See also: List of Tools for Collaborative Truth Seeking
Squiggle is a special-purpose programming language for generating probability distributions, estimates of variables over time, and similar tasks, with reasonable transparency. It was developed by the Quantified Uncertainty Research Institute.
It's a broad tool applicable to a lot of tasks with probabilistic elements. It lies somewhere between Guesstimate and full probabilistic programming languages or Python (a good summary of squiggle's features can be found here or here).
When can Squiggle be Useful?
- Estimating Return on Investment from projects
- Estimating probability of a project's success
- Comparing impact of different career paths
- Estimate the best time to run a retreat based on predicted COVID numbers
- Find cruxes where two forecasters disagree by building full models of how they derived their beliefs
- Give somebody a precise & detailed look at your internal model and assumptions on a topic
Tutorials
The official basics guide is a good written guide if you have experience programming and/or have a specific project in mind already. A video tutorial using a worked example is available here on loom, or below:
There’s also a few simple worked examples to demonstrate Squiggle’s value, and a more complex model based on GiveWell's cost effectiveness analyses. Note that Squiggle is still in development and has several known bugs. If you want to just play around with Squiggle, there's a web implementation for experimenting available here.
Here's an example lifted directly from the official documentation of using Squiggle to estimate the number of piano tuners in NYC (a canonical fermi estimation question):
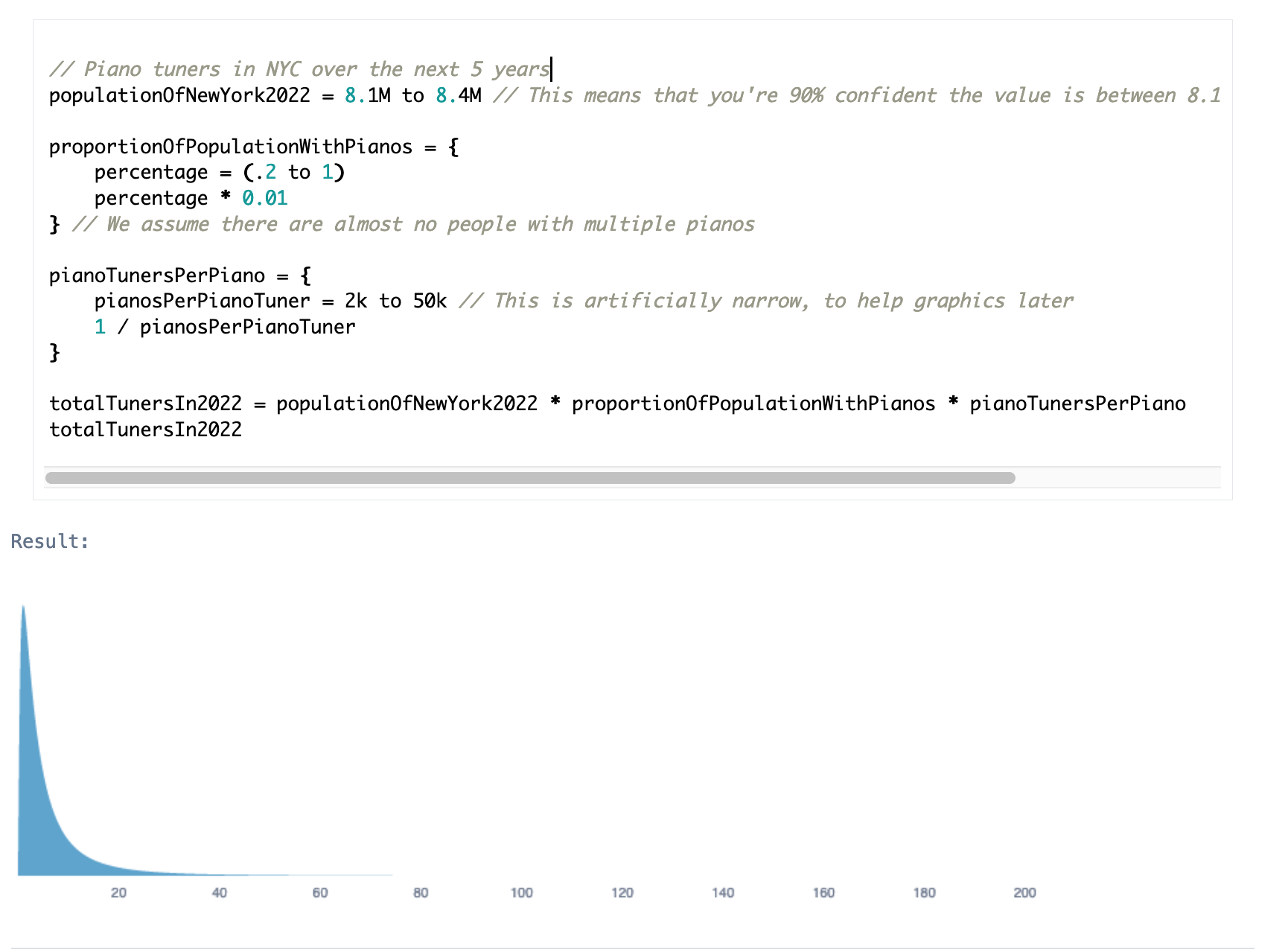
Examples
- Number of piano tuners in New York (model+explanation)
- Estimate ROI from projects with uncertain costs in effort, time, energy, and with uncertain relative value (forum post, model)
- Estimate probability of a nuclear explosion in London (forum post, model)
- Estimate impact of various career paths (forum post, model)
- Estimate impact of improving the forum karma system (forum post, model, video walkthrough)
- How much time in your lifetime do you spend brushing your teeth? (model)
Personal Experience
Squiggle is fairly easy to get started with if you've got any experience with programming (particularly statistical programming in R or python), and you get a lot of bang for your buck in terms of functionality vs ease of use.
Squiggle's main downside is that it's harder to understand[1] than similar tools like Guesstimate or Causal. This follows pretty naturally from being code-based, and can be mitigated in all the usual ways (suggestive variable names, thorough commenting, etc.), or by integrating it with google docs.
With larger models the legibility issue becomes something of a problem. By default, Squiggle outputs a huge list of all outputs in the order they appear in the code. However, if the last line is a variable or object, Squiggle only shows that variable/object; you can use this to query models (see e.g. this model).
Overall, I'd tentatively recommend squiggle for medium-complexity models (say, taking less than 10 hours total to create) if you're already comfortable working with code, and don't anticipate sharing the model with a lot of people (or any people who are especially unfamiliar with code). If you have larger models, the QURI team are happy to be contacted-- squiggle can be useful for larger models, but needs to be set up with VSCode and some other tools.
If you want simpler models that are easier to share with others, I'd recommend Guesstimate or Causal. If you want more complex models (and don't care about their shareability, or are willing to mitigate this with good documentation), I'd recommend using python or finding a suitable probabilistic programming language (making recommendations here is far beyond the scope of this guide).
Try it Yourself!
Have a look through the examples above, and think about a forum post you read recently that could benefit from being more quantitative-- make a toy model of it! It should be very simple to start with to check your basic assumptions; you can always add detail later.
Want to make a model to practice, but don't know of what?
Try one of these! I think a Squiggle model could be really useful here.
- Estimate impact of various factors on shrimp welfare (forum post)
- Estimate impact of sound therapy for tinnitus (forum post)
- Compare impact of different cause areas (forum post)
If those are a little intimidating, try these instead (click through to a guesstimate model for some inspiration on how to model the problem, if you like):
- How long do you spend brushing your teeth over a lifetime?
- How many Gremlins are lost each year to microwave-related violence?
- How many cats does it take to fill a car with fluff?
Join us at 6pm GMT tomorrow [31/01] for an event in the EA GatherTown discussing the use of squiggle & doing a short exercise with it!
Tomorrow's post will be on forecasting tools of various kinds!
Thanks to Ozzie Gooen for comments on the final draft.
- ^
To yourself six months later, or to other people you're sharing your model with
There's also a version of Squiggle in python if you're more keen to use Python! https://github.com/rethinkpriorities/squigglepy
Confirming this!
How much work would it be to make something like this as a python library, and how much would that reduce its usefulness? I think this is really cool and have been looking for something like this, but I am multiple times more likely to use something if it's a python library as opposed to a brand new language, and I assume others think similarly.
https://github.com/rethinkpriorities/squigglepy