreased population of potential spreaders have effects which reverberate throughout all future growth. Even in this lower bound estimate, it is clear that over ten thousand lives could have been saved even if the FDA had just scheduled meetings sooner and worked through the 2020 holidays. The next section will use an SIR model to more accurately estimate the effects of earlier vaccine approvals.
III. SIR Model Estimation
My second pass at estimating the number of people whose lives could have been saved by various accelerated vaccine rollouts will be through replicating Andrew Atkeson’s behavioral SIR model.
Atkeson’s model improves the accuracy of this estimate in two ways. First, it incorporates the self-referential nature of pandemic growth rates. The number of people transiting from Susceptible to Infectious depends on the number of currently infected, and similarly for Infectious to Recovered (or dead). Atkeson also adds parameters representing people’s behavioral responses to the pandemic. This is important because people’s behavioral changes have had huge effects on the transmission of Covid-19. Behavioral adaptations like working from home, avoiding restaurants, and wearing masks turned what a pure SIR model would have predicted to be an extreme but short pandemic into a longer but more moderated one. In Atkeson’s model, these behavioral responses are proportional to the current death rate, and since they influence the future death rate, it is another self-referential layer. In addition to the behavioral part of Atkeson’s paper, he modified the traditional SIR model to incorporate different infection, reinfection, and fatality rates among variants and seasonal effects on transmission. If you are interested, I explain how SIR models work in general and go in depth on how Atkeson’s model works in this public Google doc. Otherwise, I continue with the punchline: Atkeson’s SIR model closely matches observed deaths data.
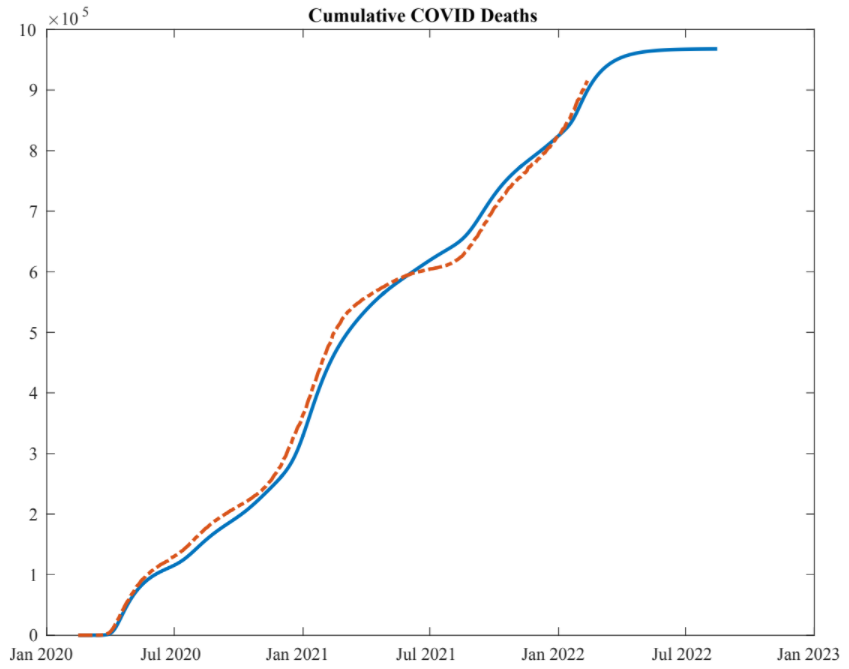
Figure 5. Atkeson’s Model Predictions (Blue) And Observed Data (Orange) For Deaths
In the baseline model shown above, Atkeson assumes that vaccines start transferring people from Susceptible to Recovered on January 1st 2021, a little over two weeks after the actual approval date of December 11th for Pfizer and 18th for Moderna. I shift this start date back 28 days at a time and estimate 12 different accelerated rollout times. Atkeson’s case and death data only goes back to February of 2020 so I am not able to use his model to estimate the cumulative lives saved from the same January 2020 date that I used in my linear estimation. This is a slight inconsistency but it is not important since such an accelerated rollout is unrealistic and probably not all that much more effective since the marginal impact of acceleration is decreasing the further back it is pushed.
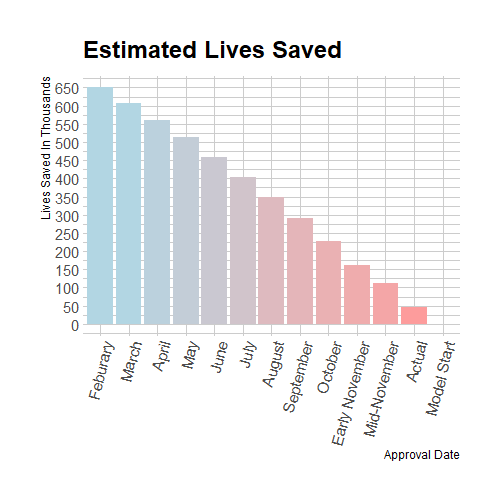
Figure 6. Extra Lives Saved At Different Approval Dates In Atkeson’s Model
This relationship between accelerated approval dates and lives saved is less linear than my initial estimate, with the largest marginal effects in the first few weeks of acceleration. As expected, the estimates of the number of lives saved is significantly higher with this model than in my linear estimation. The table below compares the results from each estimation. These estimates are both everywhere below the 981 thousand observed deaths which is a minimum condition for reasonability.
Table 2—Lives Saved By Accelerated Vaccines In Each Estimation
Accelerated Approval Date | Linear Estimation | SIR Model |
Lives Saved In Thousands: | Lives Saved In Thousands: | |
Mid-November | 17 | 112 |
Early November | 34 | 163 |
October | 63 | 228 |
September August July June May April March February January | 97 130 157 180 201 221 242 262 285 | 291 350 405 460 513 561 607 650 N/A |
Hundreds of thousands of people lay dead in the invisible graveyard. Any avoidable delays of the vaccine rollout were deadly errors of omission.
The next section will consider the tradeoffs with safety and manufacturing feasibility that come with accelerating the regulatory approval of vaccines.
IV. Feasibility Of An Accelerated Rollout
Both estimates of the number of lives saved by accelerated rollouts are monotonically increasing as the vaccine approval date gets pushed back. But these predictions should be mediated by the tradeoff between an earlier approval date and information about the safety and efficaciousness of the vaccine and physical constraints on vaccine manufacturing speed.
i) Safety and Efficaciousness Tradeoffs
In hindsight we can see that if the FDA had approved the Moderna and Pfizer vaccines for production as soon as they were synthesized, we would have dealt with the Covid-19 pandemic much faster. However, in that present moment the potential positive impact of the vaccines were diluted by the risk of negative vaccine effects. Safety risks for vaccines are not large compared to the risks of Covid, and trials confirmed the safety of all the vaccine candidates within weeks of their creation. The real challenge was determining efficacy to avoid investing production resources into useless vaccines and to avoid behavioral responses which would damage vaccine uptake. Human challenge trials would plausibly increase the information we get on safety and efficaciousness and significantly speed up the trial process.
Even after Phase 1 trials cut out the left tail of possible harmful effects of the vaccines, it was not at all clear which, if any, of the half-dozen vaccines in trials would be the most effective. This information was necessary before moving forward with production and distribution. Trying to manufacture large batches of all of the candidates would guarantee wasting scarce inputs and complementary goods on useless vaccines. Even worse, distributing all of the candidate vaccines as soon as possible, while life-saving for those who receive the Pfizer and Moderna vaccines, would be at best useless for those who receive inefficacious doses. Most likely, it would mean increased risk of exposure due to a false perception of protection from their vaccine and a greater unwillingness to receive the actually effective doses once the data is collected. Acting without information on the relative effectiveness of the vaccine candidates was not a feasible strategy for mitigating the pandemic. So then was the FDA justified in taking 11 months to gather this information before giving approval? Only if there was no other way to get the required information any faster. But there was: human challenge trials.
Human challenge trials are best understood in contrast to traditional clinical trials. For the Covid-19 vaccines, traditional trials entailed enrolling tens of thousands of volunteers into their study, randomly vaccinating half of them, and then waiting around for enough of them to get infected and provide sufficient sample size to statistically discern the efficaciousness of their vaccine. With the relatively low infection rates in 2020, and the 2 week spaced dose regimen, this means waiting over 6 months for results.
Human challenge trials are much faster than clinical trials and provide at least as much information about effectiveness, if not more. Running a human challenge trial begins with enrolling a few hundred volunteers and randomly vaccinating half of them. In departure from clinical trials, instead of waiting around for enough participants to get infected, everyone in the trial is deliberately exposed to Covid-19. This way, researchers get a large enough sample size as soon the virus has enough time to show symptoms: within two weeks. Including the time to sign up and gather volunteers, the 2 week spaced doses, and Covid-19’s symptomatic window, these human challenge trials could easily be done within 2 months. This speed is a huge advantage over clinical trials, but it is not the only one. In a human challenge trial, all of the participants can be closely monitored over the entire study, giving researchers a better look into vaccine side effects and how Covid symptoms differ in those who are vaccinated but still get infected. This kind of monitoring is not possible for 30,000 participants over 6 months. Additionally, human challenge trials could get a much larger sample size of infections, even though the number of participants is an order of magnitude smaller. There were only 170 total infections tracked in Pfizer’s clinical trial. Even a small human challenge trial of say 500 could double this number and give us more information about the course of the disease and effectiveness of the vaccine.
Adherence to tradition, deontological commission bias, and democracy’s exclusive enforcement of Type I error led public health authorities to reject human challenge trials. Any reasonable moral calculus sees this as a tragedy. The stated reason for the rejection of human challenge trials was risk to the participants. It is of course true that volunteering to be deliberately injected with Covid-19 is volunteering for a small risk of death, but this risk is required for both HCTs and traditional trials. Traditional trials rely on hundreds of people to being infected with Covid, volunteers or not. Frontline healthcare workers also volunteer for increased risk of infection and we applaud because their sacrifice saves many more lives than it risks. Given that the public health authorities have already revealed their willingness to make these tradeoffs with human lives, the human challenge trial tradeoff is attractive. Say we run a human challenge trial with a thousand participants. Even if 10% of the thousand volunteers who receive a placebo die from their deliberate infection, a massive overestimate given Covid’s fatality rate of less than one percent, we would still save thousands of lives on net. In the weeks leading up to the FDA’s approval of the vaccines in December, more than two thousand people were dying from Covid every single day. Our estimates in section I and II predict that tens of thousands of lives could be saved if vaccine approval were accelerated by a few weeks. The four months of acceleration that human challenge trials offered would have saved hundreds of thousands of lives.
ii) Manufacturing Feasibility
If FDA approval speed were not the binding constraint on the vaccine rollout, how fast could manufacturing have begun? This counterfactual is difficult to answer precisely, but there are several observations which can lead to a reasonable range of estimates.
The first observation is that, around the observed approval date of December 11th, the elasticity of vaccine rollout timeline with respect to FDA approval date was basically 1:1. “Operation Warp Speed officials said that, within 24 hours of the Pfizer vaccine winning clearance, the government would begin releasing 6.4 million doses … once the Moderna vaccine is authorized … 12.5 million doses would begin to be released, under a similar schedule.” There was at least a few weeks where vaccine manufacturing and delivery capacity was ready and waiting in the wings while the FDA dithered on scheduling meetings and data review: "Pfizer submitted data detailing the safety and effectiveness of its vaccine on Nov. 22. But rather than immediately convening experts, the FDA scheduled a review meeting on Dec. 10, almost three weeks later.” Our lower bound estimate for the number of lives saved by a three week acceleration is seventeen thousand lives. Considering the non-linear effects of increased protection from vaccines, a three week acceleration might have saved over a hundred thousand lives over the past 16 months.
Beyond these first few weeks of acceleration, things become less clear, but there is good reason to expect that manufacturing would have spun-up faster in response to a faster approval process. The large manufacturers were making big strides very early on, long before they had certainty that their vaccine would be approved. Pfizer and BioNtech signed a letter of intent in March 2020 to work together on a vaccine, “Within those first 100 days, the first manufacturing run was a success. By late July, when the Pfizer-BioNTech team selected the vaccine candidate that appeared to be the most promising, the manufacturing teams were ready to ramp up production.” The AstraZeneca vaccine was also ready for manufacturing faster than approval “Four bioreactors, each with a 2,000-liter capacity, will allow the production of a billion doses of vaccine in two months,” which would place this billion dose capacity in September of 2020.
Another place to look is at the development of strain specific boosters later in the pandemic. Uğur Şahin, founder and CEO of BioNTech, says “Technically it is possible to make a new vaccine mimicking the new strain in a few weeks.” That’s just for the synthesis of the first hundred liters of test batches, but Moderna says “If the big trials can be skipped, that suggests the timeline to bring a new shot to market would be shortened by at least four months, if not more.”
Even if the actual vaccine could be manufactured to keep up with an accelerated human challenge trial schedule, there are dozens of complementary goods which are needed to store and distribute the vaccine. It is again unclear exactly how far back the timeline could be pushed before these complementary goods became a binding constraint, but there is good reason to believe that several months of acceleration was possible. Large stockpiles and production capacity for these goods were available to be substituted from other industries, and a quicker decision on vaccine effectiveness and approval would have allowed these manufacturers to produce these complementary goods months earlier without the extra cost of uncertainty.
The two most obvious complements to vaccine material are glass vials to store it in and needles to deliver it. Because of the huge existing capital stock for producing these goods, they would not have been bottlenecks to the vaccine timeline even in an accelerated human challenge trial timeline. We didn’t start preparing vials for vaccine delivery until may of 2020, but we were still able to get enough to produce billions of doses a few months later. The CEO of Schott, one of the world’s largest producers of boron-silicate glass said in early August “We can supply vials for 2 billion vaccine doses, when the time comes.” It wasn’t until two months later in late June that an American glass maker, Sio2, got the go ahead from BARDA to actually start expanding their production capacity “In June, after extensive presentations to Barda and the U.S. Department of Defense, SiO2 won a $143 million investment to accelerate its production—from 14 million vials to 120 million by December.” So billions of boro-silicate vials were available from already large producers and smaller ones were able to 10x their output in just 6 months. If BARDA had skipped the extensive presentations and invested early in 2020, there would have been plenty of vials available to store the vaccine after human challenge trials.
It is a similar story with needles. Tens of billions of medical needles are already produced every year. Single companies measure their output in billions, and they have giant needle manufacturing plants where the marginal cost of increasing production is relatively low. Becton-Dickinson, one of the US’s largest needle makers said in the summer of 2020 “We haven’t seen any issues in these supply chains, so we aren’t worried about that currently, If the Trump administration were to order 300 million hypodermic injection sets, Becton-Dickinson could do that.”
Adjuvants are another important input to vaccines. They are basically bio-chemical catalysts that help your immune system target the injected material and prepare the immune response as intended. Adjuvants are also used in several other vaccines and medicines, so pharmaceutical giants had billions of doses of capacity ready even before the pandemic began. For example, GlaxoSmithKline “announced it would manufacture 1 billion units of its AS03 adjuvant, which has worked with pandemic flu vaccines, to supply multiple developers of Covid vaccines around the world.”
iii) Conclusion
Even with wide confidence intervals around the timelines of a human challenge trial, manufacturing schedules, and the life-saving effects of accelerated vaccine distribution, it is clear that faster vaccine distribution was both possible and desirable. The most likely point estimate of distribution start date with a human challenge trial is a 4 month acceleration, placing the first shots in arms sometime in August of 2020. This would have saved at least a hundred thousand lives, and perhaps as many as three hundred thousand. Placing a 2 month confidence interval around August expands our possible lives saved estimate from 63 to 500 thousand lives saved.
V. Recommendations For Future Pandemics
These estimates of what we could have done are only useful insofar as they influence what we will do in the future. If we start preparing now for pandemics that have not infected anyone yet, we can build up an arsenal of vaccines and delivery mechanisms that could be deployed within three months of the first sighting of the infectious agent. If we had this preparation in Covid’s case we would have had vaccines ready before the first thousand cases on American soil. This preparation would require billions of dollars a year, but the economic cost of Covid has been in the trillions .
This preparation plan would work like a more targeted version of our own immune system. Within all of us, there are T-cells containing antibodies which would neutralize any virus. This includes Covid-19, the common cold, ebola, and billions more. Your T-cells prepare these antibodies before ever encountering these viruses by mixing and matching viral genetic code it has collected with its own DNA, producing billions of unique proteins. This strategy is comprehensive but slow. After actually encountering a virus in the body, dendritic cells have to search through billions of T-cells to find the one containing the right antibody. They often don’t find it in time to curtail the exponential growth of deadly viruses.
With a little ingenuity, we can replicate most of the benefits of this system by preparing vaccine responses to the 100 or so most common viral proteins. Georgetown virologist Angela Rasmussen says “We do this every year for influenza. We don’t know which influenza viruses are going to be circulating, so we make our best guess. And then we formulate that into a vaccine using essentially the same technology platform that all the other influenza vaccines are based on.” We can make a hundred best guesses for which viruses are most likely to become the world’s next pandemic. Even if the actual culprit has some differences from the mRNA sequences we had prepared, it would likely be in a similar class of viruses that we had prepared for, like how SARS, MERS, the cold, and Covid are all coronaviruses. So we would still have a vaccine platform that we know is basically safe and effective against this class of virus. Specific immunogenicity testing could be started immediately and finished within a few weeks.
This should be combined with stockpiles of complementary goods and essential inputs for rapidly scaling vaccine manufacturing and distribution. Florian Kramer, a vaccine researcher who laid out this plan in a 2020 journal article, estimates that this would cost 20-30 million dollars per vaccine. Combined with the stockpiles of complementary goods, this plan would have a total cost of 4 to 6 billion dollars upfront, with smaller recurring costs for upkeep. This might sound like a lot, but it is less than a tenth of a percent of what the US government has already spent on economic stimulus over the past 2 years. If this plan can stop one pandemic in a thousand years it will have already more than paid for itself.
This plan is so simple and sensible that people have been proposing it for decades before the Covid pandemic. Unfortunately, the same selective enforcement of Type I errors which led our government to reject human challenge trials, continue to make long-term planning for pandemics politically unpopular. Solving these political issues is incredibly difficult. This paper does not propose a solution which would align political incentives with the most effective pandemic responses. It only takes the first step in showing the gains from improving these political incentives are measured in hundreds of thousands of human lives.
A title like "How many lives might have been saved given an earlier COVID-19 vaccine rollout?" would have given me much more information about what the post was about than the current title, which I find very vague.
Huh? 40 million doses of the 1957 flu vaccine were delivered within about 6 months of getting a virus sample to the US. Does that not count due to its similarity to existing vaccines?
I'm pretty sure that with a sufficiently bad virus, it's safer to vaccinate before effectiveness is known. We ought to plan ahead for how to make such a decision.