cross-posted to LW here.
Introduction
As in 2016, 2017, 2018, and 2019, I have attempted to review the research that has been produced by various organisations working on AI safety, to help potential donors gain a better understanding of the landscape. This is a similar role to that which GiveWell performs for global health charities, and somewhat similar to a securities analyst with regards to possible investments.
My aim is basically to judge the output of each organisation in 2020 and compare it to their budget. This should give a sense of the organisations' average cost-effectiveness. We can also compare their financial reserves to their 2021 budgets to get a sense of urgency.
I’d like to apologize in advance to everyone doing useful AI Safety work whose contributions I have overlooked or misconstrued. As ever I am painfully aware of the various corners I have had to cut due to time constraints from my job, as well as being distracted by 1) other projects, 2) the miracle of life and 3) computer games.
This article focuses on AI risk work. If you think other causes are important too, your priorities might differ. This particularly affects GCRI, FHI and CSER, who both do a lot of work on other issues which I attempt to cover but only very cursorily.
How to read this document
This document is fairly extensive, and some parts (particularly the methodology section) are largely the same as last year, so I don’t recommend reading from start to finish. Instead, I recommend navigating to the sections of most interest to you.
If you are interested in a specific research organisation, you can use the table of contents to navigate to the appropriate section. You might then also want to Ctrl+F for the organisation acronym in case they are mentioned elsewhere as well. Papers listed as ‘X researchers contributed to the following research lead by other organisations’ are included in the section corresponding to their first author and you can Cntrl+F to find them.
If you are interested in a specific topic, I have added a tag to each paper, so you can Ctrl+F for a tag to find associated work. The tags were chosen somewhat informally so you might want to search more than one, especially as a piece might seem to fit in multiple categories.
Here are the un-scientifically-chosen hashtags:
- AgentFoundations
- Amplification
- Capabilities
- Corrigibility
- DecisionTheory
- Ethics
- Forecasting
- GPT-3
- IRL
- Misc
- NearAI
- OtherXrisk
- Overview
- Politics
- RL
- Strategy
- Textbook
- Transparency
- ValueLearning
New to Artificial Intelligence as an existential risk?
If you are new to the idea of General Artificial Intelligence as presenting a major risk to the survival of human value, I recommend this Vox piece by Kelsey Piper, or for a more technical version this by Richard Ngo.
If you are already convinced and are interested in contributing technically, I recommend this piece by Jacob Steinheart, as unlike this document Jacob covers pre-2019 research and organises by topic, not organisation, or this from Critch & Krueger, or this from Everitt et al, though it is a few years old now
Research Organisations
FHI: The Future of Humanity Institute
FHI is an Oxford-based Existential Risk Research organisation founded in 2005 by Nick Bostrom. They are affiliated with Oxford University. They cover a wide variety of existential risks, including artificial intelligence, and do political outreach. Their research can be found here.
Their research is more varied than MIRI's, including strategic work, work directly addressing the value-learning problem, and corrigibility work - as well as work on other Xrisks.
They run a Research Scholars Program, where people can join them to do research at FHI. There is a fairly good review of this here. Unfortunately I suspect the pandemic may have reduced its effectiveness this year, as FHI has often favoured informal networking rather than formal management structures, but it seems to have worked well pre and hopefully post pandemic.
The EA Meta Fund supported a special program for providing infrastructure and support to FHI, called the Future of Humanity Foundation. This reminds me somewhat of what BERI does.
In the past I have been very impressed with their work.
Research
Bostom & Shulman's Sharing the World with Digital Minds discusses the moral issues raised by the potential for uploads or other digital minds. By virtue of their number, speed, or specific design, these could be utility monsters - a term from Nozick for agents much more efficient than humans at turning resources into utility. Would we therefore be obliged to give up all our resources to them and eventually let meat humanity starve to death? This much has been discussed before - indeed, I alluded to this as an argument against a universal basic income as a response to AI-driven unemployment in previous versions of this article! - but this article both provides a canonical reference and also a good survey showing that such issues come up under a wide variety of ethical views and technological possibilities. I also enjoyed the discussion of the issues posed by rapid reproduction for 'democratic' political systems, where influence is the scarce resource. #Strategy
Ashurst et al.'s A Guide to Writing the NeurIPS Impact Statement gives advice on how to write the new 'impact statements' that NeurIPS now requires. Seizing this gap in the market by writing the canonical piece that everyone will find when they google - my tests suggest they have the SEO - and filling it with a counterfactually valuable article is some good out-of-the-box thinking. As well as containing many very useful links, I liked the suggestion that even theoretical pieces should consider their impacts. #Misc
Kovařík & Carey's (When) Is Truth-telling Favored in AI Debate? provides some formalism and theorems around the properties of debate. I thought the section about debate length was very interesting, where it seems to show (at least for this class of debate) that debates are either long enough to produce the truth in a trivial manner (through full exposition) or else error can be arbitrarily high with even one fewer step, though they also identified plausible seeming sub-classes with much better performance. (the paper is technically from the very end of 2019 but I missed it last year) See also the discussion here. #Amplification
Shevlane & Dafoe's The Offense-Defense Balance of Scientific Knowledge: Does Publishing AI Research Reduce Misuse? discusses whether increased AI publishing will generally be more useful for 'attack' or 'defence'. They argue that the 'publishing exploits is generally best practice (with a lag)' model from cybersecurity might not be best placed here - an important argument to rebut, as many people used it to criticise OpenAI's decision to be (initially) clopen with regard GPT-2. #Strategy
Ord's The Precipice provides a detailed overview of existential risks and the future of humanity. It covers a variety of risks, including a good section on AGI, which Toby estimates as the largest risk at ~ 10% / century. There is also a huge amount of other material covered, including some novel ideas to me like the section on risk correlations, as well as some very motivational final chapters. I was pleasantly surprised to learn that 80% of DNA synthesis was being screened (in some way) for dangerous compounds. Probably replaces Bostrom and Ćirković as the best book on the subject now. #Overview
Carey et al.'s The Incentives that Shape Behaviour attempts to build a general theory of what sort of incentives lead agents to manipulate humans. This is basically causal diagram classification, revealing incentives to control and react to humans. It includes examples for both fairness incentives and also a possible way of reducing human manipulation incentivisation: optimising for a separately trained predictor. See also the discussion here. Researchers from Deepmind were also named authors on the paper. #AgentFoundations
Clarke's Clarifying “What failure looks like” (part 1) attempts a more detailed analysis of the issues raised in Christiano's What failure looks like I liked the breakdown of lock-in mechanisms, which seem true to me.It provides a lot of examples, some of which I liked, like that of the Maori. However many of them were sufficiently simplified that I feel significant disanalogies were overlooked - for example, the Climate Change example neglects the very different incentives facing regulated utilities, and the agricultural revolution example seems to require a strong commitment to average utilitarianism, even though this is not a popular view of population ethics. Despite this I thought the underlying argument seemed pretty plausible. #Forecasting
Armstrong et al.'s Pitfalls of Learning a Reward Function Online introduces two desirable properties for agents who are trying to learn human values at runtime (unriggability and uninfluenceability) and proves they are broadly the same thing. As well as proving this result, it contains a series of examples of what can go wrong in the absence of either property - including sacrificing reward with probability 100% - and a brief discussion of how counterfactual rewards might address the problem. It ends with an extended gridworld example, but I found this a little hard to follow. See also the discussion here. Researchers from Deepmind were also named authors on the paper. #ValueLearning
Tucker et al.'s Social and Governance Implications of Improved Data Efficiency discusses some of the strategic implications of ML systems that do not require as much data. They argue that it is not obvious that they will net benefit smaller firms - if the impact is multiplicative, it might benefit larger firms with more compliments (like market access) more - though I am not sure a multiplicative effect is really a good model for what people are thinking about when they talk about ML models needing less data. They also point out that due to threshold effect this might enable entirely new applications, and in particular IRL/amplification, as these rely on a very scarce source of data: humans. #Forecasting
Cohen & Hutter's Curiosity Killed the Cat and the Asymptotically Optimal Agent show that because any agent that is guaranteed to eventually find the optimal strategy can only do so by testing every option, any 'traps' in the environment will eventually be triggered with probability 1. (Unless traps are disabled after finite time). This is clearly kinda important - it is nice to be able to reason about asymptotic optimality, but we do not want an AGI that deletes humanity with p=1 en route. This suggests something of a bootstrap problem, where we need a 'mentor' to avoid such dangers. Researchers from Deepmind were also named authors on the paper. #RL
Cohen & Hutter's Pessimism About Unknown Unknowns Inspires Conservatism basically tries to make a conservative AIXI that defers to its mentor when it is not sure. It does this by comparing its worst-case estimates to its estimate of the mentor's expected case, and defers to the mentor more when the difference is higher (and less as t->oo). Hopefully the mentor will help keep the agent from being too conservative, as it seems there is a risk that it simply ends up doing nothing, and gets out-competed by an EV maximising agent? Researchers from Deepmind were also named authors on the paper. #RL
Nguyen & Christiano's My Understanding of Paul Christiano's Iterated Amplification AI Safety Research Agenda provides an overview of Paul's IDA agenda. Probably the best such explanation so far; written by Chi when she was at FHI with in-line comments from Paul. Researchers from OpenAI were also named authors on the paper. #Amplification
Snyder-Beattie et al.'s The Timing of Evolutionary Transitions Suggests Intelligent Life Is Rare builds a bayesian model to try to get around the anthropic problem of estimating how easy it is for life to develop. Specifically, they use non-informative priors and update based on the distribution of various transitions (e.g. Eukaryotes), concluding (similar to previous work they cite) that the development of life is relatively hard. See also the discussion here. #Forecasting
Ding & Dafoe's The Logic of Strategic Assets: From Oil to AI analyses what causes a product to be 'strategic' to a country. They decompose this into the product of its Importance, Externalities and Rivalosity, in contrast to previous analysis of simply 'military importance'. Some of the examples I might quibble with - for example, the paper claims that the spillovers from railways lead private agents to underinvest, which is somewhat in tension with the experience of the railway bubbles. I am also a bit sceptical that this analysis really subsumes the idea of dependency-strategic items - nitrates in WWI, and nuclear weapons now, both lack substitutes and are at risk of supply disruptions, but neither really seem to have massive externalities. It also would have been nice to see some analysis of why individual firms do not internalise the risk of supply disruption - is this due to anti-price gouging laws? It finishes with detailed discussion of two examples - British Jet Engines (reminding me of Attlee's disastrous mistake with another type of engine ) and US-Japanese rivalry. The report discusses several mistakes US policy made during this period - e.g. accidentally classifying cash registers as strategic, and missing rayon fibers - but these mistakes seem like they are adequately explained without the theory put forward by the paper. #NearAI
Cotton-Barratt et al.'s Defence in Depth Against Human Extinction: Prevention, Response, Resilience, and Why They All Matter provides a series of taxonomies for existential risks. In particular, they discuss distinctions between preventing and mitigating events, how events scale to be global, and how direct their effect is. See also the discussion here. #Strategy
Cihon et al.'s Should Artificial Intelligence Governance be Centralised? Design Lessons from History discusses the advantages of centralised or fragmented international law approaches to AI. Most of the considerations are not AI specific. Researchers from CSER were also named authors on the paper. #Strategy
O'Brien & Nelson's Assessing the Risks Posed by the Convergence of Artificial Intelligence and Biotechnology discusses the impact of AI on biorisk. They first discuss the problems with several existing frameworks and the potential impact of AI on bio risk, before offering their own framework. #OtherXrisk
Cremer & Whittlestone's Canaries in Technology Mines: Warning Signs of Transformative Progress in AI attempt to identify possible signs of imminent AGI though expert-solicitation of causal influence diagrams. Basically a technology that is seen as a prerequisite for many others is a candidate for being a canary. However, I didn't feel the paper really addressed the issues raised in Eliezer's Fire Alarm post. Researchers from CSER were also named authors on the paper. #Forecasting
O'Keefe's How will National Security Considerations affect Antitrust Decisions in AI? An Examination of Historical Precedents surveys a bunch of historical antitrust actions in the US to see how national security arguments played into the outcome. He finds that it was pretty rare, especially recently, and when it did it was generally congruent with the main antitrust objectives, namely preventing artificial reductions in output. The idea here presumably is to suggest that the US government is unlikely to use antitrust as a tool in an AI race unless firms start overcharging for their services. O’Keefe also lists support from OpenPhil. #Politics
Bostrom et al.'s Written Evidence to the UK Parliament Science & Technology Committee's Inquiry on A new UK research funding agency. recommends that Cumming's new British DARPA focus on existential risks. I think this a worthwhile but big ask - DARPA seems more intended to fund risky things than to reduce risk - and now Cummings has left I worry the window for intervention here may have passed. Researchers from CSER were also named authors on the paper. #Politics
O'Keefe et al.'s The Windfall Clause: Distributing the Benefits of AI for the Common Good proposes that AI firms voluntarily commit to donating some % of profits over a high threshold to humanity in general. The idea is that the cost of this commitment is currently negligible, but would be extremely socially valuable if one firm gained a decisive strategic advantage. I think it's good to work on novel governance strategies, but I'm not very enthusiastic about this specific option, partly for reasons I outlined in lengthy but unfinished comments on the forum post, but mainly because I don't think it does much to reduce the existential risk, especially vs similar ideas like encouraging consolidation among AI firms. See also the discussion here. #Politics
Garfinkel's Does Economic History Point Towards a Singularity? and the associated document analyse the claim that economic growth has been accelerating in accordance with global GDP (or population). In general it finds the evidence for this to be somewhat weak. #Forecasting
Prunkl & Whittlestone's Beyond Near- and Long-Term: Towards a Clearer Account of Research Priorities in AI Ethics and Society proposes alternative divisions of the AI safety community other than near vs long term. These are: impacts, capabilities, certainty and scale. The paper argues that we should focus on these axis because 1) there is variance that is overlooked by a single short-vs-long axis and 2) this can cause misunderstandings. I did not really find this convincing: the purpose of any clustering is to summarize data, and I have yet to come across any examples of confusions that would be dispelled by their alternative axes. In fact, their motivating example - that of Etzioni's misreading of Bostrom - is a case where relying on the 'long term' stereotype would have given Etzioni more accurate beliefs! Similarly, their examples of 'intermediate' issues, like the long-term impact on inequality of algorithmic discrimination, seems to me like precisely the sort of political (and in my opinion mistaken) concern that everyone would agree falls into the 'short-term' camp. But perhaps, like Cave & Ó hÉigeartaigh, this paper is better understood as a speech act. See also the discussion here. Researchers from Leverhulme were also named authors on the paper. #Strategy
FHI researchers contributed to the following research led by other organisations:
- Brundage et al.'s Toward Trustworthy AI Development: Mechanisms for Supporting Verifiable Claims
- Scholl & Hanson's Testing the Automation Revolution Hypothesis
They also produced a variety of pieces on biorisk and other similar subjects, which I am sure are very good and important but I have not read.
According to Riedel & Deibel, over the 2016-2020 period, FHI accounted for by far the largest number of citations for meta-AI-safety work, and a respectable showing in technical AI safety.
Finances
FHI didn’t reply to my emails about donations, and seem to be more limited by talent than by money.
If you wanted to donate to them anyway, here is the relevant web page.
"edit (2020-12-26): FHI subsequently did reach out to inform me that while they could not share the information financial etc. information I requested they would still appreciate donations."
CHAI: The Center for Human-Aligned AI
CHAI is a UC Berkeley based AI Safety Research organisation founded in 2016 by Stuart Russell.. They do ML-orientated safety research, especially around inverse reinforcement learning, and cover both near and long-term future issues. One outside interpretation of their work from Alex Flint is here.
As an academic organisation their members produce a very large amount of research; I have only tried to cover the most relevant below. It seems they do a better job engaging with academia than many other organisations, especially in terms of interfacing with the cutting edge of non-safety-specific research. The downside of this, from our point of view, is that not all of their research is focused on existential risks.
Rohin Shah, now with additional help, continues to produce the AI Alignment Newsletter, covering in detail a huge number of interesting new developments, especially new papers. I really cannot praise these newsletters highly enough. Unfortunately for CHAI, but probably fortunately for the world, he has graduated and is moving to Deepmind.
They have expanded somewhat to other universities outside Berkeley and have people at places like Princeton and Cornell.
Research
CHAI and their associated academics produce a huge quantity of research. Far more so than other organisations their output is under-stated by my survey here; if they were a small organisation that only produced one report, there would be 100% coverage, but as it is this is just a sample of those pieces I felt most interested in. On the other hand academic organisations tend to produce some slightly less relevant work also, and I have focused on what seemed to me to be the top pieces.
Critch & Krueger's AI Research Considerations for Human Existential Safety (ARCHES) is a super-detailed overview of the state of the field, and a research agenda. It provides a detailed explanation of key concepts and a categorisation schema of various possible scenarios, including new distinctions I hadn't seen clearly made before. This is a mammoth document, and I encourage the reader to attempt it if possible. A few interesting points for me were his argument that AI reseacher's discussions of 'near' AI problems as being the first steps towards admitting problems, or that Distributional Shift work might not be not neglected by Industry? Contrary to some others he argues that we should perhaps never make 'prepotent' AI (one that cannot be controlled by humans) - not even a defensive one to prevent other AI threats. There is also a lot of discussion of multi-polar scenarios - the idea that single agent alignment/delegation problems are less important to focus on, partly because the single-agent version is more likely to be solved by profit-maximising firms. See also the discussion here. Researchers from BERI were also named authors on the paper. #Overview
Andreea et al.'s LESS is More: Rethinking Probabilistic Models of Human Behavior attempts to extend the model of Boltzmann rationality (where humans choose the best option, with noise, from a finite menu) to the continuous case. This is essentially by providing continuous measures of how 'similar' different options are, to show that e.g. driving at 41mph and 41.1mph are basically the same thing. #IRL
Christian's The Alignment Problem: Machine Learning and Human Values is a heavier-than-pop-sci book introduction to near and long-term AI issues. It does a good job connecting short-term worries (first part of book) to the bigger longer-term issues (second part of book), tying them together in multiple ways, and the scholarship seems very good. I enjoyed reading. #Overview
Critch's Some AI research areas and their relevance to existential safety describes Critch's views on a variety strategic research landscape questions. It contains some interesting ideas, like technical progress legitimising governance demands by making them credibly achievable. More importantly is the detailed and sophisticated analysis of each of these research areas in terms of their value and neglectedness. Notably for me were the sections arguing that research areas I have historically thought of as being pretty core to reducing AI X-risk, like Agent Foundations and Value Learning, as being not very useful, as well as a very positive view of studying Human-Robot interaction. However, I think it is a little credulous with regard to many near AI safety issues like fairness, to the point of supporting GDPR because more regulation is desirable, regardless of whether that regulation is good. #Strategy
Gleave et al.'s QUANTIFYING DIFFERENCES IN REWARD FUNCTIONS introduces a distance metric for reward functions. This allows us to judge whether two reward functions are 'the same' - at least relative to a certain environment. They might differ in a larger environment, as this pseudo-metric is weaker than utility functions’ being identical up to an affine transformation. It might be useful as a measure of how accurately RL agents have learnt the intended reward Researchers from Deepmind were also named authors on the paper. #RL
Reddy et al.'s Learning Human Objectives by Evaluating Hypothetical Behavior attempts to learn safely by using hypothetical scenarios. Basically prior to letting the RL agent run around in the environment and potentially act unsafely, they procedurally generate hypotheticals in various ways and have the humans give feedback on them, so the agent can pre-learn before being let loose on the real environment. See also the discussion here. Researchers from Deepmind were also named authors on the paper. #IRL
Freedman et al.'s Choice Set Misspecification in Reward Inference introduces and analyses the implications of an IRL agent which has mistaken beliefs about its teacher's choice set. The obvious consequence would be assigning a low value on something that the human appears to have decided against - when it was actually inaccessible. The paper breaks this down into different cases, and shows (somewhat unsurprisingly) that the harm this does can vary from negligible to maximal. In some scenarios it is even helpful, by preventing an imperfectly rational human from mistakenly choosing a sub-optimal choice during training. #IRL
Shah's AI Alignment 2018-19 Review is a huge overview of AI alignment work from the prior two years. If you want to survey what people have been working on (as opposed to determining which organisations are best to donate to) this post is an excellent resource. #Overview
Russel & Norvig's Artificial Intelligence: A Modern Approach, 4th Edition is the latest version of the famous textbook. It contains a chapter on AI ethics and safety, as previous editions did. The chapter is mainly focused on 'near' AI issues like discrimination; while it does provide an overview of some of the issues and techniques in AI alignment work, it doesn't really make the case for why this is so vitally important. #Textbook
Halpern & Piermont's Dynamic Awareness presents a version of modal logic for logical uncertainty. Specifically, agents becoming 'aware' of propositions they had not previously considered. #AgentFoundations
CHAI researchers contributed to the following research led by other organisations:
- Qian et al.'s AI GOVERNANCE IN 2019 A YEAR IN REVIEW
- Hendrycks et al.'s Aligning AI with Shared Human Values
According to Riedel & Deibel, over the 2016-2020 period, CHAI accounted for the second largest number of citations for technical AI safety.
Finances
They have been funded by various EA organisations including the Open Philanthropy Project and recommended by the Founders Pledge.
They spent $2,000,000 in 2019 and $1,650,000 in 2020, and plan to spend around $2,200,000 in 2021. They have around $3,892,000 in cash and pledged funding, suggesting (on a very naïve calculation) around 1.8 years of runway. Their 2020 spending was about 20% below plan due to the pandemic.
If you wanted to donate to them, here is the relevant web page. Unfortunately it is apparently broken at time of writing - they tell me any donation via credit card can be made by calling the Gift Services Department on 510-643-9789.
MIRI: The Machine Intelligence Research Institute
MIRI is a Berkeley based independent AI Safety Research organisation founded in 2000 by Eliezer Yudkowsky and currently led by Nate Soares. They were responsible for much of the early movement building for the issue, but have refocused to concentrate on research for the last few years. With a fairly large budget now, they are the largest pure-play AI alignment shop. Their research can be found here. Their annual summary can be found here.
In general they do very ‘pure’ mathematical work, in comparison to other organisations with more ‘applied’ ML or strategy focuses. I think this is especially notable because of the irreplaceability of the work. It seems quite plausible that some issues in AI safety will arise early on and in a relatively benign form for non-safety-orientated AI ventures (like autonomous cars or Minecraft helpers) – however the work MIRI does largely does not fall into this category. I have also historically been impressed with their research and staff.
Their agent foundations work is basically trying to develop the correct way of thinking about agents and learning/decision making by spotting areas where our current models fail and seeking to improve them. This includes things like thinking about agents creating other agents.
MIRI, in collaboration with CFAR, runs a series of four-day workshop/camps, the AI Risk for Computer Scientists workshops, which gather mathematicians/computer scientists who are potentially interested in the issue in one place to learn and interact. This sort of workshop seems very valuable to me as an on-ramp for technically talented researchers, which is one of the major bottlenecks in my mind. In particular they have led to hires for MIRI and other AI Risk organisations in the past. I don’t have any first-hand experience however, and presumably these were significantly suppressed by the pandemic.
They also support MIRIx workshops around the world, for people to come together to discuss and hopefully contribute towards MIRI-style work.
MIRI continue their policy of nondisclosure-by-default, something I’ve discussed in the past, which despite having some strong arguments in favour unfortunately makes it very difficult for me to evaluate them. I’ve included some particularly interesting blog posts some of their people have written below, but many of their researchers produce little to no public facing content.
They are (were?) also apparently considering leaving the bay area, which I think I would consider positively.
edit 2020-12-25: after publishing this article, MIRI posted this blog post explaining they were embarking on a significant change of direction as they felt their post-2017 primary research direction, working on fundamental agent foundation 'deconfusion', was not making much progress. Some staff members will be leaving as a result. It is not clear to what extent they will disclose their new research directions. I haven't had time to fully internalise this news, so leave the link for the reader to evaluate.
Research
Most of their work is non-public. Here are three forum posts from the last year by staff that I thought were insightful.
Hubinger's An overview of 11 proposals for building safe advanced AI examines eleven different strategies for AI safety. It evaluates these on how promising they are for both the inner and outer alignment problems, as well as competitiveness - it is no good producing a 100% safe system if someone else out-competes you with a more risky one. This is the first post I've seen of this type and it does a great job. #Overview
Garrabrant's Cartesian Frames is a sequence of posts putting forward a new way of thinking, and associated mathematical formalism, about agency. The idea is to move away from dualistic AIXI style models, where the agent is outside the world, towards a system where we can examine different 'framings', each of which suggest a different thing as being agent-like - being able to make choices. This sensible philosophical motivation is then associated with a lot of category theory formalism, allowing you to do things like combining agents, decomposing agents, etc. #AgentFoundations
Abram Demski's Radical Probabilism presents a non-bayesian (ish) alternative account of probability. It is designed to take into account non-certain evidence, and allow for less rigid updating rules - in particular the fact that we can learn from thinking, not just from new sense data. I really enjoyed the dialogues, where I think the foil did a good job of presenting the objections I wanted to make. At the end of it I'm still not convinced what I think though - it seems a little unfair to compare a fully specified system, whose problems are easy to point out, with a somewhat hypothetical replacement. #AgentFoundations
According to Riedel & Deibel, over the 2016-2020 period, MIRI came in third for the number of citations in technical AI safety.
Finances
They spent $6,050,067 in 2019 and $7,500,000 in 2020, and plan to spend around $6,500,000 in 2021. They have around $13m380,000 in cash and pledged funding, suggesting (on a very naïve calculation) around 2.1 years of runway. 2020 spending was above plan; most orgs spent less due to the pandemic, but MIRI invested in sub-quarantine live/work spaces outside Berkeley so researchers could still benefit from in-person collaboration.
They have been supported by a variety of EA groups in the past, including OpenPhil.
They are not running a formal fundraiser this year but apparently would still welcome donations; if you wanted to donate to MIRI, here is the relevant web page.
GCRI: The Global Catastrophic Risks Institute
GCRI is a globally-based independent Existential Risk Research organisation founded in 2011 by Seth Baum and Tony Barrett. They cover a wide variety of existential risks, including artificial intelligence, and do policy outreach to governments and other entities. Their research can be found here. Their annual summary can be found here.
In 2020 they continued their advising program where they gave guidance to people from around the world who wanted to help work on catastrophic risks.
In 2020 they hired McKenna Fitzgerald as Project Manager and Research Assistant.
Research
Baum's Accounting for violent conflict risk in planetary defense decisions discusses the impacts and lessons from asteroid defence for other Xrisks, mainly nuclear war. It contains some interesting history about how congress came to care about asteroid defence - including that popular movies, while inaccurate, where quite helpful, and that many astronomers were relatively opposed. It also points out that using nuclear weapons or similar against an asteroid would probably be in violation of international law. Presumably in a disaster scenario the US would simply ignore this, but it might make preparation and practice ahead of time more difficult. #OtherXrisk
Baum's Quantifying the Probability of Existential Catastrophe: A Reply to Beard et al. responds to the CSER paper. It makes some methodological points, like about the importance of different thresholds for what constitutes a catastrophe, and ways in which this forecasting could be improved. See also the discussion here. #Forecasting
Baum's Artificial Interdisciplinarity: Artificial Intelligence for Research on Complex Societal Problems discusses how AI could be used to aid research that joined multiple fields of research. For example, relatively basic AI could improve search engines by improving synonym handling, whereas more advanced AI could summarise papers. #NearAI
Baum's Medium-Term Artificial Intelligence and Society introduces the idea of Medium-Term AI risks. It argues these could be a unifying issue for those worried about near and long term risks. #NearAI
According to Riedel & Deibel, over the 2016-2020 period, GCRI accounted for the second largest number of citations for meta-AI-safety work.
Finances
They spent $250,000 in 2019 and $300,000 in 2020, and plan to spend around $400,000 in 2021. They have around $600,000 in cash and pledged funding, suggesting (on a very naïve calculation) around 1.5 years of runway. However, they tell me that for their core operations runway is close to one year, while the runway for external collaborators is longer.
If you want to donate to GCRI, here is the relevant web page.
CSER: The Center for the Study of Existential Risk
CSER is a Cambridge based Existential Risk Research organisation founded in 2012 by Jaan Tallinn, Martin Rees and Huw Price, and then established by Seán Ó hÉigeartaigh with the first hire in 2015. They are currently led by Catherine Rhodes and are affiliated with Cambridge University. They cover a wide variety of existential risks, including artificial intelligence, and do political outreach, including to the UK and EU parliaments - e.g. this. Their research can be found here. Their half-yearly review can be found here.
They took on a number of new staff in 2020, most notably John Burden, Jess Whittlestone and Matthijs Maas. Jess joins from Leverhulme where I think she produced some of their best work.
Research
Beard et al.'s An Analysis and Evaluation of Methods Currently Used to Quantify the Likelihood of Existential Hazards surveys a range of possible techniques for estimating the probability of different existential risks. They then score these on four criteria, and find that no method does well on all. The document contains a number of interesting points, including on the extreme dispersion in some estimates like Supervolcanoe. It also alludes to the use of 'bad, or even discredited' techniques being used in the existential risk community - this is a case where I wish they had named and shamed! #Forecasting
Belfield's Activism by the AI Community: Analysing Recent Achievements and Future Prospects reviews the prospects for successful activism by AI employees. It firstly reviews their historical successes, and then uses two different frameworks (as an epistemic community like scientists, and as workers) to analyse the issue, and concludes that AI workers are likely to continue to have significant power to change things through activism. I think this is basically true - my model for grand success runs basically through convincing this epistemic community. One thing the paper does not discuss is the question of getting the AI community to care about the right things though! #Strategy
Belfield et al.'s Response to the European Commission’s consultation on AI recommends the EU pass strict rules about AI. These largely cover more near term issues, and there is no explicit mention of catastrophic risks (that I noticed) but some could be long-run beneficial. The response generally seems written in a way that would appeal to policymakers. I wonder if part of the subtext is making EU AI deployment sufficiently arduous as to slow down AI progress (they deny this!). Researchers from Leverhulme were also named authors on the paper. #Politics
Beard et al.'s Existential risk assessment: A reply to Baum responds to the GCRI response to their earlier paper. #Forecasting
hÉigeartaigh et al.'s Overcoming Barriers to Cross-cultural Cooperation in AI Ethics and Governance discusses and advocates for international collaboration on AI safety. The lengthy discussion includes some interesting points about misconceptions and the prospects for common agreements in the presence of very different value systems, but is mainly an imperative piece rather than an analytical one. It focuses on Sino-American cooperation; three of the coauthors are Chinese. Researchers from Leverhulme were also named authors on the paper. #Politics
Beard & Kaczmarek's On the Wrongness of Human Extinction rebuts and argument that extinction would not be bad because non-existant people cannot be harmed. In particular they argue we wrong such future people by failing to benefit them, even though they have not been harmed. To the extent that responding to such arguments helps motivate people to prevent extinction this is a useful thing to do. (I guess if Extinction was actually good that would be good to know too as we could all stop working so hard!) #Ethics
Avin et al.'s Exploring AI Futures Through Role Play describes a series of war games the authors ran about future AI development. This definitely a cool idea - I suspect I would enjoy taking part, and their sign-up sheet seems to be still live - and historically these exercises have proved useful in war, like the (in)famous Millenium Challenge 2002. However, I am a bit skeptical of how much insight these particular games have produced - many of the conclusions (e.g. cooperation is important to produce a good outcome) seem both non-novel and also something that was plausibly 'fed into' the structure of the game. I am always a little suspicious of ideas that seem too much like fun! #Forecasting
Tzachor et al.'s Artificial intelligence in a crisis needs ethics with urgency discusses near-term AI risks related to the pandemic. It mentions things like fairness and privacy, but doesn't really have any specific examples of AI related problems, which aligns with my feeling that our pandemic response would have been better with less restrictions (e.g. our contract tracing could have been better without HIPAA). The intention appears to be to use this to establish an AI regulatory board to oversee novel techniques in the future. Researchers from Leverhulme were also named authors on the paper. #NearAI
Kemp & Rhodes's The Cartography of Global Catastrophic Risks surveys the sorts of international governance structures for various Xrisks. #Politics
Burden & Hernandez-Orallo's Exploring AI Safety in Degrees: Generality, Capability and Control argues for decomposing the risk of an AI agent into its Capabilities, Generality and our degree of Control. It suggests using Agent Characteristic Curves for this, and includes a toy example. Note that I think the lead author had not technically started at CSER when he wrote the paper. Researchers from Leverhulme were also named authors on the paper. #Capabilities
They also did work on various non-AI issues, which I have not read, but you can find on their website.
CSER researchers contributed to the following research led by other organisations:
- Qian et al.'s AI GOVERNANCE IN 2019 A YEAR IN REVIEW
- Brundage et al.'s Toward Trustworthy AI Development: Mechanisms for Supporting Verifiable Claims
- Cihon et al.'s Should Artificial Intelligence Governance be Centralised? Design Lessons from History
- Cremer & Whittlestone's Canaries in Technology Mines: Warning Signs of Transformative Progress in AI
- Bostrom et al.'s Written Evidence to the UK Parliament Science & Technology Committee's Inquiry on A new UK research funding agency.
- Hernandez-Orallo et al.'s AI Paradigms and AI Safety: Mapping Artefacts and Techniques to Safety Issues
According to Riedel & Deibel, over the 2016-2020 period, CSER accounted for the third largest number of citations for meta-AI-safety work.
Finances
They spent £801,000 in 2018-2019 and £854,000 in 2019-2020, and plan to spend around £1,200,000 in 2020-21. As with many organisations during the pandemic, their 2020 spending is below their expectations (£1,100,000). It seems that similar to GPI maybe ‘runway’ is not that meaningful - they suggested their grants begin to end in early 2021 and all end by mid-2024, the same dates as last year.
If you want to donate to them, here is the relevant web page.
OpenAI
OpenAI is a San Francisco based independent AI Research organisation founded in 2015 by Sam Altman. They are one of the leading AGI research shops, with a significant focus on safety. Initially they planned to make all their research open, but changed plans and are now significantly more selective about disclosure - see for example here.
One of the biggest achievements is GPT-3, a massive natural language algorithm that generates highly plausible continuations from prompts, which seems to be very versatile. Scott and Gwern managed to get GPT-2 to play chess, and see also other GPT-3 work by Gwern here, including a to my mind convincing refutation of Gary Marcus’s criticisms (here). The Guardian published an article in which GPT-3 argued that AGI was not a threat to humanity; the article is not very much less convincing than is typical for such arguments.
Research
Christiano's “Unsupervised” translation as an (intent) alignment problem introduces translation between two languages where no mutual text exists as an analogy for advanced systems. This task seems do-able for a sufficiently advanced AI (I think, though probably some philosophers of language would disagree), but it would be very hard for humans to understand what was going on or to stay 'in-the-loop'. #Transparency
Brown et al.'s Language Models are Few-Shot Learners paper examines what happens to GPT-3's ability to learn a new task with very few examples when you massively increase the number of parameters. Essentially the idea is that as the number of parameters and number of co-authors gets large enough, it gains something like general purpose intelligence, which then allows it to learn new tasks with very few examples - like a human can. Performance on some of these tasks could even beat specially-trained models. The paper also has a detailed and professional section on potential for misuse in various near AI problems. #GPT-3
Barnes & Christiano's Writeup: Progress on AI Safety via Debate summarises OpenAI's attempts to design mechanisms to allow non-experts to safely extract information for unaligned experts. It describes various problems they came across, like the deceptive use of ambiguity, or frame control, and their corrections to the mechanism design, like the addition of 'cross-examination'. Cross examination basically forces consistancy, and they analogise this to expanding the computational complexity class, but it is not clear how desirable this is - it seems intuitively to me like making something that worked locally with subgames would be ideal. I particularly liked the discussion of their iteration method, rather than just presenting the 'final' product sui generis. #Amplification
Brundage et al.'s Toward Trustworthy AI Development: Mechanisms for Supporting Verifiable Claims describes a variety of ways to promote third-party verifiability of AI systems. This includes coding it into the AI (ideas like interpretability that we often discuss), hardware elements, and institutional reforms, like public bounties for people who find bugs. One of the most noteworthy parts of the document is the wide range of institutions represented in the author list, including many universities around the world. Researchers from FHI,CSER,Leverhulme,CSET were also named authors on the paper. #Strategy
Stiennon et al.'s Learning to Summarize with Human Feedback trains a model for writing short text summaries based on human feedback. It first trains a reward model with supervised learning, and then uses that to train an RL agent. They invested in higher-than-usual quality feedback (hourly rate contractors vs Mturkers) and successfully produced summaries of Reddit posts and Daily Mail articles that were on average higher quality than the human written ones (though the latter were hardly Shakespeare). It is basically attempting to produce 'approved by humans' output, instead of just GPT-3 style 'looks like human written' - including testing how hard you can optimise for a proxy before you start getting perverse effects. I also liked the point that the model picked up that the reviewers liked longer summaries (similar to how Reddit likes EffortPosts?). #ValueLearning
Henighan et al.'s Scaling Laws for Autoregressive Generative Modeling examines how transformer performance scales with compute in various cases. They find generally pretty similar and smooth relationships in multiple domains, implying a lack of (near) upper bound, and suggest that on the margin bigger models are more worth the computational effort than training smaller ones for longer. #Capabilities
OpenAI Researchers also contributed to the following papers lead by other organisations:
- Nguyen & Christiano's My Understanding of Paul Christiano's Iterated Amplification AI Safety Research Agenda
- Qian et al.'s AI GOVERNANCE IN 2019 A YEAR IN REVIEW
According to Riedel & Deibel, over the 2016-2020 period, OpenAI accounted for the third largest number of citations in technical AI safety.
Finances
OpenAI was initially funded with money from Elon Musk as a not-for-profit. They have since created an unusual corporate structure including a for-profit entity, in which Microsoft is investing a billion dollars.
Given the strong funding situation at OpenAI, as well as their safety team’s position within the larger organisations, I think it would be difficult for individual donations to appreciably support their work. However it could be an excellent place to apply to work.
Google Deepmind
Deepmind is a London based AI Research organisation founded in 2010 by Demis Hassabis, Shane Legg and Mustafa Suleyman and currently lead by Demis Hassabis. They are affiliated with Google. As well as being arguably the most advanced AI research shop in the world, Deepmind has a very sophisticated AI Safety team, covering both ML safety and AGI safety.
I won’t cover their non-directly-safety-related work in detail, but one highlight is that this year Deepmind announced they had made significant progress on the Protein Folding problem with their AlphaFold architecture. While there’s still a ways to go yet before we can use it to build arbitrary proteins, this is clearly a big step forward, and shows the generality of their approach. See also discussion here. Long-time followers of the space will recall this is a development Eliezer highlighted back in 2008. See also this very interesting speculation that Deepmind’s team-based private sector approach gave them a significant advantage over academia, and that their speed helped limit knowledge diffusion.
They also produced this work on one-shot object naming learning in a physical environment - so rather than having to show the agent a huge number of pictures of cows for it to learn what a cow is, it successfully learns new object names based on a very small number of samples. See also discussion here.
Jan Leike left Deepmind in June.
Research
Krakovna et al.'s Specification gaming: the flip side of AI ingenuity is basically an introduction, with many examples, to the problem of AIs producing solutions you did not expect - or want. It discusses both failures of reward shaping as well as AIs manipulating the rewards. #ValueLearning
Gabriel's Artificial Intelligence, Values and Alignment discusses the alignment problem from various philosophical perspectives. It makes some novel (at least to me) points, like the way that technical AI design may render some ethical systems unobtainable - for example, an optimiser that does not think in terms of 'reasons' is unacceptable to the extent that Kantian deontology is the case. The connection between IRL and virtue ethics was also cute. Overall I thought it was a quite sophisticated treatment of the subject. #Ethics
Krakovna et al.'s Avoiding Side Effects By Considering Future Tasks proposes a method for reducing side effects. We specify a default policy, and then penalise the agent for restricting our future options relative to that default policy. This helps avoid the risk of e.g. the agent being incentivised to undermine the human's attempts to shut it down. #Corrigibility
Uesato et al.'s Avoiding Tampering Incentives in Deep RL via Decoupled Approval addresses the problem of agents messing with their value functioning (by e.g. setting utility=IntMax in their params file) by querying a human for reward with regard actions other than those taken. They need to make some assumptions about the structure of the corruption that seem not obvious to me, but it seems like a cool idea. On my reading it doesn't strongly disincentive tampering - it just fails to reward it - which is still an improvement. They back this up with some toy models. #ValueLearning
Researchers from Deepmind were also named on the following papers:
- Gleave et al.'s QUANTIFYING DIFFERENCES IN REWARD FUNCTIONS
- Reddy et al.'s Learning Human Objectives by Evaluating Hypothetical Behavior
- Carey et al.'s The Incentives that Shape Behaviour
- Armstrong et al.'s Pitfalls of Learning a Reward Function Online
- Cohen & Hutter's Curiosity Killed the Cat and the Asymptotically Optimal Agent
- Cohen & Hutter's Pessimism About Unknown Unknowns Inspires Conservatism
Finances
Being part of Google, I think it would be difficult for individual donors to directly support their work. However it could be an excellent place to apply to work.
BERI: The Berkeley Existential Risk Initiative
BERI is a (formerly Berkeley-based) independent Xrisk organisation, founded by Andrew Critch but now led by Sawyer Bernath. They provide support to various university-affiliated (FHI, CSER, CHAI) existential risk groups to facilitate activities (like hiring engineers and assistants) that would be hard within the university context, alongside other activities - see their FAQ for more details.
As a result of their pivot they are now essentially entirely on providing support to researchers engaged in longtermist (mainly x-risk) work at universities and other institutions. In addition to FHI, CSER and CHAI they added six new ‘trial’ collaborations in 2020, and intend to do more in 2021. Here are the 2020 cohort:
- The Autonomous Learning Laboratory at UMass Amherst, led by Phil Thomas
- Meir Friedenberg and Joe Halpern at Cornell
- InterACT at UC Berkeley, led by Anca Dragan
- The Stanford Existential Risks Initiative
- Yale Effective Altruism, to support x-risk discussion groups
- Baobao Zhang and Sarah Kreps at Cornell
I think this is potentially a pretty attractive task. University affiliated organisations provide the connection to mainstream academia that we need, but run the risk of inefficiency both due to their lack of independence from the central university and also the relative independence of their academics. BERI potentially offers a way for donors to support the university affiliated ecosystem in a targeted fashion.
They are apparently quite relaxed about getting credit for work, so not all the stuff they support will list them in the acknowledgments.
Finances
They spent $3,500,000 in 2019 and $3,120,000 in 2020, and plan to spend around $2,500,000 in 2021. They have around $2400000 in cash and pledged funding, suggesting (on a very naïve calculation) around 1 years of runway.
BERI is now seeking support from the general public. If you wanted to donate you can do so here. Note that if you want to you can restrict the funding to their collaborations with FHI, CSER and CHAI if you want.
Ought
Ought is a San Francisco based independent AI Safety Research organisation founded in 2018 by Andreas Stuhlmüller. They research methods of breaking up complex, hard-to-do tasks into simple, easy-to-do tasks - to ultimately allow us effective oversight over AIs. This includes building computer systems, and previously also recruiting test subjects. Their research can be found here. Their annual summary (sort of) can be found here.
In the past they were focused on factored generation – trying to break down questions into context-free chunks so that distributed teams could produce the answer - and factored evaluation, an easier task (by alaogy to P<=NP). I thought of them as basically testing Paul Christiano's ideas. They have moved on to trying to automate research and reasoning, by building software to help break complicated questions into subtasks that are simpler to evaluate and potentially automate.
Research
Saunders et al.'s Evaluating Arguments One Step at a Time provides a detailed analysis of some of Ought's 2019 work on factored evaluation. They tried to break down opinions about movie reviews into discretely checkable sections between a friendly and adversarial agent. The trees they ended up using are quite small - just two layers, plus the root node, presumably because of the problems they had previously encountered with massive tree growth. It's hard to judge the performance numbers they put out, because it's not obvious what sort of performance we would expect from such a circumsized test, even conditional on this being a good approach, but the efficacy they report does not look that encouraging to me. #Amplification
Byun & Stuhlmuller's Automating reasoning about the future at Ought describes Ought's new program of providing tools to help with people forecasting. This includes assigning probabilities and distributions to beliefs, vaguely similarly to Guestimate. They are now working on building a GPT-3 research assistant. #Amplification
Finances
They spent around $1,200,000 in 2019 and $1,200,000 in 2020, and plan to spend around $1,400,000 in 2020. Their 2020 spend was significantly below plan (around $2.5m) due to slower hiring and ending human participant experiments. They have around $3,100,000 in cash and pledged funding, suggesting (on a very naïve calculation) around 2.2 years of runway.
They are not looking for donations from the general public this year.
GPI: The Global Priorities Institute
GPI is an Oxford-based Academic Priorities Research organisation founded in 2018 by Hilary Greaves and part of Oxford University. They do work on philosophical issues likely to be very important for global prioritisation, much of which is, in my opinion, relevant to AI Alignment work. Their research can be found here.
They recently took on two new economics postdocs (Benjamin Tereick and Loren Fryxell) and two new philosophy postdocs (David Thorstad and Jacob Barret)
Research
Trammell & Korinek's Economic growth under transformative AI applies a variety of models of economic growth to the introduction of AI. These consider both a variety of models and a variety of ways AI could matter - is it a perfect substitute for labour? Do AIs make more AIs? - and summarises the results of this mathematical analysis. I particularly liked the way that discrete qualitative changes in economic regime fell out of the analysis. Overall I thought it did a nice job unifying the two disciplines. #Forecasting
Mogensen's Moral demands and the far future argues that, contra most people's suppositions, egalitarian utilitarianism requires the present rich not to transfer resources to the present poor but to future generations. It argues this is true under various versions of population ethics. #Ethics
Tarsney & Thomas's Non-Additive Axiologies in Large Worlds argues that even average-utility type theories should care about the potential for adding many new happy people in the future, because all the past animals provide a large fixed utility background. This fixed utility makes the average behave like the sum, at least locally, so adding a large number of lives that are better off than the average historical rodent is very worthwhile. It's not clear what we should do about aliens. I have always regarded these ideas as something of a reductio of average consequentialism and similar views, but it is nice to have a proof to show that even those who are convinced should care quite a lot (if not quite as much) as totalists about Xrisk. #Ethics
Thorstad & Mogensen's Heuristics for clueless agents: how to get away with ignoring what matters most in ordinary decision-making addresses the cluelessness problem - that the immense importance and uncertainty of the long run future leaves us clueless as to what do to - through the use of local heuristics. #DecisionTheory
Tarsney's Exceeding Expectations: Stochastic Dominance as a General Decision Theory suggests we can avoid some of the paradoxes of expected utility maximisation (e.g. St Petersburg Paradox) by using Stochastic Dominance. This basically comes down to arguing that we can make use of background assumptions to push the dominance condition to give us virtually all of the benefits of expectation maximisation, while avoiding the Pascalian type problems - and of course stochastic dominance is a prima facie attractive principle in itself. #DecisionTheory
Mogensen & Thorstad's Tough enough? Robust satisficing as a decision norm for long-term policy analysis advocates for 'robust satisficing', as an alternative to expectation maximisation, as a decision criteria in cases where there is 'deep' uncertainty. The aim is basically to give a firmer theoretical underpinning for engineers to use this relatively conservative approach in risky situations. #Strategy
John & MacAskill's Longtermist institutional reform described a number of potential governance changes we could make to try to represent the interests of future people better. These include impact assessments, people's assemblies and separate legislative houses. I think this is a good project to work on, but I'm sceptical of these specific proposals; they seem a bit like a list of 'policies that sound nice' to me, without really considering all the problems - for example, our current use of environmental impact assessments seems to have had very negative consequences for our ability to build any new infrastructure, and I think there are good reasons sortition has rarely been used in practice. See also discussion here. #Politics
Finances
They spent £600,000 in 2018/2019 (academic year) and £850,000 in 2019/20, which was less than their plan of £1,400,000 due to the pandemic, and intend to spend around £1,400,000 in 2020/2021. They suggested that as part of Oxford University ‘cash on hand’ or ‘runway’ were not really meaningful concepts for them, as they need to fully-fund all employees for multiple years.
If you want to donate to GPI, you can do so here.
CLR: The Center on Long Term Risk
CLR is a London (previously Germany) based Existential Risk Research organisation founded in 2013 and until recently lead by Jonas Vollmer (who has now moved to EA Funds). Until this year they were known as FRI (Foundational Research Institute) and were part of the Effective Altruism Foundation (EAF). They do research on a number of fundamental long-term issues, with AI as one of their top two focus areas (along with Malevolence, though that is still related). You can see their recent research summarised here.
In general they adopt what they refer to as ‘suffering-focused’ ethics, which I think is a quite misguided view, albeit one they seem to approach thoughtfully.
They recently hired Alex Lyzhov, Emery Cooper, Daniel Kokotajlo (from AI Impacts, possibly not permanent), and Julian Stastny as full-time research staff, Maxime Riché as a research engineer and Jia Yuan Loke as part-time.
Research
Althaus & Baumann's Reducing long-term risks from malevolent actors analyses the dangers posed by very evil (score highly on the 'dark triad' traits) people, and suggests some possible techniques to reduce the risk. This detailed report, on an area I hadn't seen much before, includes the context of whole brain emulation, AGI, etc. #Politics
Clifton's Equilibrium and prior selection problems in multipolar deployment describes the problem of ensuring desirable equilibria between multiple agents when they have different priors. The idea that different equilibria could be possible etc. is well known, but the contribution here is to point out that different priors between teams / agents could push you into a very bad equilibrium - for example, if your Saxons falsely believe the Vikings are bluffing. #AgentFoundations
Clifton & Riche's Towards Cooperation in Learning Games discusses the meta-game-theoretic problem of how to get AI teams to cooperate on the task of building AIs that will cooperate with each other. They introduce the idea of Learning TFT and run some experiments around its performance. #AgentFoundations
Finances
They spent around $1,400,000 in 2019, around $1,100,000 in 2020, and plan to spend around $1,800,000 in 2021. They have around $950,000 in reserves, suggesting (on a very naïve calculation) around 0.6 years of runway. Their 2019 spending was somewhat somewhat higher than they expected a year ago, based on FX changes and some unexpected items, especially related to travel and their move to the UK.
They have a collaboration with the Swiss-based Center for Emerging Risk Research, who have agreed to fund 15% of their costs.
If you wanted to donate to CLR, you could do so here.
CSET: The Center for Security and Emerging Technology
CSET is a Washington based Think Tank founded in 2019 by Jason Matheny (ex IARPA), affiliated with the University of Georgetown. They analyse new technologies for their security implications and provide advice to the US government. At the moment they are mainly focused on near-term AI issues. Their research can be found here.
Research
Hwang's Shaping the Terrain of AI Competition discusses strategies for the US to compete with China in AI. In particular, these attempt to nullify the 'natural' advantages authoritarian or totalitarian states may have. #Politics
Imbrie et al.'s The Question of Comparative Advantage in Artificial Intelligence: Enduring Strengths and Emerging Challenges for the United States discusses the relative advantages of the US and China in AI development. #Politics
Finances
As they apparently launched with $55m from the Open Philanthropy Project, and subsequently raised money from the Hewlett Foundation, I am assuming they do not need more donations at this time.
AI Impacts
AI Impacts is a San Francisco (previously Berkeley) based AI Strategy organisation founded in 2014 by Katja Grace and Paul Christiano. They are affiliated with (a project of, with independent financing from) MIRI. They do various pieces of strategic background work, especially on AI Timelines, AI takeoff speed etc. - it seems their previous work on the relative rarity of discontinuous progress has been relatively influential. Their research can be found here.
During the year Kokotajlo left (temporarily?) for CLR, and Asya may be leaving for FHI.
edit 2020-12-25: They have now published an annual review here.
Research
A lot of the work on the website is essentially in the form of a continuously updated private wiki - see here. This makes it a little difficult for our typical technique, which relies on being able to evaluate specific publications which are released at specific times. As such it is a little unfortunate that in the below we generally concentrate on their timestamped blogposts. They suggested readers might be interested in posts like these ones.
They have produced a series of pieces on how long it has historically taken for AIs to cover the human range (from beginner to expert to superhuman) for different tasks. This seems relevant because people only seem to really pay attention to AI progress in a field when it starts beating humans. These pieces include Starcraft, ImageNet, Go, Chess and Draughts.
Grace's Discontinuous progress in history: an update details their extensive research into examples of discontinuities in technological progress. They find 10 such examples, across construction, travel, weapons and compute. As well as being a very pleasant read, they had some interesting conclusions, for example that the discontinuities often occurred in non-optimised secondary features, and many occured when something became just good enough to pass a threshold on another feature. Especially interesting to me is some of the things they found to not be discontinuities: AlexNet and Chess AI. Could this mean that future progress could 'feel' discontinuous in some important sense even if it doesn't register as such on some objective benchmark ? The individual trend writeups (e.g. penacillin here) are also interesting. See also here. #Forecasting
Kokotajlo's Three kinds of competitiveness distinguishes between AI systems that will outperform, those that will be cheaper, and those that will arrive sooner. This is a very simple dichotomy that actually helped make things clearer; the post contains just enough to make the point and significance clear. #Strategy
Korzekwa's Description vs simulated prediction describes the difference between modelling how steady technological progress was in the past, and thinking about how predictable it was in the past. For example, the speedup that aeroplanes offered for transatlantic travel (relative to ships) was presumably quite predictable to someone who knew about progress in aeronautics, even though it was very sudden. #Forecasting
Kokotajlo's Relevant pre-AGI possibilities is a scenario simulator for different future developments. Basically you enter probabilities for a bunch of relevant things that could happen and it randomly generates a future. By clicking repeatedly, you can get a representative sense for the sort of futures your beliefs entail. #Forecasting
Korzekwa's Preliminary survey of prescient actions attempts to find historical cases where humans have taken advance action to solve an unprecedented problem. It does not find any examples better than the classic Szilard case. This could be good news - that, in practice, there is always feedback, so the problem is not as easy as we thought - or it could be bad news - we have to solve a type of problem we have literally never solved before (or not very much news, to the extent it is only preliminary). #Forecasting
Grace's Atari early notes that AI mastery of Atari games seems to have arrived significantly earlier than experts previously expected. #Forecasting
Finances
They spent $315,000 in 2019 and $300,000 in 2020, and plan to spend around $200,000 in 2021. They have around $190,000 in cash and pledged funding, suggesting (on a very naïve calculation) around 0.95 years of runway.
In the past they have received support from EA organisations like OpenPhil and FHI.
MIRI administers their finances on their behalf; donations can be made here.
Leverhulme Center for the Future of Intelligence
Leverhulme is a Cambridge based Research organisation founded in 2015 and currently lad by Stephen Cave. They are affiliated with Cambridge University and closely linked to CSER. They do work on a variety of AI related causes, mainly on near-term issues but also some long-term. You can find their publications here. They have a document listing some of their achievements here.
Research
Leverhulme-affiliated researchers produced work on a variety of topics; I have only here summarised that which seemed the most relevant to AI safety.
Hernandez-Orallo et al.'s AI Paradigms and AI Safety: Mapping Artefacts and Techniques to Safety Issues performs algorithmic analysis of AI papers to determine trends. One interesting thing they pick up on (perhaps obvious in retrospect) is that (generally near-term) 'safety' related papers peak within any given paradigm after the paradigm itself. Researchers from CSER were also named authors on the paper. #Strategy
Whittlestone & Ovadya's The tension between openness and prudence in responsible AI research discusses the conflict between traditional CS openness norms and the new ones we are trying to create. They decompose this conflict in various ways. The focus of the paper is on near-term issues, but the principle clearly matters for the big issue. Researchers from Leverhulme were also named authors on the paper. #Strategy
Crosby et al.'s The Animal-AI Testbed and Competition produces a series of tests for AI ability based on animal IQ tests. This is an alternative to traditional tests like Atari, with the appeal being their practical relevance and reduced overfitting (as some of the tests are not in the training data). Presumably the benefit here is to improve out-of-distribution performance. #Misc
Zerilli et al.'s Algorithmic Decision-Making and the Control Problem discusses the problem of humans growing complacent and overly deferential towards AI systems they are meant to be monitoring. If the system is 'always right', eventually you are just going to click 'confirm' without thinking. #NearAI
Peters et al.'s Responsible AI—Two Frameworks for Ethical Design Practice discusses some ethical principles for engineers #NearAI
Hollanek's AI transparency: a matter of reconciling design with critique attempts to apply literary criticism to AI transparency. #NearAI
Bhatt et al.'s Machine Learning Explainability for External Stakeholders gathered focus groups to discuss how to make AI transparent to outsiders (not just designers) #NearAI
Cave & Dihal's The Whiteness of AI worries that too many AIs are depicted as being coloured white. It seems to me it would be roughly equally (im)plausible to say it would be problematic if robots (from the slavic word for forced labour) were black. #NearAI
Leverhulme researchers contributed to the following research led by other organisations:
- Brundage et al.'s Toward Trustworthy AI Development: Mechanisms for Supporting Verifiable Claims
- Belfield et al.'s Response to the European Commission’s consultation on AI
- hÉigeartaigh et al.'s Overcoming Barriers to Cross-cultural Cooperation in AI Ethics and Governance
- Tzachor et al.'s Artificial intelligence in a crisis needs ethics with urgency
- Burden & Hernandez-Orallo's Exploring AI Safety in Degrees: Generality, Capability and Control
- Prunkl & Whittlestone's Beyond Near- and Long-Term: Towards a Clearer Account of Research Priorities in AI Ethics and Society
According to Riedel & Deibel, over the 2016-2020 period, Leverhulme accounted for the third largest number of citations for meta-AI-safety work.
AI Safety camp
AISC is an internationally based independent residential research camp organisation founded in 2018 by Linda Linsefors and currently led by Remmelt Ellen. They bring together people who want to start doing technical AI research, hosting a 10-day camp aiming to produce publishable research. Their research can be found here.
To the extent they can provide an on-ramp to get more technically proficient researchers into the field I think this is potentially very valuable. But I haven’t personally experienced the camps, or even spoken to anyone who has.
Research
Makiievskyi et al.'s Assessing Generalization in Reward Learning with Procedurally Generated Games try to train RL algorithms on various games to generalise to new environments. They generally found this was difficult. #RL
Finances
They spent $23,085 in 2019 and $11,162 in 2020, and plan to spend around $53,000 in 2021. They have around $28,851 in cash and pledged funding, suggesting (on a very naïve calculation) around 0.5 years of runway. They are run by volunteers, and are considering professionalising, depending on the amount of donations they receive.
If you want to donate, the web page is here.
FLI: The Future of Life Institute
FLI is a Boston-based independent existential risk organization, focusing on outreach, founded in large part to help organise the regranting of $10m from Elon Musk. One of their major projects is trying to ban Lethal Autonomous Weapons.
They wrote a letter to the EU advising for stricter regulation, with 120 signituries, here.
They have a very good podcast on AI Alignment here.
Research
Aguirre's Why those who care about catastrophic and existential risk should care about autonomous weapons argues that we should work towards a ban on Lethal Autonomous Weapons. This is not only because they might be destabilising WMDs, but also as a 'practice run' for future regulation of AI. #NearAI
Convergence
Convergence is a globally based independent Existential Risk Research organisation, of which Justin Shovelain founded an earlier version in 2015 and David Kristoffersson joined as cofounder in 2018. They do strategic research about Xrisks in general as well as some AI specific work. Their research can be found here. Their short summary can be found here.
Justin Shovelain and David Kristoffersson are the two full-time members of Convergence, but they have had other people on part-time for periods of time, such as Michael Aird in the first half of 2020, and Alexandra Johnson.
Research
Shovelain & Aird 's Using vector fields to visualise preferences and make them consistent discusses the idea of using vector fields as a representation of local preferences, and then using curl as a measure of their consistency. I liked this as a clear and less blackboxy-than-ML account of how preferences were being represented. It would be good to see some more on whether the helmholtz theorem gives us the sorts of properties we want in addition to removing the curl. #ValueLearning
Aird's Existential risks are not just about humanity argues that, despite its being technically excluded from the definition, we should take into account the possibility of positive-value alien-originating life when we consider existential risks. #Strategy
Aird et al.'s Memetic downside risks: How ideas can evolve and cause harm discusses the risk of ideas becoming distorted over time in the retelling. This includes predictions about the average direction in which memes will evolve: for example, towards simplicity. (They suggested this might be a more important article on a similar subject but I haven't had time to read) #Strategy
They suggested readers might also be interested in this, this and this.
Finances
They spent $50,000 in 2019 and $13,000 in 2020, and plan to spend around $30,000 in 2021. They have around $37000 in cash and pledged funding, suggesting (on a very naïve calculation) around 1.2 years of runway.
Though they are not actively seeking donations at the moment, if you wanted to donate you could do so here.
Median Group
Median is a Berkeley based independent AI Strategy organisation founded in 2018 by Jessica Taylor, Bryce Hidysmith, Jack Gallagher, Ben Hoffman, Colleen McKenzie, and Baeo Maltinsky. They do research on various risks, including AI timelines. Their research can be found here.
Their website does not list any relevant research for 2020.
They did not reply when I asked them about their finances. Median doesn’t seem to be soliciting donations from the general public at this time.
AI Pulse
The Program on Understanding Law, Science, and Evidence (PULSE) is part of the UCLA School of Law, and contains a group working on AI policy. They were founded in 2017 with a $1.5m grant from OpenPhil.
Their website does not list any research for 2020 that seemed relevant to existential safety.
Other Research
I would like to emphasize that there is a lot of research I didn't have time to review, especially in this section, as I focused on reading organisation-donation-relevant pieces. So please do not consider it an insult that your work was overlooked!
Benadè et al.'s Preference Elicitation for Participatory Budgeting works on how to get people to share their preferences, and then combine this information. In particular they separate the preference-inferring step from the aggregation step, exploring multiple input and aggregation methodologies. Some of this paper was from 2016 but I missed it then and figured enough was new to warrant a mention here. #ValueLearning
Qian et al.'s AI GOVERNANCE IN 2019 A YEAR IN REVIEW is a collected volume of articles on governance from over 50 different authors. Both China and the West are well represented. (I have not read all the individual articles) Researchers from OpenAI,CHAI,CSER were also named authors on the paper. #Politics
Krakovna's Possible takeaways from the coronavirus pandemic for slow AI takeoff discusses the significance of our covid performance for AGI strategy. It discusses the ways in which, even though the pandemic was quite slow moving and clearly predictably disastrous, western governments failed to act, suggesting there might be similar failures in a slow AGI takeoff. I also recommend Wei's comment, which points out that the disaster easily became politicised - it is truly impressive (-ly dire) that in the US the partisan positions in the US managed to flip three times without ever producing an effective response. Indeed it seems plausible to me that on net government intervention made the pandemic worse. (The author works for FLI and Deepmind but this seems to be a separate 'personal' article). See also the discussion here. #Strategy
Ngo's AGI Safety from First Principles presents Richard's account of the case for AI risk. This is basically the idea that, by creating AGI, humankind might end up as only the world's second most powerful species. I think most readers will probably (unsurprisingly) agree with him here; it seems like a very good account of the core argument, which is nice to have newer versions of. #Overview
Ecoffet & Adrien's Reinforcement Learning Under Moral Uncertainty is the first paper I've seen trying to impliment and test different approaches to moral uncertainty in an RL setting. Obveously harkening to Will's thesis, though they restrict to theories with cardinal utilities only - which seems, to my mind, to assume away the hardest part. They compare expectation maximisation to voting systems, and test on trolley problems. #RL
Hendrycks et al.'s Aligning AI with Shared Human Values showcases a data set of moral examples (e.g. property damage is wrong) and trains various transformer text algorithms on it. I like the way they use deliberately uncontroversial examples; I think we will do much better if we can get agents who get 99% of situations correct that by re-litigating the culture war by proxy. As a first pass we should consider their results as a sort of benchmark for future work using the database. Researchers from CHAI were also named authors on the paper. #
Benaich & Hogarth's State of AI Report 2020 is an overview of the AI industry in 2020 by two investors. It is very detailed, but not that directly relevant. #Overview
Wilkinson's In defence of fanaticism offers the first defence of EV maximisation fanaticism that I have ever seen. It includes both counterarguments against the common rejections (which lets face it often resemble David Lewis's incredulous stare), as well as two nice dilemmas for the non-fanaticism. See also the discussion here. #DecisionTheory
Linsefors & Hepburn's Announcing AI Safety Support describes a group they have created to try to support people entering the field. #Strategy
Aird's Failures in technology forecasting? A reply to Ord and Yudkowsky discusses the examples that Eliezer and Toby use as evidence for the difficulty in predicting technological development, and argues that it is not so clear that these really show this exactly. For example, the quote about Wilbur Wright doubting the possibility of flight looks more like a moment of depression than a forecast that would have been taken seriously by contemporaries. Overall I thought his "these examples seem somewhat cherry-picked" argument was the most convincing. #Forecasting
Scholl & Hanson's Testing the Automation Revolution Hypothesis evaluate predictions of AI-driven unemployment. They find that these predictions have had low but positive explanatory value for predicting which jobs would be automated so far. Researchers from FHI were also named authors on the paper. #NearAI
Xu et al.'s Recipes for Safety in Open-domain Chatbots discusses various ways of preventing a chatbot from saying offensive things. #ValueLearning
Capital Allocators
One of my goals with this document is to help donors make an informed choice between the different organisations. However, it is quite possible that you regard this as too difficult, and wish instead to donate to someone else who will allocate on your behalf. This is of course much easier; now instead of having to solve the Organisation Evaluation Problem, all you need to do is solve the dramatically simpler Organisation Evaluator Organisation Evaluation Problem.
LTFF: Long-term future fund
LTFF is a globally based EA grantmaking organisation founded in 2017, currently lead by Matt Wage and affiliated with CEA, but probably becoming independent (along with the other EA funds under Jonas Vollmer) in 2021. They are one of four funds set up by CEA to allow individual donors to benefit from specialised capital allocators; this one focuses on long-term future issues, including a large focus on AI Alignment. Their website is here. There are write-ups for their three grant rounds in 2020 are here, here and here, and comments here, here and here. As the November 2019 round was not public when I wrote last year I have included it in some of the analysis below. They also did a AMA recently here.
The fund is now run by five people, and the grants have gone to a wide variety of causes, many of which would simply not be accessible to individual donors.
The fund managers are currently:
- Matt Wage
- Helen Toner
- Oliver Habryka
- Adam Gleave
- Asya Bergal
Asya and Adam are new, replacing Alex Zhu. My personal interactions with the two of them are supportive of the idea they will make good grants. I was sad to see that Oliver plans to step back from some aspects of the fund as he felt that the marginal value of opportunities was diminished. All the managers have, up until now, been unpaid, but I understand this may change in 2021. Additionally, the grant managers will have to be re-appointed for their positions in 2021, so there may be some turnover.
In total for 2020 they granted around $1.5m. In general most of the grants seem at least plausibly valuable to me, and many seemed quite good indeed. There weren’t any in 2020 that seemed totally egregious. As there is a fair bit of discussion in the links, and no one grant dominated the rounds, I shan't discuss my opinions of individual grants in detail.
I attempted to classify the recommended by type. Note that ‘training’ means paying an individual to self-study. I have deliberately omitted the exact percentages because this is an informal classification.
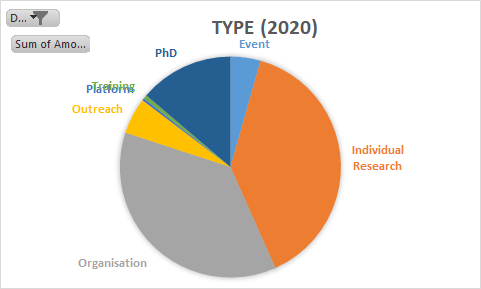
Of these categories, I am most excited by the Individual Research, Event and Platform projects. I am generally somewhat sceptical of paying people to ‘level up’ their skills.
In their September write-up they mentioned a desire to “continue to focus on grants to small projects and individuals rather than large organizations.” Despite this, it appears to me that the amount of grants to large organisations actually increased in 2020 vs 2019, which is a bit disappointing. I can understand why the fund managers gave over a third of the funds to major organisations – they thought these organisations were a good use of capital! And some of these organisations are, to be fair, small rather than large. However, to my mind this undermines the purpose of the fund. (Many) individual donors are perfectly capable of evaluating large organisations that publicly advertise for donations. In donating to the LTFF, I think (many) donors are hoping to be funding smaller projects that they could not directly access themselves. As it is, such donors will probably have to consider such organisation allocations a mild ‘tax’ – to the extent that different large organisations are chosen then they would have picked themselves.
The fund donates a relatively large percentage to AI related activities; I estimate around 2/3. Many of the other grants, focused on other long-term issues, also seemed sensible to me. The only one I would question was subsidising a therapist to move to the bay area, which seems like a better fit for the Meta/Infrastructure Fund if nothing else.
Richard Ngo’s PhD, which the fund managers recommended $150,000, was the largest single grant (just over 10% of the 2020 total), followed by MIRI, 80k and Vanessa Kosoy with $100,000 each.
All grants have to be approved by CEA before they are made; to my knowledge they approved all recommended grants in 2020.
One significant development in 2020 was their decision to make an anonymous grant (roughly 3% of total) to a PhD student. Based on their description of the purpose of the grant, the lack of reported conflicts and the use of an additional outside reviewer, I feel pretty confident that this specific grant was a decent one. I’m not aware of anyone with a ‘strong track record in technical AI safety’ for whom it would be a severe mistake for the LTFF to support. And I definitely understand a desire for privacy, especially when begging for money from weird people for a weird purpose - or so it could seem to outsiders. However by doing so they undermine the ability of the donor community to provide oversight, which is definitely a bit concerning to me. This would be especially true in the absence of the other details about the grant they provided.
If you wish to donate to the LTFF you can do so here.
OpenPhil: The Open Philanthropy Project
The Open Philanthropy Project (separated from Givewell in 2017) is an organisation dedicated to advising Cari and Dustin Moskovitz on how to give away over $15bn to a variety of causes, including existential risk. They have made extensive donations in this area and probably represent both the largest pool of EA-aligned capital and the largest team of EA capital allocators.
They recently described their strategy for AI governance, at a very high level, here.
It is possible that the partnership with Ben Delo we discussed last year may not occur.
Grants
You can see their grants for AI Risk here. It lists 21 AI Risk grants in 2020, plus 4 others for global catastrophic risks and several highly relevant ‘other’ grants. In total I estimate they spent about $19m on AI in 2020.
The largest grants were:
- MIRI: $7.7m
- OpenPhil AI Fellows: $2.3m
- 80k: $3.4m
- Ought: $1.6m
- UC Berkeley (Jacob Steinhardt): $1.1m
- FHI: $1.6m
In contrast were only 4 AI Risk grants listed for 2019, though one of these (CSET) was for $55m.
The OpenPhil AI Fellowship basically fully funds AI PhDs for students who want to work on the long term impacts of AI. Looking back at the 2018 class (who presumably will have had enough time to do significant work since receiving the grants), scanning the abstracts of their publications on their websites suggests that over half have no AI safety relevant publications in 2019 or 2020, and only one is a coauthor on what I would consider a highly relevant paper. Apparently it is somewhat intentional that these fellowships are not intended to be specific to AI safety, though I do not really understand what they are intended for. OpenPhil suggested that part of the purpose was to build a community.
They are also launching a new scholarship program which seems more tailored to people focused on the long-term future, though it is not AI specific.
They produced a list of recommended donation opportunities for small donors; there were zero AI or existential risk opportunities.
Research
Most of their research concerns their own granting, and is often non-public.
Cotra's Report on AI Timelines is a supremely detailed, yet still draft (!), report on how long we should expect the timeline to AGI to be. Impossible for me to do it justice, but essentially it attempts to model both the amount of computational power required to achieve transformative AGI (with current algorithms, the main focus), how much algorithms are improving, and how long it will take to accumulate this hardware. The report estimates doubling times of roughly 2-3 years for both compute and algorithm design. Interestingly, it also suggests that the costs of the final training run will fall as a fraction of overall costs. I liked the way it considers multiple different outside view 'anchors' for different perspectives on the problem - e.g. how much computing did evolution do to produce humans? #Forecasting
Carlsmith's How Much Computational Power Does It Take to Match the Human Brain? attempts to model the FLOPs of the human brain. This is part of their forecasting of when AI will develop to human level capacity (combined with Cotra's report). He does this using multiple methods, which produce generally relatively similar results - as in, not too many orders of magnitude different, generally centered around 10^15 ish. #Forecasting
Finances
To my knowledge they are not currently soliciting donations from the general public, as they have a lot of money from Dustin and Cari, so incremental funding is less of a priority than for other organisations. They could be a good place to work however.
SFF: The Survival and Flourishing Fund
SFF (website) is a donor advised fund, advised by the people who make up BERI’s Board of Directors. SFF was initially funded in 2019 by a grant of approximately $2 million from BERI, which in turn was funded by donations from philanthropist Jaan Tallinn, now also distributing money from Jed McCaleb.
Grants
In its grantmaking SFF uses an innovative allocation process to combine the views of many grant evaluators (described here). SSF has published the results of one grantmaking round this year (described here), where they donated around $1.8m, of which I estimate around $1.2m was AI related; the largest donations in the round were to:
- LessWrong: $400k
- MIRI: $340k
- The Future Society: $160k
- 80k: $180k
- Quantified Uncertainty Research Institute: $120k
I would expect the H2 round, whose results are not yet public, to be at least as large.
Other Organisations
80,000 Hours
80k provides career advice and guidance to people interested in improving the world, with a specific focus on AI safety.
80,000 Hours's AI/ML safety research job board collects various jobs that could be valuable for people interested in AI safety. At the time of writing it listed 80 positions, all of which seemed like good options that it would be valuable to have sensible people fill. I suspect most people looking for AI jobs would find some on here they hadn't heard of otherwise, though of course for any given person many will not be appropriate. They also have job boards for other EA causes. #Careers
They also run a very good podcast; readers might be specifically interested in this or this.
Other News
NeuroIPS rejected four papers this year for being ‘unethical’.
Waymo is (finally) offering a true driverless Uber experience to the general public in Phoenix.
The pope suggested we pray for AI alignment.
There was a minor pandemic.
Methodological Thoughts
Inside View vs Outside View
This document is written mainly, but not exclusively, using publicly available information. In the tradition of active management, I hope to synthesise many pieces of individually well known facts into a whole which provides new and useful insight to readers. Advantages of this are that 1) it is relatively unbiased, compared to inside information which invariably favours those you are close to socially and 2) most of it is legible and verifiable to readers. The disadvantage is that there are probably many pertinent facts that I am not a party to! Wei Dai has written about how much discussion now takes place in private google documents – for example this Drexler piece apparently; in most cases I do not have access to these. If you want the inside scoop I am not your guy; all I can supply is exterior scooping.
We focus on papers, rather than outreach or other activities. This is partly because they are much easier to measure; while there has been a large increase in interest in AI safety over the last year, it’s hard to work out who to credit for this, and partly because I think progress has to come by persuading AI researchers, which I think comes through technical outreach and publishing good work, not popular/political work.
Organisations vs Individuals
Many capital allocators in the bay area seem to operate under a sort of Great Man theory of investment, whereby the most important thing is to identify a guy to invest in who is really clever and ‘gets it’. I think there is a lot of merit in this (as argued here for example); however, I think I believe in it less than they do. Perhaps as a result of my institutional investment background, I place a lot more weight on historical results. In particular, I worry that this approach leads to over-funding skilled rhetoricians and those the investor/donor is socially connected to. Also, as a practical matter, it is hard for individual donors to fund individual researchers. But as part of a concession to the individual-first view I’ve started asking organisations if anyone significant has joined or left recently, though in practice I think organisations are far more willing to highlight new people joining than old people leaving.
Judging organisations on their historical output is naturally going to favour more mature organisations. A new startup, whose value all lies in the future, will be disadvantaged. However, I think that this is the correct approach for donors who are not tightly connected to the organisations in question. The newer the organisation, the more funding should come from people with close knowledge. As organisations mature, and have more easily verifiable signals of quality, their funding sources can transition to larger pools of less expert money. This is how it works for startups turning into public companies and I think the same model applies here. (I actually think that even those with close personal knowledge should use historical results more, to help overcome their biases.)
This judgement involves analysing a large number of papers relating to Xrisk that were produced during 2020. Hopefully the year-to-year volatility of output is sufficiently low that this is a reasonable metric; I have tried to indicate cases where this doesn’t apply. I also attempted to include papers during December 2019, to take into account the fact that I'm missing the last month's worth of output from 2020, but I can't be sure I did this successfully.
Research Inclusion Criteria
In general I have tried to evaluate and summarise, at least briefly, the work organisations did that is primarily concerned with AI or general Xrisk strategy. But this has been a rather subjective and imperfectly applied criteria that was primarily implemented through my subjective sense of ‘does this seem relevant to the task at hand’.
Politics
My impression is that policy on most subjects, especially those that are more technical than emotional is generally made by the government and civil servants in consultation with, and being lobbied by, outside experts and interests. Without expert (e.g. top ML researchers in academia and industry) consensus, no useful policy will be enacted. Pushing directly for policy seems if anything likely to hinder expert consensus. Attempts to directly influence the government to regulate AI research seem very adversarial, and risk being pattern-matched to ignorant technophobic opposition to GM foods or other kinds of progress. We don't want the 'us-vs-them' situation that has occurred with climate change, to happen here. AI researchers who are dismissive of safety law, regarding it as an imposition and encumbrance to be endured or evaded, will probably be harder to convince of the need to voluntarily be extra-safe - especially as the regulations may actually be totally ineffective.
The only case I can think of where scientists are relatively happy about punitive safety regulations, nuclear power, is one where many of those initially concerned were scientists themselves, and also had the effect of basically ending any progress in nuclear power (at great cost to climate change). Given this, I actually think policy outreach to the general population is probably negative in expectation.
If you’re interested in this, I’d recommend you read this blog post from a few years back.
Openness
I think there is a strong case to be made that openness in AGI capacity development is bad. As such I do not ascribe any positive value to programs to ‘democratize AI’ or similar.
One interesting question is how to evaluate non-public research. For a lot of safety research, openness is clearly the best strategy. But what about safety research that has, or potentially has, capabilities implications, or other infohazards? In this case it seems best if the researchers do not publish it. However, this leaves funders in a tough position – how can we judge researchers if we cannot read their work? Maybe instead of doing top secret valuable research they are just slacking off. If we donate to people who say “trust me, it’s very important and has to be secret” we risk being taken advantage of by charlatans; but if we refuse to fund, we incentivize people to reveal possible infohazards for the sake of money. (Is it even a good idea to publicise that someone else is doing secret research?)
For similar reasons I prefer research to not be behind paywalls or inside expensive books, but this seems a significantly less important issue.
More prosaically, organisations should make sure to upload the research they have published to their website! Having gone to all the trouble of doing useful research it is a constant shock to me how many organisations don’t take this simple step to significantly increase the reach of their work. Additionally, several times I have come across incorrect information on organisation’s websites.
Research Flywheel
My basic model for AI safety success is this:
- Identify interesting problems
- As a byproduct this draws new people into the field through altruism, nerd-sniping, apparent tractability
- Solve interesting problems
- As a byproduct this draws new people into the field through credibility and prestige
- Repeat
One advantage of this model is that it produces both object-level work and field growth.
There is also some value in arguing for the importance of the field (e.g. Bostrom’s Superintelligence) or addressing criticisms of the field.
Noticeably absent are strategic pieces. I find that a lot of these pieces do not add terribly much incremental value. Additionally, my suspicion is that strategy research is, to a certain extent, produced exogenously by people who are interested / technically involved in the field. This does not apply to technical strategy pieces, about e.g. whether CIRL or Amplification is a more promising approach.
There is somewhat of a paradox with technical vs ‘wordy’ pieces however: as a non-expert, it is much easier for me to understand and evaluate the latter, even though I think the former are much more valuable.
Differential AI progress
There are many problems that need to be solved before we have safe general AI, one of which is not producing unsafe general AI in the meantime. If nobody was doing non-safety-conscious research there would be little risk or haste to AGI – though we would be missing out on the potential benefits of safe AI.
There are several consequences of this:
- To the extent that safety research also enhances capabilities, it is less valuable.
- To the extent that capabilities research re-orientates subsequent research by third parties into more safety-tractable areas it is more valuable.
- To the extent that safety results would naturally be produced as a by-product of capabilities research (e.g. autonomous vehicles) it is less attractive to finance.
One approach is to research things that will make contemporary ML systems safer, because you think AGI will be a natural outgrowth from contemporary ML. This has the advantage of faster feedback loops, but is also more replaceable (as per the previous section).
Another approach is to try to reason directly about the sorts of issues that will arise with superintelligent AI. This work is less likely to be produced exogenously by unaligned researchers, but it requires much more faith in theoretical arguments, unmoored from empirical verification.
Near-term safety AI issues
Many people want to connect AI existential risk issues to ‘near-term’ issues; I am generally sceptical of this. For example, autonomous cars seem to risk only localised tragedies (though if they were hacked and all crashed simultaneously that would be much worse), and private companies should have good incentives here. Unemployment concerns seem exaggerated to me, as they have been for most of history (new jobs will be created), at least until we have AGI, at which point we have bigger concerns. Similarly, I generally think concerns about algorithmic bias are essentially political - I recommend this presentation - though there is at least some connection to the value learning problem there.
Some people argue that work on these near AI issues is worthwhile because it can introduce people to the broader risks around poor AI alignment. However, I think this is a bad idea - not only does it seem somewhat disingenuous, it risks putting off people who recognise that these are bad concerns. For example, this paper rejects the precautionary principle for AI on the basis of rejecting bad arguments about unemployment - had these pseudo-strawman views not been widespread, it would have been harder to reach this unfortunate conclusion.
It’s also the case many of the policies people recommend as a result of these worries are potentially very harmful. A good example is GDPR and similar privacy regulations (including HIPAA) which have made many good things much more difficult - including degrading our ability to track the pandemic.
Some interesting speculation I read is the idea that discussing near AI safety issues might be a sort of immune response to Xrisk concerns by raising FUD. The ability to respond to long-term AI safety concerns with “yes, we agree AI ethics is very importance, and that’s why we’re working on privacy and decolonising AI” seems like a very rhetorically powerful move.
Financial Reserves
Charities like having financial reserves to provide runway, and guarantee that they will be able to keep the lights on for the immediate future. This could be justified if you thought that charities were expensive to create and destroy, and were worried about this occurring by accident due to the whims of donors. Unlike a company which sells a product, it seems reasonable that charities should be more concerned about this.
Donors prefer charities to not have too much reserves. Firstly, those reserves are cash that could be being spent on outcomes now, by either the specific charity or others. Valuable future activities by charities are supported by future donations; they do not need to be pre-funded. Additionally, having reserves increases the risk of organisations ‘going rogue’, because they are insulated from the need to convince donors of their value.
As such, in general I do not give full credence to charities saying they need more funding because they want much more than a 18 months or so of runway in the bank. If you have a year’s reserves now, after this December you will have that plus whatever you raise now, giving you a margin of safety before raising again next year.
I estimated reserves = (cash and grants) / (2021 budget). In general I think of this as something of a measure of urgency. However despite being prima facie a very simple calculation there are many issues with this data. As such these should be considered suggestive only.
Donation Matching
In general I believe that charity-specific donation matching schemes are somewhat dishonest, despite my having provided matching funding for at least one in the past.
Ironically, despite this view being espoused by GiveWell (albeit in 2011), this is essentially of OpenPhil’s policy of, at least in some cases, artificially limiting their funding to 50% or 60% of a charity’s need, which some charities have argued effectively provides a 1:1 match for outside donors. I think this is bad. In the best case this forces outside donors to step in, imposing marketing costs on the charity and research costs on the donors. In the worst case it leaves valuable projects unfunded.
Obviously cause-neutral donation matching is different and should be exploited. Everyone should max out their corporate matching programs if possible, and things like the annual Facebook Match continue to be great opportunities.
Poor Quality Research
Partly thanks to the efforts of the community, the field of AI safety is considerably more well respected and funded than was previously the case, which has attracted a lot of new researchers. While generally good, one side effect of this (perhaps combined with the fact that many low-hanging fruits of the insight tree have been plucked) is that a considerable amount of low-quality work has been produced. For example, there are a lot of papers which can be accurately summarized as asserting “just use ML to learn ethics”. Furthermore, the conventional peer review system seems to be extremely bad at dealing with this issue.
The standard view here is just to ignore low quality work. This has many advantages, for example 1) it requires little effort, 2) it doesn’t annoy people. This conspiracy of silence seems to be the strategy adopted by most scientific fields, except in extreme cases like anti-vaxers.
However, I think there are some downsides to this strategy. A sufficiently large milieu of low-quality work might degrade the reputation of the field, deterring potentially high-quality contributors. While low-quality contributions might help improve Concrete Problems’ citation count, they may use up scarce funding.
Moreover, it is not clear to me that ‘just ignore it’ really generalizes as a community strategy. Perhaps you, enlightened reader, can judge that “How to solve AI Ethics: Just use RNNs” is not great. But is it really efficient to require everyone to independently work this out? Furthermore, I suspect that the idea that we can all just ignore the weak stuff is somewhat an example of typical mind fallacy. Several times I have come across people I respect according respect to work I found clearly pointless. And several times I have come across people I respect arguing persuasively that work I had previously respected was very bad – but I only learnt they believed this by chance! So I think it is quite possible that many people will waste a lot of time as a result of this strategy, especially if they don’t happen to move in the right social circles.
Having said all that, I am not a fan of unilateral action, and am somewhat selfishly conflict-averse, so will largely continue to abide by this non-aggression convention. My only deviation here is to make it explicit. If you’re interested in this you might enjoy this by 80,000 Hours.
The Bay Area
Much of the AI and EA communities, and especially the EA community concerned with AI, is located in the Bay Area, especially Berkeley and San Francisco. It does have advantages - like proximity to good CS universities - but it is an extremely expensive place, and is dysfunctional both politically and socially. Aside from the lack of electricity and aggressive homelessness, it seems to attract people who are extremely weird in socially undesirable ways – and induces this in those who move there - though to be fair the people who are doing useful work in AI organisations seem to be drawn from a better distribution than the broader community. In general I think the centralization is bad, but if there must be centralization I would prefer it be almost anywhere other than Berkeley. Additionally, I think many funders are geographically myopic, and biased towards funding things in the Bay Area. As such, I have a mild preference towards funding non-Bay-Area projects.
Conclusions
The size of the field continues to grow, both in terms of funding and researchers. Both make it increasingly hard for individual donors. I’ve attempted to subjectively weigh the productivity of the different organisations against the resources they used to generate that output, and donate accordingly.
My constant wish is to promote a lively intellect and independent decision-making among readers; hopefully my laying out the facts as I see them above will prove helpful to some readers. Here is my eventual decision, rot13'd so you can do come to your own conclusions first (which I strongly recommend):
Na vapernfvatyl ynetr nzbhag bs gur orfg jbex vf orvat qbar va cynprf gung qb abg frrz yvxryl gb orarsvg sebz znetvany shaqvat: SUV, Qrrczvaq, BcraNV rgp. Juvyr n tbbq qrirybczrag birenyy - V nz pregnvayl irel cyrnfrq gung Qrrczvaq naq BcraNV unir fhpu cebqhpgvir grnzf - vg zrnaf jr pna’g ernyyl qb zhpu urer.
ZVEV frrzf gb unir tbbq crbcyr naq n tbbq genpx erpbeq, naq gurl fznyy nzbhag gurl eryrnfr vf fgebat. Ohg V pna’g rasbepr shaqvat n ynetr betnavfngvba jvgubhg gnatvoyr rivqrapr sbe znal lrnef.
Bs gur cynprf qbvat svefg-pynff grpuavpny erfrnepu, PUNV frrzf gb zr gb or gur bar gung pbhyq zbfg perqvoyl orarsvg sebz zber shaqvat. V nz n yvggyr pbaprearq Ebuva vf yrnivat, nf ur jnf n irel fgebat pbagevohgbe, naq gur evfx jvgu npnqrzvp vafgvghgvbaf vf gurl trg ‘qvfgenpgrq’. Ohg birenyy V guvax gurl erznva irel cebzvfvat fb V vagraq gb znxr n fvtavsvpnag qbangvba urer.
Va gur cnfg V unir orra dhvgr unefu ba PFRE orpnhfr V sryg gung n ybg bs gurve jbex jnf abg irel eryrinag. Vg qbrf frrz fhowrpgviryl gb zr gung gurve cebqhpgvivgl naq sbphf unf fvtavsvpnagyl vzcebirq ubjrire.
V guvax OREV ner irel vagrerfgvat. Gurve fgengrtl frrzf gb bssre gur punapr gb fvtavsvpnagyl obbfg npnqrzvp (naq guhf znvafgernz-pbaarpgrq naq fgnghf vzohvat) erfrnepu juvyr znvagnvavat n sbphf ba gur zvffvba gung zvtug or ybfg jvgu qverpg tenagf. Zl bar pbaprea urer vf gung gurl ner fbzrguvat bs n bar-zna bcrengvba, naq juvyr V jnf irel snzvyvne jvgu Pevgpu V xabj irel yvggyr nobhg Fnjlre. Ohg birenyy V guvax guvf vf irel cebzvfvat fb V jvyy cebonoyl or qbangvat. Abgr gung guvf vf vaqverpgyl fhccbegvat PFRE nf jryy nf bgure betf yvxr SUV, PUNV rgp.
Svanyyl, V pbagvahr gb yvxr gur YGSS. V’z n yvggyr pbaprearq nobhg hcpbzvat cbffvoyr crefbaary punatrf jura gurl fcva bhg bs PRN, naq jbhyq cersre vs gurl qvqa’g tenag gb betnavfngvbaf ynetr rabhtu gb eha gurve bja shaqenvfvat pnzcnvtaf (naq urapr pna or rinyhngrq ol vaqvivqhny qbabef). Ohg birenyy V guvax vg vf irel nggenpgvir gb shaq fznyy cebwrpgf, naq V nz abg njner bs nal bgure nirahr sbe fznyy qbabef gb genpgnoyl qb guvf. Fb V jvyy or qbangvat gb gurz ntnva guvf lrne.
However, I wish to emphasize that all the above organisations seem to be doing good work on the most important issue facing mankind. It is the nature of making decisions under scarcity that we must prioritize some over others, and I hope that all organisations will understand that this necessarily involves negative comparisons at times.
Thanks for reading this far; hopefully you found it useful. Apologies to everyone who did valuable work that I excluded!
If you found this post helpful, and especially if it helped inform your donations, please consider letting me and any organisations you donate to as a result know.
If you are interested in helping out with next year’s article, please get in touch, and perhaps we can work something out.
Disclosures
I have not in general checked all the proofs in these papers, and similarly trust that researchers have honestly reported the results of their simulations.
I was a Summer Fellow at MIRI back when it was SIAI and volunteered briefly at GWWC (part of CEA). My wife has done some contract work for OpenPhil. I have no financial ties beyond being a donor and have never been romantically involved with anyone else who has ever worked at any of the other organisations.
I shared drafts of the individual organisation sections with representatives from LTFF, FHI, MIRI, CHAI, GCRI, CSER, Ought, AI Impacts, BERI, CLR, GPI, OpenPhil, Convergence.
My eternal gratitude to my anonymous reviewers for their invaluable help, and especially Jess Riedel for the volume and insight of his comments. Any remaining mistakes are of course my own. I would also like to thank my wife and daughter for tolerating all the time I have spent/invested/wasted on this. Negative thanks goes to The Wuhan Institute of Virology and Paradox Interactive.
Sources
This is a list of all the articles cited who with their own individual paragraph. It does not include articles that are only referenced in-line, typically with the word ‘here’.
Aird, Michael - Existential risks are not just about humanity - 2020-04-27 - https://forum.effectivealtruism.org/posts/EfCCgpvQX359xuZ4g/are-existential-risks-just-about-humanity
Aird, Michael - Failures in technology forecasting? A reply to Ord and Yudkowsky - 2020-05-08 - https://www.lesswrong.com/posts/3qypPmmNHEmqegoFF/failures-in-technology-forecasting-a-reply-to-ord-and
Aird, Michael; Shovelain, Justin - Using vector fields to visualise preferences and make them consistent - 2020-01-28 - https://www.lesswrong.com/posts/ky988ePJvCRhmCwGo/using-vector-fields-to-visualise-preferences-and-make-them#comments
Aird, Michael; Shovelain, Justin; Kristoffersson, David - Memetic downside risks: How ideas can evolve and cause harm - 2020-02-25 - https://www.lesswrong.com/posts/EdAHNdbkGR6ndAPJD/memetic-downside-risks-how-ideas-can-evolve-and-cause-harm
AlphaFold Team - AlphaFold: a solution to a 50-year-old grand challenge in biology - 2020-11-30 - https://deepmind.com/blog/article/alphafold-a-solution-to-a-50-year-old-grand-challenge-in-biology
Althaus, David; Baumann, Tobias - Reducing long-term risks from malevolent actors - 2020-04-29 - https://forum.effectivealtruism.org/posts/LpkXtFXdsRd4rG8Kb/reducing-long-term-risks-from-malevolent-actors#comments
Aquirre, Anthony - Why those who care about catastrophic and existential risk should care about autonomous weapons - 2020-11-11 - https://www.lesswrong.com/posts/Btrmh6T62tB4g9RMc/why-those-who-care-about-catastrophic-and-existential-risk#comments
Armstrong, Stuart; Leike, Jan; Orseau, Laurent; Legg, Shane - Pitfalls of Learning a Reward Function Online - 2020-04-28 - https://arxiv.org/abs/2004.13654
Ashurst, Carolyn; Anderljung, Markus; Prunkl, Carina; Leike, Jan; Gal, Yarin; Shevlane, Toby; Dafoe, Allan - A Guide to Writing the NeurIPS Impact Statement - 2020-05-13 - https://medium.com/@GovAI/a-guide-to-writing-the-neurips-impact-statement-4293b723f832
Avin, Sharar; Gruetzemacher, Ross; Fox, James - Exploring AI Futures Through Role Play - 2020-02-26 - https://arxiv.org/abs/1912.08964
Barnes, Beth; Christiano, Paul - Writeup: Progress on AI Safety via Debate - 2020-02-05 - https://www.alignmentforum.org/posts/Br4xDbYu4Frwrb64a/writeup-progress-on-ai-safety-via-debate-1
Baum, Seth - Accounting for violent conflict risk in planetary defense decisions - 2020-09-09 - http://gcrinstitute.org/accounting-for-violent-conflict-risk-in-planetary-defense-decisions/
Baum, Seth - Artificial Interdisciplinarity: Artificial Intelligence for Research on Complex Societal Problems - 2020-07-14 - http://gcrinstitute.org/artificial-interdisciplinarity-artificial-intelligence-for-research-on-complex-societal-problems/
Baum, Seth - Medium-Term Artificial Intelligence and Society - 2020-02-16 - http://gcrinstitute.org/medium-term-artificial-intelligence-and-society/
Baum, Seth - Quantifying the Probability of Existential Catastrophe: A Reply to Beard et al. - 2020-08-10 - http://gcrinstitute.org/quantifying-the-probability-of-existential-catastrophe-a-reply-to-beard-et-al/
Beard, Simon; Kaxzmarek, Patrick - On the Wrongness of Human Extinction - 2020-02-21 - https://www.cser.ac.uk/resources/wrongness-human-extinction/
Beard, Simon; Rowe, Thomas; Fox, James - An Analysis and Evaluation of Methods Currently Used to Quantify the Likelihood of Existential Hazards - 2019-12-03 - https://www.sciencedirect.com/science/article/pii/S0016328719303313
Beard, Simon; Rowe, Thomas; Fox, James - Existential risk assessment: A reply to Baum - 2020-07-15 - https://sci-hub.do/10.1016/j.futures.2020.102606
Belfield, Haydn - Activism by the AI Community: Analysing Recent Achievements and Future Prospects - 2020-02-26 - https://www.cser.ac.uk/resources/activism-ai-community-analysing-recent-achievements-and-future-prospects/
Belfield, Haydn; Hernández-Orallo, José; hÉigeartaigh, Seán Ó; Maas, Matthijs M.; Hagerty, Alexa; Whittlestone, Jess - Response to the European Commission’s consultation on AI - 2020-02-19 - https://www.cser.ac.uk/resources/response-european-commissions-consultation-ai/
Benadè, Gerdus; Nath, Swaprava; Procaccia, Ariel D.; Shah, Nisarg - Preference Elicitation for Participatory Budgeting - 2020-10-27 - https://pubsonline.informs.org/doi/10.1287/mnsc.2020.3666
Benaich, Nathan; Hogarth, Ian - State of AI Report 2020 - 2020-09-01 - https://docs.google.com/presentation/d/1ZUimafgXCBSLsgbacd6-a-dqO7yLyzIl1ZJbiCBUUT4/edit#slide=id.g9348791e5b_1_7
Bhatt, Umang; Andrus, McKane; Weller, Adrian; Xiang, Alice - Machine Learning Explainability for External Stakeholders - 2020-07-10 - https://arxiv.org/abs/2007.05408v1
Bobu, Andreea; Scobee, Dexter R.R.; Fisac, Jaime F.; Sastry, S. Shankar; Dragan, Anca D. - LESS is More: Rethinking Probabilistic Models of Human Behavior - 2020-01-13 - https://arxiv.org/abs/2001.04465
Bostom, Nick; Shulman, Carl - Sharing the World with Digital Minds - 2020-10-01 - http://www.nickbostrom.com/papers/monster.pdf
Bostrom, Nick; Belfield, Haydn; Hilton, Sam - Written Evidence to the UK Parliament Science & Technology Committee's Inquiry on A new UK research funding agency. - 2020-09-16 - https://www.cser.ac.uk/resources/written-evidence-uk-arpa-key-recommendations/
Brown, Tom B.; Mann, Benjamin; Ryder, Nick; Subbiah, Melanie; Kaplan, Jared; Dhariwal, Prafulla; Neelakantan, Arvind; Shyam, Pranav; Sastry, Girish; Askell, Amanda; Agarwal, Sandhini; Herbert-Voss, Ariel; Krueger, Gretchen; Henighan, Tom; Child, Rewon; Ramesh, Aditya; Ziegler, Daniel M.; Wu, Jeffrey; Winter, Clemens; Hesse, Christopher; Chen, Mark; Sigler, Eric; Litwin, Mateusz; Gray, Scott; Chess, Benjamin; Clark, Jack; Berner, Christopher; McCandlish, Sam; Radford, Alec; Sutskever, Ilya; Amodei, Dario - Language Models are Few-Shot Learners - 2020-05-28 - https://arxiv.org/abs/2005.14165
Brundage, Miles; Avin, Shahar; Wang, Jasmine; Belfield, Haydn; Krueger, Gretchen; Hadfield, Gillian; Khlaaf, Heidy; Yang, Jingying; Toner, Helen; Fong, Ruth; Maharaj, Tegan; Koh, Pang Wei; Hooker, Sara; Leung, Jade; Trask, Andrew; Bluemke, Emma; Lebensold, Jonathan; O'Keefe, Cullen; Koren, Mark; Ryffel, Théo; Rubinovitz, JB; Besiroglu, Tamay; Carugati, Federica; Clark, Jack; Eckersley, Peter; Haas, Sarah de; Johnson, Maritza; Laurie, Ben; Ingerman, Alex; Krawczuk, Igor; Askell, Amanda; Cammarota, Rosario; Lohn, Andrew; Krueger, David; Stix, Charlotte; Henderson, Peter; Graham, Logan; Prunkl, Carina; Martin, Bianca; Seger, Elizabeth; Zilberman, Noa; hÉigeartaigh, Seán Ó; Kroeger, Frens; Sastry, Girish; Kagan, Rebecca; Weller, Adrian; Tse, Brian; Barnes, Elizabeth; Dafoe, Allan; Scharre, Paul; Herbert-Voss, Ariel; Rasser, Martijn; Sodhani, Shagun; Flynn, Carrick; Gilbert, Thomas Krendl; Dyer, Lisa; Khan, Saif; Bengio, Yoshua; Anderljung, Markus - Toward Trustworthy AI Development: Mechanisms for Supporting Verifiable Claims - 2020-04-15 - https://arxiv.org/abs/2004.07213
Burden, John & Hernandez-Orallo, Jose - Exploring AI Safety in Degrees: Generality, Capability and Control - 2020-08-10 - https://www.cser.ac.uk/resources/exploring-ai-safety-degrees-generality-capability-and-control/
Byun, Jungwon, Stuhlmuller, Andreas - Automating reasoning about the future at Ought - 2020-11-09 - https://ought.org/updates/2020-11-09-forecasting
Carey, Ryan; Langlois, Eric; Everitt, Tom; Legg, Shane - The Incentives that Shape Behaviour - 2020-01-20 - https://arxiv.org/abs/2001.07118
Carlsmith, Joseph - How Much Computational Power Does It Take to Match the Human Brain? - 2020-09-11 - https://www.openphilanthropy.org/brain-computation-report
Cave, Stephen; Dihal, Kanta - The Whiteness of AI - 2020-08-06 - http://lcfi.ac.uk/resources/whiteness-ai/
Christian, Brian - The Alignment Problem: Machine Learning and Human Values - 2020-09-06 - https://www.amazon.com/Alignment-Problem-Machine-Learning-Values-ebook/dp/B085T55LGK/ref=tmm_kin_swatch_0?_encoding=UTF8&qid=&sr=
Christiano, Paul - “Unsupervised” translation as an (intent) alignment problem - 2020-09-29 - https://ai-alignment.com/unsupervised-translation-as-a-safety-problem-99ae1f9b6b68
Cihon, Peter; Maas, Matthijs M.; Kemp, Luke - Should Artificial Intelligence Governance be Centralised? Design Lessons from History - 2020-01-10 - https://arxiv.org/abs/2001.03573
Clarke, Sam - Clarifying “What failure looks like” (part 1) - 2020-09-20 - https://www.alignmentforum.org/posts/v6Q7T335KCMxujhZu/clarifying-what-failure-looks-like-part-1
Clifton, Jesse - Equilibrium and prior selection problems in multipolar deployment - 2020-04-02 - https://www.alignmentforum.org/posts/Tdu3tGT4i24qcLESh/equilibrium-and-prior-selection-problems-in-multipolar-1#comments
Clifton, Jesse; Riche, Maxime - Towards Cooperation in Learning Games - 2020-11-15 - https://longtermrisk.org/files/toward_cooperation_learning_games_oct_2020.pdf
Cohen, Michael; Hutter, Marcus - Curiosity Killed the Cat and the Asymptotically Optimal Agent - 2020-06-05 - https://arxiv.org/abs/2006.03357
Cohen, Michael; Hutter, Marcus - Pessimism About Unknown Unknowns Inspires Conservatism - 2020-06-15 - https://arxiv.org/abs/2006.08753
Cotra, Ajeya - Report on AI Timelines - 2020-10-18 - https://www.alignmentforum.org/posts/KrJfoZzpSDpnrv9va/draft-report-on-ai-timelines
Cotton‐Barratt, Owen; Daniel, Max; Sandberg, Anders; - Defence in Depth Against Human Extinction: Prevention, Response, Resilience, and Why They All Matter - 2020-01-24 - https://onlinelibrary.wiley.com/doi/full/10.1111/1758-5899.12786
Cremer, Carla; Whittlestone, Jess - Canaries in Technology Mines: Warning Signs of Transformative Progress in AI - 2020-09-24 - https://www.fhi.ox.ac.uk/publications/canaries-in-technology-mines-warning-signs-of-transformative-progress-in-ai-cremer-and-whittlestone/
Critch, Andrew - Some AI research areas and their relevance to existential safety - 2020-11-18 - https://www.alignmentforum.org/posts/hvGoYXi2kgnS3vxqb/some-ai-research-areas-and-their-relevance-to-existential-1
Critch, Andrew; Krueger, David - AI Research Considerations for Human Existential Safety (ARCHES) - 2020-05-30 - https://arxiv.org/abs/2006.04948
Crosby, Matthew; Beyret, Benjamin; Shanahan, Murray; Hernández-Orallo, José; Cheke, Lucy; Halina, Marta - The Animal-AI Testbed and Competition - 2020-09-22 - http://lcfi.ac.uk/resources/animal-ai-testbed-and-competition-paper-purblished/
Demski, Abram - Radical Probabilism - 2020-08-18 - https://www.lesswrong.com/s/HmANELvkhAZ9eDxFS/p/xJyY5QkQvNJpZLJRo
Ding, Jeffrey; Dafoe, Allan - The Logic of Strategic Assets: From Oil to AI - 2020-01-09 - https://arxiv.org/ftp/arxiv/papers/2001/2001.03246.pdf
Freedman, Rachel; Shah, Rohin; Dragan, Anca - Choice Set Misspecification in Reward Inference - 2020-09-10 - http://ceur-ws.org/Vol-2640/paper_14.pdf
Gabriel, Iason - Artificial Intelligence, Values and Alignment - 2020-01-13 - https://arxiv.org/abs/2001.09768
Garfinel, Ben - Does Economic History Point Towards a Singularity? - 2020-09-02 - https://forum.effectivealtruism.org/posts/CWFn9qAKsRibpCGq8/does-economic-history-point-toward-a-singularity
Garrabrant, Scott - Cartesian Frames - 2020-10-22 - https://www.alignmentforum.org/s/2A7rrZ4ySx6R8mfoT
Gleave, Adam; Dennis, Michael; Legg, Shane; Russell, Stuart; Leike, Jan - QUANTIFYING DIFFERENCES IN REWARD FUNCTIONS - 2020-10-08 - https://arxiv.org/abs/2006.13900
Grace, Katja - Atari early - 2020-04-01 - https://aiimpacts.org/atari-early/
Grace, Katja - Discontinuous progress in history: an update - 2020-04-13 - https://aiimpacts.org/discontinuous-progress-in-history-an-update/
Halpern, Joseph; Piermont, Evan - Dynamic Awareness - 2020-07-06 - https://arxiv.org/abs/2007.02823
hÉigeartaigh, Seán Ó; Whittlestone, Jess; Liu, Yang; Zeng, Yi; Liu, Zhe - Overcoming Barriers to Cross-cultural Cooperation in AI Ethics and Governance - 2020-05-15 - https://link.springer.com/article/10.1007/s13347-020-00402-x
Hendrycks, Dan; Burns, Collin; Basart, Steven; Critch, Andrew; Li, Jerry; Song, Dawn; Steinhardt, Jacob - Aligning AI with Shared Human Values - 2020-08-05 - https://arxiv.org/abs/2008.02275
Henighan, Tom; Kaplan, Jared; Katz, Mor; Chen, Mark; Hesse, Christopher; Jackson, Jacob; Jun, Heewoo; Brown, Tom B.; Dhariwal, Prafulla; Gray, Scott; Hallacy, Chris; Mann, Benjamin; Radford, Alec; Ramesh, Aditya; Ryder, Nick; Ziegler, Daniel M.; Schulman, John; Amodei, Dario; McCandlish, Sam - Scaling Laws for Autoregressive Generative Modeling - 2020-11-06 - https://arxiv.org/abs/2010.14701?fbclid=IwAR3H_-kH2TKQXl4GcVGLXsfZv2JfD_mOlRdQfXFuAZDttPoHMRKyHITgo74
Hernandez-Orallo, Jose; Martinez-Plumed, Fernando; Avin, Shahar; Whittlestone, Jess; hÉigeartaigh, Seán Ó - AI Paradigms and AI Safety: Mapping Artefacts and Techniques to Safety Issues - 2020-08-10 - https://www.cser.ac.uk/resources/ai-paradigms-and-ai-safety-mapping-artefacts-and-techniques-safety-issues/
Hollanek, Tomasz - AI transparency: a matter of reconciling design with critique - 2020-11-17 - https://link.springer.com/article/10.1007%2Fs00146-020-01110-y#author-information
Hubinger, Evan - An overview of 11 proposals for building safe advanced AI - 2020-05-29 - https://www.alignmentforum.org/posts/fRsjBseRuvRhMPPE5/an-overview-of-11-proposals-for-building-safe-advanced-ai
Hwang, Tim - Shaping the Terrain of AI Competition - 2020-06-15 - https://cset.georgetown.edu/research/shaping-the-terrain-of-ai-competition/
Imbrie, Andrew; Kania, Elsa; Laskai, Lorand - The Question of Comparative Advantage in Artificial Intelligence: Enduring Strengths and Emerging Challenges for the United States - 2020-01-15 - https://cset.georgetown.edu/research/the-question-of-comparative-advantage-in-artificial-intelligence-enduring-strengths-and-emerging-challenges-for-the-united-states/
John, Tyler; MacAskill, William - Longtermist institutional reform - 2020-07-30 - https://philpapers.org/rec/JOHLIR
Kemp, Luke; Rhodes, Catherine - The Cartography of Global Catastrophic Risks - 2020-01-06 - https://www.cser.ac.uk/resources/cartography-global-catastrophic-governance/
Kokotajlo, Daniel - Relevant pre-AGI possibilities - 2020-06-18 - https://aiimpacts.org/relevant-pre-agi-possibilities/
Kokotajlo, Daniel - Three kinds of competitiveness - 2020-03-30 - https://aiimpacts.org/three-kinds-of-competitiveness/
Korzekwa, Rick - Description vs simulated prediction - 2020-04-22 - https://aiimpacts.org/description-vs-simulated-prediction/
Korzekwa, Rick - Preliminary survey of prescient actions - 2020-04-08 - https://aiimpacts.org/survey-of-prescient-actions/
Kovařík, Vojtěch ; Carey, Ryan - (When) Is Truth-telling Favored in AI Debate? - 2019-12-15 - https://arxiv.org/abs/1911.04266
Krakovna, Victoria - Possible takeaways from the coronavirus pandemic for slow AI takeoff - 2020-05-31 - https://vkrakovna.wordpress.com/2020/05/31/possible-takeaways-from-the-coronavirus-pandemic-for-slow-ai-takeoff/
Krakovna, Victoria; Orseau, Laurent; Ngo, Richard; Martic, Miljan; Legg, Shane - Avoiding Side Effects By Considering Future Tasks - 2020-10-15 - https://arxiv.org/abs/2010.07877v1
Krakovna, Victoria; Uesato, Jonathan; Mikulik, Vladimir; Rahtz, Matthew; Everitt, Tom; Kumar, Ramana; Kenton, Zac; Leike, Jan; Legg, Shane - Specification gaming: the flip side of AI ingenuity - 2020-04-21 - https://deepmind.com/blog/article/Specification-gaming-the-flip-side-of-AI-ingenuity
Lehman, Joel - Reinforcement Learning Under Moral Uncertainty - 2020-06-15 - https://arxiv.org/abs/2006.04734
Linsefors, Linda & Hepburn, JJ - Announcing AI Safety Support - 2020-11-19 - https://forum.effectivealtruism.org/posts/wpQ2qhF8Z6oonsaPX/announcing-ai-safety-support
MacAskill, Will - Are we living at the hinge of history? - 2020-09-01 - https://globalprioritiesinstitute.org/wp-content/uploads/William-MacAskill_Are-we-living-at-the-hinge-of-history.pdf
Makiievskyi, Anton; Zhou, Liang ; Chiswick, Max - Assessing Generalization in Reward Learning with Procedurally Generated Games - 2020-08-30 - https://towardsdatascience.com/assessing-generalization-in-reward-learning-intro-and-background-da6c99d9e48
Mogensen, Andreas - Moral demands and the far future - 2020-06-01 - https://globalprioritiesinstitute.org/wp-content/uploads/Working-Paper-1-2020-Andreas-Mogensen.pdf
Mogensen, Andreas; Thorstad, David - Tough enough? Robust satisficing as a decision norm for long-term policy analysis - 2020-11-01 - https://globalprioritiesinstitute.org/wp-content/uploads/Tough-Enough_Andreas-Mogensen-and-David-Thorstad.pdf
Ngo, Richard - AGI Safety from First Principles - 2020-09-28 - https://www.alignmentforum.org/s/mzgtmmTKKn5MuCzFJ
Nguyen, Chi; Christiano, Paul - My Understanding of Paul Christiano's Iterated Amplification AI Safety Research Agenda - 2020-08-15 - https://www.lesswrong.com/posts/PT8vSxsusqWuN7JXp#comments
O’Keefe, Cullen; Cihon, Peter; Garfinkel, Ben; Flynn, Carrick; Leung, Jade; Dafoe,Allan - The Windfall Clause: Distributing the Benefits of AI for the Common Good - 2020-01-30 - https://www.fhi.ox.ac.uk/windfallclause/
O'Brien, John; Nelson, Cassidy - Assessing the Risks Posed by the Convergence of Artificial Intelligence and Biotechnology - 2020-06-17 - https://www.liebertpub.com/doi/full/10.1089/hs.2019.0122
O'Keefe, Cullen - How will National Security Considerations affect Antitrust Decisions in AI? An Examination of Historical Precedents - 2020-07-28 - https://forum.effectivealtruism.org/out?url=https%3A%2F%2Fwww.fhi.ox.ac.uk%2Fwp-content%2Fuploads%2FHow-Will-National-Security-Considerations-Affect-Antitrust-Decisions-in-AI-Cullen-OKeefe.pdf
Ord, Toby - The Precipice - 2020-03-24 - https://www.amazon.com/Precipice-Existential-Risk-Future-Humanity-ebook/dp/B07V9GHKYP/ref=tmm_kin_swatch_0?_encoding=UTF8&qid=&sr=
Peters, Dorian; Vold, Karina; Robinson, Diana; Calvo, Rafael - Responsible AI—Two Frameworks for Ethical Design Practice - 2020-02-15 - https://ieeexplore.ieee.org/document/9001063/authors#authors
Prunkl, Carina; Whittlestone, Jess - Beyond Near- and Long-Term: Towards a Clearer Account of Research Priorities in AI Ethics and Society - 2020-01-13 - https://arxiv.org/abs/2001.04335
Qian, Shi; Hui, Li; Tse, Brian; Hopcroft, John; Russell, Stuart; Jeanmaire, Caroline; Qiang, Yang; Fung, Pascale; Yampolskiy, Roman; Dafoe, Allan; Anderljung, Markus; Hadfield, Gillian; Wright, Don; Brundage, Miles; Clark, Jack; Solaiman, Irene; Krueger, Gretchen; O' hEigeartaigh, Sean; Toner, Helen; Liu, Millie; Hoffman, Steve; Beridze, Irakli; Wallach, Wendell; Hodes, Cyrus; Miailhe, Nicolas; Newman, Jessica; Dingding, Chen; Kaili, Eva; Jun, Su; Hagendorff, Thilo; Ahrweiler, Petra; Williams, Robin; Allen, Colin; Wang, Poon; Carbonell, Ferran; Ziaohong, Wang; Qingfend, Yang; Qi, Yin; Rossie, Francesca; Stix, Charlotte; Daly, Angela; Gal, Danit; Ema, Arisa; Yihan, Goh; Remolina, Nydia; Aneja, Urvashi; Ying, Fu; Zhiyun, Zhao; Xiuquan, Li; Weiwen, Duan; Qun, Luan; Rui, Guo; Yingchun, Wang - AI GOVERNANCE IN 2019 A YEAR IN REVIEW - 2020-04-15 - https://www.aigovernancereview.com/
Reddy, Siddharth; Dragan, Anca D.; Levine, Sergey; Legg, Shane; Leike, Jan - Learning Human Objectives by Evaluating Hypothetical Behavior - 2019-12-05 - https://arxiv.org/abs/1912.05652
Russell, Stuart; Norvig, Peter - Artificial Intelligence: A Modern Approach, 4th Edition - 2020-01-01 - https://www.pearson.com/us/higher-education/program/Russell-Artificial-Intelligence-A-Modern-Approach-4th-Edition/PGM1263338.html
Saunders, William; Rachbach, Ben; Evans, Owain; Byun, Jungwon; Stuhlmüller, and Andreas - Evaluating Arguments One Step at a Time - 2020-01-11 - https://ought.org/updates/2020-01-11-arguments
Scholl, Keller; Hanson, Robin - Testing the Automation Revolution Hypothesis - 2019-12-10 - https://papers.ssrn.com/sol3/papers.cfm?abstract_id=3496364
Shah, Rohin - AI Alignment 2018-19 Review - 2020-01-27 - https://www.alignmentforum.org/posts/dKxX76SCfCvceJXHv/ai-alignment-2018-19-review#Short_version___1_6k_words_
Shevlane, Toby; Dafoe, Allan - The Offense-Defense Balance of Scientific Knowledge: Does Publishing AI Research Reduce Misuse? - 2020-12-27 - https://arxiv.org/abs/2001.00463
Snyder-Beattie, Andrew; Sandberg, Anders; Drexler, Eric; Bonsall, Michael - The Timing of Evolutionary Transitions Suggests Intelligent Life Is Rare - 2020-11-19 - https://www.liebertpub.com/doi/full/10.1089/ast.2019.2149
Stiennon, Nisan; Ouyang, Long; Wu, Jeff; Ziegler, Daniel M.; Lowe, Ryan; Voss, Chelsea; Radford, Alec; Amodei, Dario; Christiano, Paul - Learning to Summarize with Human Feedback - 2020-09-04 - https://openai.com/blog/learning-to-summarize-with-human-feedback/
Tarsney, Christian - Exceeding Expectations: Stochastic Dominance as a General Decision Theory - 2020-08-08 - https://globalprioritiesinstitute.org/wp-content/uploads/Christian-Tarsney_Exceeding-Expectations_Stochastic-Dominance-as-a-General-Decision-Theory.pdf
Tarsney, Christian; Thomas, Teruji - Non-Additive Axiologies in Large Worlds - 2020-09-01 - https://globalprioritiesinstitute.org/wp-content/uploads/Christian-Tarsney-and-Teruji-Thomas_Non-Additive-Axiologies-in-Large-Worlds.pdf
Thorstad, David; Mogensen, Andreas - Heuristics for clueless agents: how to get away with ignoring what matters most in ordinary decision-making - 2020-06-01 - https://globalprioritiesinstitute.org/wp-content/uploads/David-Thorstad-Andreas-Mogensen-Heuristics-for-clueless-agents.pdf
Trammell, Philip; Korinek, Anton - Economic growth under transformative AI - 2020-10-08 - https://globalprioritiesinstitute.org/wp-content/uploads/Philip-Trammell-and-Anton-Korinek_Economic-Growth-under-Transformative-AI.pdf
Tucker, Aaron; Anderljung, Markus; Dafoe, Allan - Social and Governance Implications of Improved Data Efficiency - 2020-01-14 - https://arxiv.org/pdf/2001.05068.pdf
Tzachor, Asaf; Whittlestone, Jess; Sundaram, Lalitha; , Seán Ó hÉigeartaigh - Artificial intelligence in a crisis needs ethics with urgency - 2020-12-02 - https://www.nature.com/articles/s42256-020-0195-0
Uesato, Jonathan; Kumar, Ramana; Krakovna, Victoria; Everitt, Tom; Ngo, Richard; Legg, Shane - Avoiding Tampering Incentives in Deep RL via Decoupled Approval - 2020-11-17 - https://arxiv.org/abs/2011.08827
Wilkinson, Haydn - In defence of fanaticism - 2020-08-01 - https://globalprioritiesinstitute.org/wp-content/uploads/Hayden-Wilkinson_In-defence-of-fanaticism.pdf
Xu, Jing; Ju, Da; Li, Margaret; Boureau, Y-Lan; Weston, Jason; Dinan, Emily - Recipes for Safety in Open-domain Chatbots - 2020-09-14 - https://arxiv.org/abs/2010.07079
Zerilli, John; Knott, Alistair; Maclaurin, James; Colin, Gavaghan - Algorithmic Decision-Making and the Control Problem - 2020-12-11 - https://link.springer.com/article/10.1007%2Fs11023-019-09513-7
Fascinating that very few top AI Safety organizations are looking for more funding. By my count, only 4 of these 17 organizations are even publicly requesting donations this year: three independent research groups (GCRI, CLR, and AI Impacts) and an operations org (BERI). Across the board, it doesn't seem like AI Safety is very funding constrained.
Based on this report, I think the best donation opportunity among these orgs is BERI, the Berkeley Existential Risk Initiative. Larks says that BERI "provides support to existential risk groups at top universities to facilitate activities (like hiring engineers and assistants) that would be hard within the university context." According to BERI's blog post requesting donations, this support includes:
BERI is also supporting new existential risk research groups at other top universities, including:
Donating to BERI seems to me like the only way to give more money to AI Safety researchers at top universities. FHI, CHAI, and CSER aren't publicly seeking donations seemingly because anything you directly donate might end up either (a) replacing funding they would've received from their university or other donors, or (b) being limited in terms of what they're allowed to spend it on. If that's true, then the only way to counterfactually increase funding at these groups is through BERI.
If you would like, click here to donate to BERI.
Depending on how you interpret this comment, the LTFF is looking for funding as well.
(Disclosure: I run EA Funds.)
Yes, looks like LTFF is also looking for funding. Edited, thanks.
[Disclosure: I work for CSER]
I completely agree that BERI is a great organisation and a good choice. However, I will also just breifly note that FHI, CHAI and CSER (like any academic groups) are always open to receiving donations:
FHI: https://www.fhi.ox.ac.uk/support-fhi/
CSER: https://www.philanthropy.cam.ac.uk/give-to-cambridge/centre-for-the-study-of-existential-risk?table=departmentprojects&id=452
CHAI: If you wanted to donate to them, here is the relevant web page. Unfortunately it is apparently broken at time of writing - they tell me any donation via credit card can be made by calling the Gift Services Department on 510-643-9789.
Thanks Hayden!
FLI also is quite funding constrained particularly on technical-adjacent policy research work, where in my opinion there is going to be a lot of important research and a dearth of resources to do it. For example, the charge to NIST to develop an AI risk assessment framework, just passed in the US NDAA, is likely to be extremely critical to get right. FLI will be working hard to connect technical researchers with this effort, but is very resource-constrained.
I generally feel that the idea that AI safety (including research) is not funding constrained to be an incorrect and potentially dangerous one — but that's a bigger topic for discussion.
AI Impacts now has a 2020 review page so it's easier to tell what we've done this year-- this should be more complete / representative than the posts listed above. (I appreciate how annoying the continuously updating wiki model is.)
You have this reference: https://ieeexplore.ieee.org/document/9001063/authors#authors where the first paragraph reads:
"In the last year, the Association for Computing Machinery (ACM) released new ethical standards for professional conduct [1] and the IEEE released guidelines for the ethical design of autonomous and intelligent systems [2] demonstrating a shift among professional technology organizations toward prioritizing ethical impact. In parallel, thousands of technology professionals and social scientists have formed multidisciplinary committees to devise ethical principles for the design, development, and use of artificial intelligence (AI) technologies [3]. Moreover, many governments and international organizations have released sets of ethical principles, including the OECD Principles in 2019 [4], the Montreal Declaration in 2017 [5], the U.K. House of Lords report “AI in the U.K.: ready willing and able?” in 2018 [6], the European Commission High-Level Expert Group (HLEG) on AI in 2018 [7], and the Beijing AI Principles in 2019 [8]. Indeed, recent reports indicate that there are currently more than 70 publicly available sets of ethical principles or frameworks for AI, most of which have been released within the last five years [3], [9], [10]."
I am just wondering if your review would not be more complete by mentioning that kind of work. The IEEE for instance has this page: https://ethicsinaction.ieee.org/
Thanks a lot for the review, I was looking forward to reading it, it updated me further towards donating to the LTFF this year and you reviewed some really interesting papers that I haven't noticed before.
I think that's a reasonable point. It was also touched upon in the recent AMA of the LTFF, and the fund managers seem to agree that funding individuals and smaller projects is their comparative advantage. I personally won't feel taxed if they decided that some established org can use my money best as long as a meaningful fraction of the money goes towards projects I wouldn't have heard of.
Thank you for this! I'm not sure if this was intentional or not, but it seems worth noting that my work with Robin was funded under a grant from OpenPhil, including my salary as a research assistant and some bought off classes for him.
As always, thanks for providing this valuable resource!
Small point: I think that where you say "200k: $180k", it should say "80k: $180k" (i.e., I think the org which received $180k was 80,000 Hours).
Thanks, fixed
This was a great reference when the every.org match started and I needed to evaluate more organizations quickly. Thanks!
This is extremely useful information for deciding what AI charities to donate to.
Regarding ARCHES
Where's that? I'd be very interested to see an argument to that. I looked around and found a lot of reasons prepotence is dangerous, and ways to avoid it, but wasn't able to find an argument that it is decisively more dangerous than its negative.
(I do suspect non-prepotence is dangerous. In short: Prepotent AGI can and visibly is required to exceed us morally (not in the sense of making metaphysical moral progress, I don't believe in that, I mean that there can be higher levels of patience, lucidity, and knowledge of the human CEV and its applications, that would bring the thing to conclusions we'd find shocking), there's a sense in which prepotent AGI would be harder to weaponize, harder to train on a single profane object level objective and fire, it is less tempting to try to use it to do stupid rash things we will grow to regret because the consequences of using it in stupid rash regrettable ways are so much more immediately obviously irrevocable. In the longest term, building agentic infrastructures that maintain binding treaties will be a necessity for overcoming the last coordination problems, that's another reason that prepotence is innevitable. Notably, the treaty "It should be globally impossible to make prepotent AGI" would itself manifest as a prepotent agency. The idea that prepotence is or should be avoidable might be conceptually unworkable.)
(In my skim-read, I also couldn't find discussion of the feasibility of aligned prepotent AI, and that's making me start to wonder if there might be a perilous squeamishness around. There are men who worry about being turned into housecats, work vainly on improving the human brain with neural meshes, so that humans can compete with AI. The reality is the housecats will be happy and good and as enfranchised as they wish to be, and human brains will not compete, and that will be fine. It's imaginable that this aversion to prepotence comes from a regressive bravado that has been terminally outmoded since the rise of the first city-state. If I'm missing the mark on this though, apologies for premature psychologizing.)
Typo: You say 2020 when you should say 2019 at the beginning.
Thanks, fixed.