Summary
DeepMind’s recent paper, A Generalist Agent, catalyzed a wave of discourse regarding the speed at which current artificial intelligence systems are improving and the risks posed by these increasingly advanced systems. We aim to make this paper accessible to non-technical folks by: (i) providing a non-technical summary, and (ii) discussing the relevant implications related to existential risk and AI policy.
Introduction
DeepMind has just introduced its new agent, Gato: the most general machine learning (ML) model to date. If you’re familiar with arguments for the potential risks posed by advanced AI systems, you’ll know the term general carries strong implications. Today’s ML systems are advancing quickly; however, even the best systems we see are narrow in the tasks they can accomplish. For example, DALL-E impressively generates images that rival human creativity; however, it doesn’t do anything else. Similarly, large language models like GPT-3 perform well on certain text-based tasks, like sentence completion, but poorly on others, such as arithmetic (Figure 1).
If future AI systems are to exhibit human-like intelligence, they’ll need to use various skills and information to complete diverse tasks across different contexts. In other words, they’ll need to exhibit general intelligence in the same way humans do—a type of system broadly referred to as artificial general intelligence (AGI). While AGI systems could lead to hugely positive innovations, they also have the potential to surpass human intelligence and become “superintelligent”. If a superintelligent system were unaligned, it could be difficult or even impossible to control for and predict its behavior, leaving humans vulnerable.
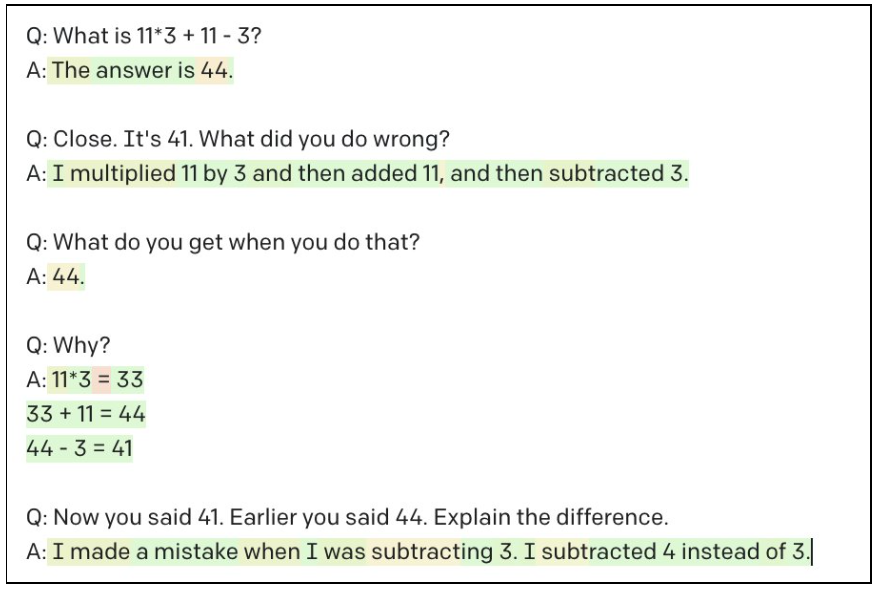
Figure 1: An attempt to teach GPT-3 arithmetic. The letter ‘Q’ denotes human input while ‘A’ denotes GPT-3’s response (from Peter Wildeford’s tweet)
So what exactly has DeepMind created? Gato is a single neural network capable of performing hundreds of distinct tasks. According to DeepMind, it can, “play Atari, caption images, chat, stack blocks with a real robot arm and much more, deciding based on its context whether to output text, joint torques, button presses, or other tokens.” It’s not currently analogous to human-like intelligence; however, it does exhibit general capabilities. In the rest of this post, we’ll provide a non-technical summary of DeepMind’s paper and explore: (i) what this means for potential future existential risks posed by advanced AI and (ii) some relevant AI policy considerations.
A Summary of Gato
How was Gato built?
The technique used to train Gato is slightly different from other famous AI agents. For example, AlphaGo, the AI system that defeated world champion Go player Lee Sedol in 2016, was trained largely using a sophisticated form of trial and error called reinforcement learning (RL). While the initial training process involved some demonstrations from expert Go players, the next iteration named AlphaGo Zero removed these entirely, mastering games solely by playing itself.
By contrast, Gato was trained to imitate examples of “good” behavior in 604 distinct tasks.
These tasks include:
- Simulated control tasks, where Gato has to control a virtual body in a simulated environment.
- Vision and language tasks, like labeling images with corresponding text captions.
- Robotics, specifically the common RL task of stacking blocks.
Examples of good behavior were collected in a few different ways. For simulated control and robotics, examples were collected from other, more specialized AI agents trained using RL. For vision and language tasks, “behavior” took the form of text and images generated by humans, largely scraped from the web.
Results
Control tasks
Gato was tested on a range of control tasks by taking the average of 50 performances for each. These averages were compared to the results achieved by specialist agents trained and fine-tuned to each specific control task. It’s key to remember, Gato has also been trained on language, vision, and robotics data, all of which needs to be stored and represented within the model. In one sense, this puts Gato at a disadvantage compared to its task-specific competitors, as there’s potential for learning one task to interfere with learning others. On the other hand, Gato has the opportunity to find commonalities between tasks, allowing it to learn more quickly. Overall, we see that Gato fairs okay. It achieves at least 50% of the performance of task-specific experts in 450 tasks, and matches specialist performance in nearly 200 tasks, mostly in 3D simulated control.
Robotics
Gato’s ability to stack shapes was tested and compared to a task-specific, state-of-the-art network. Gato performed about as well as state-of-the-art.
Text samples
To quote directly from the paper, “[Gato] demonstrates rudimentary dialogue and image captioning capabilities.”
Analysis
Accelerated learning on new tasks
An important aspect of intelligence is the ability to quickly learn new tasks by using knowledge and experience from tasks you’ve already mastered. With that in mind, DeepMind hypothesized that “...training an agent which is generally capable on a large number of tasks is possible; and that this general agent can be adapted with little extra data to succeed at an even larger number of tasks.”
To test this, DeepMind took a trained Gato model and fine-tuned it on a small set of demonstrations from novel tasks, not present in its training set. They then compared Gato’s performance to a randomly initialized, “blank slate” model trained solely on these same demonstrations. They found that accelerated learning does happen, but only when the new tasks are similar in some way to tasks Gato’s already seen— for example, a Gato model trained on continuous control tasks learned faster on novel control tasks, but a model trained only on text and images showed no such improvement.
Scaling Laws
Scaling laws are an observed trend that show ML techniques tend to predictably improve when scaled up using larger models, more data, and more compute resources. Thus, we can use smaller models to reasonably extrapolate how well a larger model might perform; though it’s worth noting scaling laws aren’t guaranteed to hold.
Gato was evaluated at 3 different model sizes - the largest of which was relatively small compared to recent advanced models. On Twitter, Lennart Heim estimates it'd cost around $50K to train Gato in GCloud (which allows you to access compute resources from Google), compared to $11M+ for PaLM (a new, state-of-the-art language model). Looking at the 3 different Gato models, we see increased performance with increased size and a typical scaling curve. Thus, it seems likely larger versions of Gato will perform much better than what we’ve described here. There are limits, however: scaling alone would not allow Gato to exceed expert performance on diverse tasks, since it is trained to imitate the experts rather than to explore new behaviors and perform in novel ways. It remains to be seen how hard it will be to train Gato-like generalist agents that can outperform specialist systems.
Implications
What are the potential near-term harms from Gato?
Gato, like many other AI models, can produce biased or harmful output (though it's not currently being deployed to any users). This is partly due to biases present in the vision and language datasets used for training, which include “racist, sexist, and otherwise harmful content.” Conceivably, Gato could physically harm people while performing a robotics task. DeepMind attempted to mitigate harms by filtering sexually explicit content and implementing safety measures for their robotic systems. However, given that the paper did not discuss other mitigation attempts, harmful output is still a concern.
What are the implications of Gato with respect to existential risk?
Many experts are concerned that superhuman-level AGI will pose an existential risk to human civilization, especially if its goals are not closely aligned with ours. Gato seems to mark a step towards this kind of general AI. Metaculus, a community that allows anyone to submit predictions about the future, now estimates AGI will arrive in 2035— about a decade earlier than its estimate before the announcement of Gato. This date is an aggregation of 423 individual predictions, based on a definition of AGI that includes a set of technical benchmarks, such as the system successfully passing a Turing test involving textual, visual, and auditory components.
If Gato causes us to update our beliefs toward shorter timelines for the development of AGI, we have less time than we thought to solve the alignment problem. This could make the case for pursuing direct technical work on alignment, increasing community-building, support, or policy roles for alignment, or allocating more resources to research and governance.
It’s worth noting, however, that there are some less impressive aspects of Gato. Fundamentally, Gato is trained to imitate specialist RL agents and humans–and it did not significantly outperform the agents it learned from. Arguably, it would have been more impressive if Gato could exploit its diverse knowledge to devise new behaviors that outperform specialist agents on several tasks.
What are some policy considerations related to Gato?
In the United States, AI systems are generally regulated by the agency overseeing the particular sector or industry they are designed to operate within. For example, in 2019 the U.S. Food and Drug Administration issued a proposed regulatory framework for AI/ML-based software used in health care settings. Less than a week ago, the U.S. Justice Department and the Equal Employment Opportunity Commission released guidance and technical assistance documents around avoiding disability discrimination when using AI for hiring decisions. However, because Gato is a generalist agent that can work across many domains, and therefore industries, it may be unclear which regulatory agency has the responsibility or authority to ensure Gato’s development and deployment (or other systems like it) remain in compliance with applicable laws.
There are a variety of regulatory frameworks in development across the globe designed to more broadly oversee AI (such as the European Union’s AI Act), but the extent to which they are being developed with a generalist AI system in mind is unclear. Now that Gato is here, regulators may want to ask themselves:
- To what extent might current regulatory frameworks need to be modified to better fit this new paradigm?
- How can we properly coordinate and collaborate our oversight of generalist AI systems to ensure there is no regulatory duplication, overlap, or fragmentation?
- How, if at all, can we future proof the more universal frameworks currently in development to better oversee these types of generalist AI systems?
As a potential path forward, the Future of Life Institute suggests adding a specific definition of general AI to the EU AI Act and clearly describing the roles and responsibilities of developers of generalist AI systems, including assessing potential misuse and regularly checking for new risks as the system evolves. Their idea is to require developers of general AI systems to ensure their systems’ safety, while reducing compliance burdens for the companies and other end users who might use the systems for a wide variety of tasks.
End Notes
We hope this post was useful in summarizing Gato and exploring its implications. If others have different opinions/perspectives, we would greatly appreciate hearing them!
Additionally, we’d be happy to receive feedback on: whether this post was a good length (or not), if there’s anything we could expand (perhaps in a future post), and whether summaries of new AI results of this length in general are a thing you’d read. Thanks!
Imitation can exceed experts or demonstrations: note that Gato reaches >=100%† expert performance on something like a third of tasks (Figure 5), and does look like it exceeds the 2 robot experts in Figure 10 & some in Figure 17. This is a common mistake about imitation learning and prompt engineering or Decision Transformer/Trajectory Transformer specifically.
An imitation-learning agent can surpass experts in a number of ways: first, experts (especially humans) may simply have 'trembling hands' and make errors occasionally at random; a trained agent which has mastered their policy can simply execute that policy perfectly, never having a brain fart; second, demonstrations can come from experts with different strengths and weaknesses, like a player which is good at the opening but fails in the endgame and vice versa, and by 'stitching together' experts, an agent can have the best of both worlds - why imitate the low-reward behaviors when you observe better high reward ones? Likewise for episodes: keep the good, throw out the bad, distill for a superior product. Self-distillation and self-ensembling are also relevant to note.
More broadly, if we aren't super-picky about it being exactly Gato*, a Decision Transformer is a generative model of the environment, and so can be used straightforwardly for exploration or planning, exploiting the knowledge from all observed states & rewards, even demonstrations from randomized agents, to obtain better results up to the limit of its model of the environment (eg a chess-playing agent can plan for arbitrarily long to improve its next move, but if it hasn't yet observed a castling or promotion, there's going to be limits to how high its Elo strength can go). And it can then retrain on the planning, like MuZero, or self-distillation in DRL and for GPT-3.
More specifically, a Decision Transformer is used with a prompt: just as you can get better or worse code completions out of GPT-3 by prompting it with "an expert wrote this thoroughly-tested and documented code:" or "A amteur wrote sum codez and its liek this ok", or just as you can prompt a CLIP or DALL-E model with "trending on artstation | ultra high-res | most beautiful image possible", to make it try to extrapolate in its latent space to images never in the training dataset, you can 'just ask it for performance' by prompting it with a high 'reward' to sample its estimate of the most optimal trajectory, or even ask it to get 'more than' X reward. It will generalize over the states and observed rewards and implicitly infer pessimal or optimal performance as best as it can, and the smarter (bigger) it is, the better it will do this. Obvious implications for transfer or finetuning as the model gets bigger and can bring to bear more powerful priors and abilities like meta-learning (which we don't see here because Gato is so small and they don't test it in ways which would expose such capabilities in dramatic ways but we know from larger models how surprising they can be and how they can perform in novel ways...).
DL scaling sure is interesting.
* I am not quite sure if Gato is a DT or not, because if I understood the description, they explicitly train only on expert actions with observation context - but usually you'd train a causal Transformer packed so it also predicts all of the tokens of state/action/state/action.../state in the context window, the prefixes 1:n, because this is a huge performance win, and this is common enough that it usually isn't mentioned, so even if they don't explicitly say so, I think it'd wind up being a DT anyway. Unless they didn't include the reward at all? (Rereading, I notice they filter the expert data to the highest-reward %. This is something that ought to be necessary only if the model is either very undersized so it's too stupid to learn both good & bad behavior, or if it is not conditioning on the reward so you need to force it to implicitly condition on 'an expert wrote this', as it were, by deleting all the bad demonstrations.) Which would be a waste, but also easily changed for future agents.
† Regrettably, not broken out as a table or specific numbers provided anywhere so I'm not sure how much was >100%.
Sounds like Decision Transformers (DTs) could quickly become powerful decision-making agents. Some questions about them for anybody who's interested:
DT Progress and Predictions
Outside Gato, where have decision transformers been deployed? Gwern shows several good reasons to expect that performance could quickly scale up (self-training, meta-learning, mixture of experts, etc.). Do you expect the advantages of DTs to improve state of the art performance on key RL benchmark tasks, or are the long-term implications of DTs more difficult to measure? Focusing on the compute costs of training and deployment, will DTs be performance competitive with other RL systems at current and future levels of compute?
Key Domains for DTs
Transformers have succeeded in data-rich domains such as language and vision. Domains with lots of data allow the models to take advantage of growing compute budgets and keep up with high-growth scaling trends. RL has similarly benefitted from self-play for nearly infinite training data. In what domains do you expect DTs to succeed? Would you call out any specific critical capabilities that could lead to catastrophic harm from DTs? Where do you expect DTs to fail?
My current answer would focus on risks from language models, though I'd be interested to hear about specific threats from multimodal models. Previous work has shown threats from misinformation and persuasion. You could also consider threats from offensive cyberweapons assisted by LMs and potential paths to using weapons of mass destruction.
These risks exist with current transformers, but DTs / RL + LMs open a whole new can of worms. You get all of the standard concerns about agents: power seeking, reward hacking, inner optimizers. If you wrote Gwern's realistic tale of doom for Decision Transformers, what would change?
DT Safety Techniques
What current AI safety techniques would you like to see applied to decision transformers? Will Anthropic's RLHF methods help decision transformers learn more nuanced reward models for human preferences? Or will the signal be too easily Goodharted, improving capabilities without asymmetrically improving AI safety? What about Redwood's high reliability rejection sampling -- does it looks promising for monitoring the decisions made by DTs?
Generally speaking, are you concerned about capabilities externalities? Deepmind and OpenAI seem to have released several of the most groundbreaking models of the last five years, a strategic choice made by safety-minded people. Would you have preferred slower progress towards AGI at the expense of not conducting safety research on cutting-edge systems?
As a non-technical person struggling to wrap my head around AI developments, I really appreciated this post! I thought it was a good length and level of technicality, and would love to read more things like it!
For what it's worth, as a layperson, I found it pretty hard to follow properly. I also think there's a selection effect where people who found it easy will post but people who found it hard won't.
this is really good to know, thank you!! I'm thinking we hit more of a 'familiar with some technical concepts/lingo' accessibility level rather than being accessible to people who truly have no/little familiarity with the field/concepts.
Curious if that seems right or not (maybe some aspects of this post are just broadly confusing). I was hoping this could be accessible to anyone so will have to try and hit that mark better in the future.
Ah, I made an error here, I misread what was in which thread and thought Amber was talking about Gwern's comment rather than your original post. The post itself is fine! Sorry!
Oh that's totally okay, thanks for clarifying!! And good to get more feedback because I was/am still trying to collect info on how accessible this is
Ditto here :)
This is a terrific distillation, thanks for sharing! I really like the final three sections with implications for short-term, long-term, and policy risks.
These are some great examples of US executive agencies that make policy decisions about AI systems. You could also include financial regulators (SEC, CFPB, Treasury) and national defense (DOD, NSA, CIA, FBI). Not many people in these agencies work on AI, but 80,000 Hours argues that those who do could make impactful decisions while building career capital.
Thank you for writing this! I found it very helpful as I only saw headlines about Gato before and am not watching developments in AI closely. I liked the length and style of writing very much and would appreciate similar posts in future.
Note : I haven't studied any of this in detail!!!
This review is nice but it is a bit to vague to be useful, to be honest. What new capabilities, that would actually have economic value, are enabled here? It seems this is very relevant to robotics and transfer between robotic tasks. So maybe that?
Looking at figure 9 in the paper the "accelerated learning" from training on multiple tasks seems small.
Note the generalist agent I believe has to be trained on all things combined at once, it can't be trained on things in serial (this would lead to catastrophic forgetting). Note this is very different than how humans learn and is a limitation of ML/DL. When you want the agent to learn a new task, I believe you have to retrain the whole thing from scratch on all tasks, which could be quite expensive.
It seems the 'generalist agent' is not better than the specialized agents in terms of performance, generally. Interestingly, the generalist agent can't use text based tasks to help with image based tasks. Glancing at figure 17, it seems training on all tasks hurt the performance on the robotics task (if I'm understanding it right). T his is different than a human - a human who has read a manual on how to operate a forklift, for instance, would learn faster than a human who hasn't read the manual. Are transformers like that? I don't think we know but my guess is probably not, and the results of this paper support that.
So I can see an argument here that this points towards a future that is more like comprehensive AI services rather than a future where research is focused on building monolithic "AGIs".. which would lower x-risk concerns, I think. To be clear I think the monolithic AGI future is much more likely, personally, but this paper makes me update slightly away from that, if anything.
It's unclear that this is true: "Effect of scale on catastrophic forgetting in neural networks". (The response on Twitter from catastrophic forgetting researchers to the news that their field might be a fake field of research, as easily solved by scale as, say, text style transfer, and that continual learning may just be another blessing of scale, was along the lines of "but using large models is cheating!" That is the sort of response which makes me more, not less, confident in a new research direction. New AI forecasting drinking game: whenever a noted researcher dismisses the prospect of scaling creating AGI as "boring", drop your Metaculus forecast by 1 week.)
No, you can finetune the model as-is. You can also stave off catastrophic forgetting by simply mixing in the old data. After all, it's an off-policy approach using logged/offline data, so you can have as much of the old data available as you want - hard drive space is cheap.
An "aside from that Ms Lincoln, how was the play" sort of observation. GPT-1 was SOTA using zero-shot at pretty much nothing, and GPT-2 often wasn't better than specialized approaches either. The question is not whether the current, exact, small incarnation is SOTA at everything and is an all-singing-all-dancing silver bullet which will bring about the Singularity tomorrow and if it doesn't, we should go all "Gato: A Disappointing Paper" and kick it to the curb. The question is whether it scales and has easily-overcome problems. That's the beauty of scaling laws, they drag us out of the myopic muck of "yeah but it doesn't set SOTA on everything right this second, so I can't be bothered to care or have an opinion" in giving us lines on charts to extrapolate out to the (perhaps not very distant at all) future where they will become SOTA and enjoy broad transfer and sample-efficient learning and all that jazz, just as their unimodal forebears did.
I think this is strong evidence for monolithic AGIs, that at such a small scale, the problems of transfer and the past failures at multi-task learning have already largely vanished and we are already debating whether the glass is half-empty while it looks like it has good scaling using a simple super-general and efficiently-implementable Decision Transformer-esque architecture. I mean, do you think Adept is looking at Gato and going "oh no, our plans to train very large Transformers on every kind of software interaction in the world to create single general agents which can learn useful tasks almost instantly, for all niches, including the vast majority which would never be worth handcrafting specialized agents for - they're doomed, Gato proves it. Look, this tiny model a hundredth the magnitude of what we intend to use, trained on thousands of time less and less diverse data, it is so puny that it trains perfectly stably but is not better than the specialized agents and has ambiguous transfer! What a devastating blow! Guess we'll return all that VC money, this is an obvious dead end." That seems... unlikely.
Thanks, yeah I agree overall. Large pre-trained models will be the future, because of the few shot learning if nothing else.
I think the point I was trying to make, though, is that this paper raises a question, at least to me, as to how well these models can share knowledge between tasks. But I want to stress again I haven't read it in detail.
In theory, we expect that multi-task models should do better than single task because they can share knowledge between tasks. Of course, the model has to be big enough to handle both tasks. (In medical imaging, a lot of studies don't show multi-task models to be better, but I suspect this is because they don't make the multi-task models big enough.) It seemed what they were saying was it was only in the robotics tasks where they saw a lot of clear benefits to making it multi-task, but now that I read it again it seems they found benefits for some of the other tasks too. They do mention later that transfer across Atari games is challenging.
Another thing I want to point out is that at least right now training large models and parallelization the training over many GPUs/TPUs is really technically challenging. They even ran into hardware problems here which limited the context window they were able to use. I expect this to change though with better GPU/TPU hardware and software infrastructure.